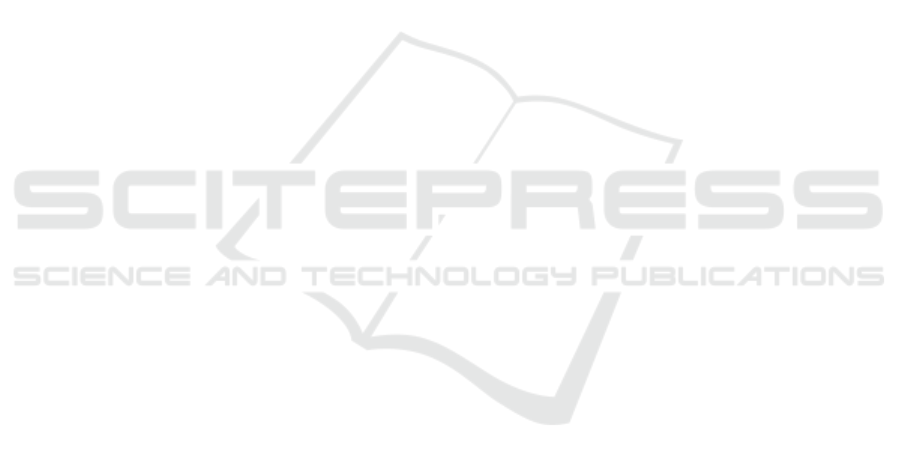
REFERENCES
Abati, D., Tomczak, J., Blankevoort, T., Calderara, S.,
Cucchiara, R., and Bejnordi, B. E. (2020). Condi-
tional channel gated networks for task-aware contin-
ual learning. In Proceedings of the IEEE/CVF Con-
ference on Computer Vision and Pattern Recognition,
pages 3931–3940.
Abbasi, A., Nooralinejad, P., Braverman, V., Pirsiavash, H.,
and Kolouri, S. (2022). Sparsity and heterogeneous
dropout for continual learning in the null space of neu-
ral activations. arXiv preprint arXiv:2203.06514.
Adel, T., Zhao, H., and Turner, R. E. (2020). Con-
tinual learning with adaptive weights (CLAW). In
8th International Conference on Learning Represen-
tations, ICLR 2020, Addis Ababa, Ethiopia, April 26-
30, 2020. OpenReview.net.
Bengio, E., Bacon, P.-L., Pineau, J., and Precup, D. (2015).
Conditional computation in neural networks for faster
models. arXiv preprint arXiv:1511.06297.
Bolukbasi, T., Wang, J., Dekel, O., and Saligrama, V.
(2017). Adaptive neural networks for efficient infer-
ence. In International Conference on Machine Learn-
ing, pages 527–536. PMLR.
Buzzega, P., Boschini, M., Porrello, A., Abati, D., and
Calderara, S. (2020). Dark experience for general con-
tinual learning: a strong, simple baseline. Advances
in neural information processing systems, 33:15920–
15930.
Chaudhry, A., Ranzato, M., Rohrbach, M., and Elhoseiny,
M. (2019). Efficient lifelong learning with A-GEM.
In 7th International Conference on Learning Repre-
sentations, ICLR 2019, New Orleans, LA, USA, May
6-9, 2019. OpenReview.net.
Chen, H.-J., Cheng, A.-C., Juan, D.-C., Wei, W., and
Sun, M. (2020). Mitigating forgetting in online con-
tinual learning via instance-aware parameterization.
Advances in Neural Information Processing Systems,
33:17466–17477.
Collier, M., Kokiopoulou, E., Gesmundo, A., and Berent, J.
(2020). Routing networks with co-training for contin-
ual learning. arXiv preprint arXiv:2009.04381.
Davari, M., Asadi, N., Mudur, S., Aljundi, R., and
Belilovsky, E. (2022). Probing representation forget-
ting in supervised and unsupervised continual learn-
ing. arXiv preprint arXiv:2203.13381.
Delange, M., Aljundi, R., Masana, M., Parisot, S., Jia,
X., Leonardis, A., Slabaugh, G., and Tuytelaars, T.
