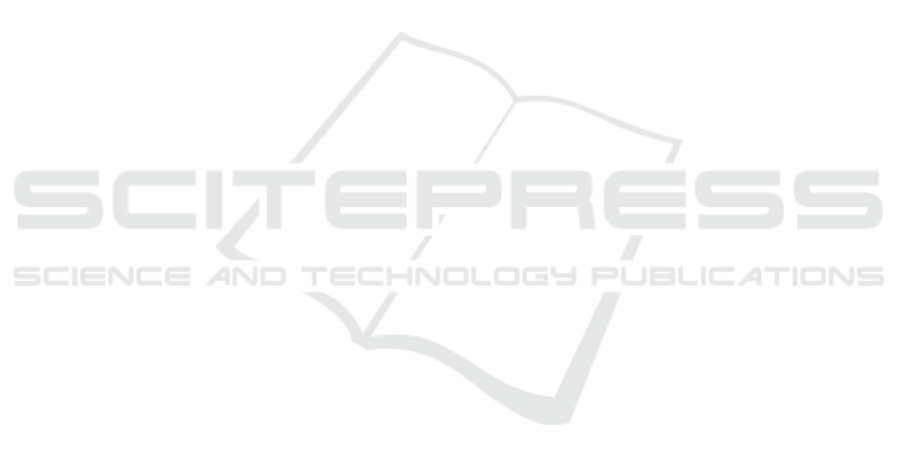
4.3 Ablation Study
In order to investigate the contribution of each part
of the propose framework to the final accuracy we
perform experiments on Columbia database. Specif-
ically, we studied the impact of fine-tuning the gaze
estimation network using the calibration samples, as
well as, the impact of importing the head pose infor-
mation to the final performance. Results presented
on Table 3 demonstrate the importance of these parts.
Specifically, the head pose information increases ac-
curacy by 1.2
o
, while the fine-tuning of the network
adds 1.8
o
more accuracy increase.
Finally, we studied the impact of the applied gaze-
preserving transformation of Eq. (2) to the reference
images. Results showed an accuracy decrease of 1.6
o
(from 6.1
o
to 7.7
o
) after removing this step, revealing
that this step is crucial in order to disentangle the gaze
feature from the eye-related features.
5 CONCLUSIONS
In this paper a few-shot gaze estimation method was
introduced. In order to overcome the dependency of
the labeled data, the proposed framework aimed to
learn an unsupervised gaze representation via the joint
training of a gaze transfer and a gaze estimation net-
work. Only few calibration samples were enough to
fine-tune the gaze estimation network with promis-
ing accuracy results. Extensive evaluation of the pro-
posed method was performed on two publicly avail-
able databases. A comparison with existing few-shot
gaze estimation methods demonstrated a significant
improvement in accuracy in within-dataset experi-
ments. Also, the benefits of every individual step of
the proposed framework to the achieved performance
were highlighted. The validity of this work makes us
believe that this approach can be used as a pretraining
process in order to exploit the great amount of the ex-
isting unlabeled data and become less dependent from
the labeled ones.
ACKNOWLEDGEMENTS
This research has been co-financed by the Euro-
pean Union and Greek national funds through the
Operational Program Competitiveness, Entrepreneur-
ship and Innovation, under the call RESEARCH –
CREATE – INNOVATE (project SignGuide, code:
T2EDK - 00982)
REFERENCES
Caron, M., Bojanowski, P., Joulin, A., and Douze, M.
(2018). Deep clustering for unsupervised learning of
visual features. In European Conference on Computer
Vision (ECCV), pages 132–149.
Chen, M., Jin, Y., Goodall, T., Yu, X., and Bovik, A. C.
(2020). Study of 3d virtual reality picture quality.
IEEE Journal of Selected Topics in Signal Processing,
14:89–102.
Cheng, Y., Lu, F., and Zhang, X. (2018). Appearance-based
gaze estimation via evaluation-guided asymmetric re-
gression. In European Conference on Computer Vi-
sion (ECCV), pages 100–115.
Crawford, E. and Pineau, J. (2019). Spatially invariant un-
supervised object detection with convolutional neural
networks. In AAAI Conference on Artificial Intelli-
gence, pages 3412–3420.
Eckstein, K. M., Guerra-Carrillo, B., Miller Singley, A. T.,
and Bunge, A. S. (2017). Beyond eye gaze: What
else can eyetracking reveal about cognition and cog-
nitive development? Developmental Cognitive Neu-
roscience, 25:69–91.
Gideon, J., S. S. and Stent, S. (2021). Unsupervised multi-
view gaze representation learning. In International
Conference on Computer Vision and Pattern Recog-
nition Workshops (CVPRW), pages 5001–5009.
Grill, J., Strub, F., Altch?, F., Tallec, C., Richemond, P.,
Buchatskaya, E., Doersch, C., Avila Pires, B., Guo, Z.,
Gheshlaghi Azar, M., and Piot, B. (2020). Bootstrap
your own latent-a new approach to self-supervised
learning. In Advances in Neural Information Process-
ing Systems, pages 21271–21284.
Huang, M. X., Li, J., Ngai, G., and Leong, H. V. (2016).
Stressclick: Sensing stress from gaze-click patterns.
In 24th ACM International Conference on Multime-
dia, pages 1395–1404.
Kartynnik, Y., Ablavatski, A., Grishchenko, I., and Grund-
mann, M. (2019). Real-time facial surface geome-
try from monocular video on mobile gpus. In Inter-
national Conference on Computer Vision and Pattern
Recognition Workshops (CVPRW).
Kellnhofer, P., Recasens, A., Stent, S., Matusik, W., and
Torralba, A. (2019). Gaze360: Physically uncon-
strained gaze estimation in the wild. In International
Conference on Computer Vision (ICCV).
Kingma, D. and Ba, J. (2015). Adam: A method for
stochastic optimization. In International Conference
on Learning Representations (ICLR).
Krafka, K., Khosla, A., Kellnhofer, P., Kannan, H., Bhan-
darkar, S., Matusik, W., and Torralba, A. (2016). Eye
tracking for everyone. In International Conference
on Computer Vision and Pattern Recognition (CVPR),
pages 2176–2184.
Lu, F., Sugano, Y., Okabe, T., and Y., S. (2014). Adap-
tive linear regression for appearance-based gaze esti-
mation. IEEE Transactions on Pattern Analysis and
Machine Intelligence (PAMI), 36:2033–2046.
Moriya, T., Roth, H., Nakamura, S., Oda, H., Nagara, K.,
Oda, M., and Mori, K. (2018). Unsupervised segmen-
VISAPP 2023 - 18th International Conference on Computer Vision Theory and Applications
812