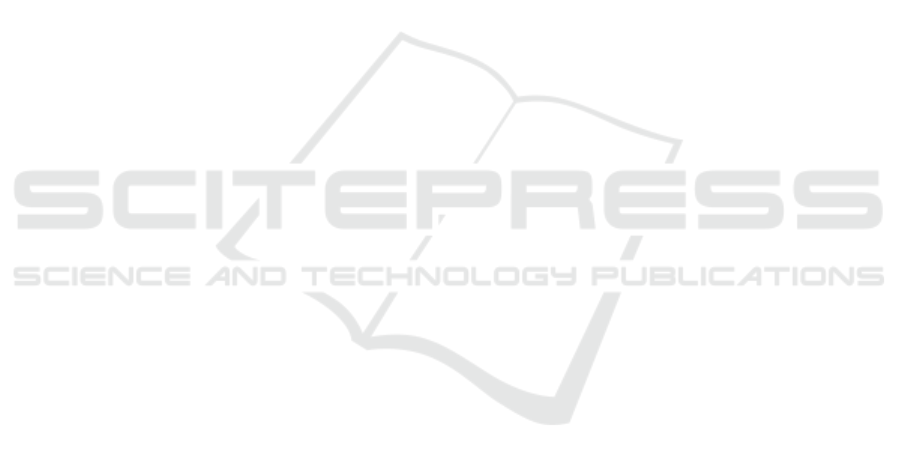
mance needs to be studied in more detail. In partic-
ular, what compromises are needed to run the NN in
embedded platforms with limited memory. In conclu-
sion, the preliminary results obtained in the present
article provide a solid foundation to many paths of
further improvements and system development.
REFERENCES
Almeida, J., Cruz, G., and Oliveira, T. (2022). Foreign Ob-
ject Debris at Aerodromes.
ATSB (2010). Ground operations occurrences at australian
airports 1998 to 2008 (safety report no. 42). Technical
Report 42, Australian Transport Safety Bureau.
Cao, X., Wang, P., Meng, C., Bai, X., Gong, G., Liu, M.,
and Qi, J. (2018). Region based cnn for foreign ob-
ject debris detection on airfield pavement. Sensors,
18(3):737.
Chen, L.-C., Papandreou, G., Schroff, F., and Adam,
H. (2017). Rethinking atrous convolution for
semantic image segmentation. arXiv preprint
arXiv:1706.05587.
Chollet, F. (2017). Xception: Deep learning with depthwise
separable convolutions. In Proceedings of the IEEE
conference on computer vision and pattern recogni-
tion, pages 1251–1258.
Deng, J., Dong, W., Socher, R., Li, L.-J., Li, K., and Fei-
Fei, L. (2009). Imagenet: A large-scale hierarchical
image database. In 2009 IEEE conference on com-
puter vision and pattern recognition, pages 248–255.
Ieee.
FAA (2009). AC 150/5220-24 - Foreign Object Debris De-
tection Equipment – Document Information.
FAA (2018). Faa reauthorization bill 2018 foreign object
debris (fod) detection technology.
Han, Z., Fang, Y., and Xu, H. (2015a). Fusion of low-level
feature for fod classification. In 2015 10th Interna-
tional Conference on Communications and Network-
ing in China (ChinaCom), pages 465–469. IEEE.
Han, Z., Fang, Y., Xu, H., and Zheng, Y. (2015b). A
novel fod classification system based on visual fea-
tures. In International Conference on Image and
Graphics, pages 288–296. Springer.
He, K., Zhang, X., Ren, S., and Sun, J. (2016). Deep resid-
ual learning for image recognition. In Proceedings of
the IEEE conference on computer vision and pattern
recognition, pages 770–778.
Herricks, E. E., Mayer, D., and Majumdar, S. (2015). For-
eign object debris characterization at a large interna-
tional airport. Technical report.
Huang, T. S. (1996). Computer vision: Evolution and
promise. In 19th CERN School of Computing
(CSC’19), pages 25–21. CERN.
Kraus, D. and Watson, J. (2001). Guidelines for the
Prevention an Elimination of Foreign Object Dam-
age/Debris (FOD) in the Aviation Maintenance En-
vironment through Improved Human Performance.
Technical report, Federal Aviation Administration -
Flight Standards Service - Aircraft Maintenance Di-
vision.
Lakshmanan, V., G
¨
orner, M., and Gillard, R. (2021). Prac-
tical Machine Learning for Computer Vision. ”
O’Reilly Media, Inc.”.
Li, P. and Li, H. (2020). Research on fod detection for air-
port runway based on yolov3. In 2020 39th Chinese
Control Conference (CCC), pages 7096–7099. IEEE.
Lin, T.-Y., Maire, M., Belongie, S., Hays, J., Perona, P.,
Ramanan, D., Doll
´
ar, P., and Zitnick, C. L. (2014).
Microsoft coco: Common objects in context. In Euro-
pean conference on computer vision, pages 740–755.
Springer.
Liu, Y., Li, Y., Liu, J., Peng, X., Zhou, Y., and Murphey,
Y. L. (2018). Fod detection using densenet with fo-
cal loss of object samples for airport runway. In
2018 IEEE Symposium Series on Computational In-
telligence (SSCI), pages 547–554. IEEE.
McCreary, I. (2010). Runway safety: Fod, birds and the
case for automated scanning. Insight SRI, pages 146–
157.
Munyer, T., Huang, P.-C., Huang, C., and Zhong, X. (2021).
Fod-a: A dataset for foreign object debris in airports.
arXiv preprint arXiv:2110.03072.
PoAF (2018). Programa de prevenc¸
˜
ao de danos por objetos
estranhos. Technical Report MBA5 330-3 (A), Por-
tuguese Air Force.
Redmon, J. and Farhadi, A. (2018). Yolov3: An incremental
improvement. arXiv preprint arXiv:1804.02767.
Ronneberger, O., Fischer, P., and Brox, T. (2015). U-net:
Convolutional networks for biomedical image seg-
mentation. In International Conference on Medical
image computing and computer-assisted intervention,
pages 234–241. Springer.
Shapiro, L. G. (2020). Computer vision: the last 50 years.
International Journal of Parallel, Emergent and Dis-
tributed Systems, 35(2):112–117.
Warren, J., Gorton, C., Hoff, S., and Alby, F. (2005). Best
practices for the mitigation and control of foreign ob-
ject damage-induced high cycle fatigue in gas tur-
bine engine compression system airfoils. Annex B Ef-
fects of Sand and Dust on Small Gas Turbine Engines,
NATO RTO Applied Vehicle Technology Panel (AVT)
Task Group-094.
Xiao, J., Hays, J., Ehinger, K. A., Oliva, A., and Torralba,
A. (2010). Sun database: Large-scale scene recogni-
tion from abbey to zoo. In 2010 IEEE computer soci-
ety conference on computer vision and pattern recog-
nition, pages 3485–3492. IEEE.
Application of Deep Learning to the Detection of Foreign Object Debris at Aerodromes’ Movement Area
821