(2021). A continual learning survey: Defying forget-
ting in classification tasks. IEEE Transactions on Pat-
tern Analysis and Machine Intelligence.
Faisal, A. A., Selen, L. P., and Wolpert, D. M. (2008). Noise
in the nervous system. Nature reviews neuroscience,
9(4):292–303.
Farquhar, S. and Gal, Y. (2018). Towards Robust Evalua-
tions of Continual Learning. Lifelong Learning: A Re-
inforcement Learning Approach Workshop at ICML.
French, R. M. (1999). Catastrophic forgetting in con-
nectionist networks. Trends in cognitive sciences,
3(4):128–135.
Goyal, A. and Bengio, Y. (2020). Inductive biases for
deep learning of higher-level cognition. arXiv preprint
arXiv:2011.15091.
Graham, D. J. and Field, D. J. (2006). Sparse coding in the
neocortex. Evolution of nervous systems, 3:181–187.
Greenwald, N. F., Miller, G., Moen, E., Kong, A., Kagel,
A., Dougherty, T., Fullaway, C. C., McIntosh, B. J.,
Leow, K. X., Schwartz, M. S., et al. (2021). Whole-
cell segmentation of tissue images with human-level
performance using large-scale data annotation and
deep learning. Nature biotechnology, pages 1–11.
Hadsell, R., Rao, D., Rusu, A. A., and Pascanu, R. (2020).
Embracing change: Continual learning in deep neural
networks. Trends in cognitive sciences, 24(12):1028–
1040.
He, K., Zhang, X., Ren, S., and Sun, J. (2016). Deep resid-
ual learning for image recognition. In Proceedings of
the IEEE conference on computer vision and pattern
recognition, pages 770–778.
Hu, T., Chen, T., Wang, H., and Wang, Z. (2020). Triple
wins: Boosting accuracy, robustness and efficiency to-
gether by enabling input-adaptive inference. In ICLR.
Isele, D. and Cosgun, A. (2018). Selective experience re-
play for lifelong learning. In Proceedings of the AAAI
Conference on Artificial Intelligence, volume 32.
Kirkpatrick, J., Pascanu, R., Rabinowitz, N., Veness, J.,
Desjardins, G., Rusu, A. A., Milan, K., Quan, J.,
Ramalho, T., Grabska-Barwinska, A., et al. (2017).
Overcoming catastrophic forgetting in neural net-
works. Proceedings of the national academy of sci-
ences, 114(13):3521–3526.
LeCun, Y., Bottou, L., Bengio, Y., and Haffner, P. (1998).
Gradient-based learning applied to document recogni-
tion. Proceedings of the IEEE, 86(11):2278–2324.
Li, X., Liu, Z., Luo, P., Change Loy, C., and Tang, X.
(2017). Not all pixels are equal: Difficulty-aware se-
mantic segmentation via deep layer cascade. In Pro-
ceedings of the IEEE conference on computer vision
and pattern recognition, pages 3193–3202.
McCloskey, M. and Cohen, N. J. (1989). Catastrophic in-
terference in connectionist networks: The sequential
learning problem. In Psychology of learning and mo-
tivation, volume 24, pages 109–165. Elsevier.
Meunier, D., Lambiotte, R., and Bullmore, E. T. (2010).
Modular and hierarchically modular organization of
brain networks. Frontiers in neuroscience, 4:200.
Netzer, Y., Wang, T., Coates, A., Bissacco, A., Wu, B., and
Ng, A. Y. (2011). Reading digits in natural images
with unsupervised feature learning.
Nose, Y., Kojima, A., Kawabata, H., and Hironaka, T.
(2019). A study on a lane keeping system using cnn
for online learning of steering control from real time
images. In 2019 34th International Technical Confer-
ence on Circuits/Systems, Computers and Communi-
cations (ITC-CSCC), pages 1–4. IEEE.
Ostapenko, O., Rodriguez, P., Caccia, M., and Charlin, L.
(2021). Continual learning via local module composi-
tion. Advances in Neural Information Processing Sys-
tems, 34:30298–30312.
VISAPP 2023 - 18th International Conference on Computer Vision Theory and Applications
270