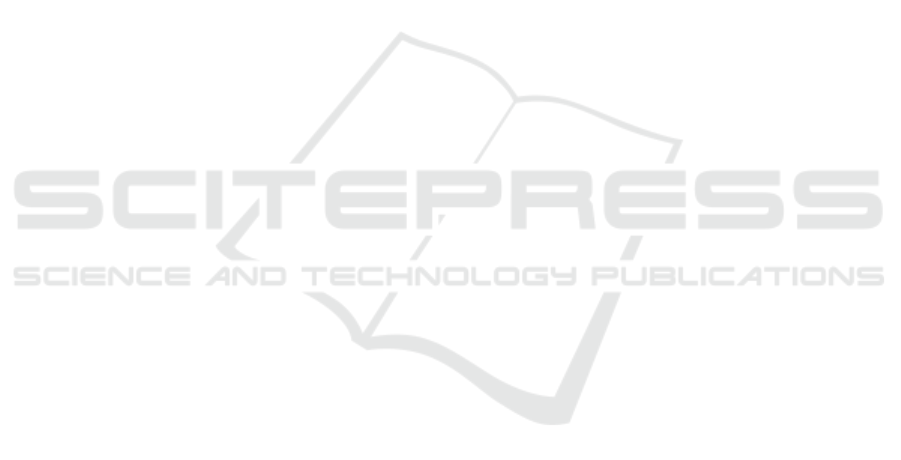
are applied.
6 CONCLUSION AND FUTURE
WORK
This study proposes a patch-based CNN model train-
ing technique to classify breast mammograms into be-
nign or malignant categories and test on a publicly
available dataset of mammograms, CBIS-DDSM,
which was used to classify cancerous and non-
cancerous regions. Our proposed system extracts
overlapping patches using the Overlapping Patch Ex-
traction method, and we compare them with the Non-
Overlapping Patch Extraction approach and Region-
Based-Extraction approach, which is state-of-the-art.
The state-of-the-art approach downsizes the images,
which may result in the loss of discriminative fea-
tures. However, full-size images are used in this work
for patch extraction. The patches are labelled based
on the threshold of ROI using the segmented masks.
The latest CNN models are explored to test the per-
formance of the proposed technique. In our suggested
Overlapping method, whole images are scanned using
the sliding window approach, and a patch database
is created for the training. The best results are ob-
tained using an augmented version of our proposed
approach, the Overlapping Patch Extraction method
trained on the EfficentNet-V2L architecture revealing
an AUC of 0.90.
In the future, a density-based patch extraction
technique can extract more informative patches that
help improve the model’s performance. Moreover,
Generative Adversarial Networks (GANS) can be
used to generate more synthetic data that can directly
contribute towards the successful training of DL mod-
els.
ACKNOWLEDGEMENTS
This work was conducted with the financial support of
the Science Foundation Ireland Centre for Research
Training in Artificial Intelligence under Grant No.
18/CRT/6223. Moreover, we would like to thank
Naveed Shahid and Allan de Lima for their immense
support.
REFERENCES
Agarwal, R., Diaz, O., Llad
´
o, X., Yap, M. H., and Mart
´
ı, R.
(2019). Automatic mass detection in mammograms
using deep convolutional neural networks. Journal of
Medical Imaging, 6(3):031409.
Al-Antari, M. A., Han, S.-M., and Kim, T.-S. (2020). Eval-
uation of deep learning detection and classification to-
wards computer-aided diagnosis of breast lesions in
digital x-ray mammograms. Computer methods and
programs in biomedicine, 196:105584.
El Houby, E. M. and Yassin, N. I. (2021). Malig-
nant and nonmalignant classification of breast le-
sions in mammograms using convolutional neural net-
works. Biomedical Signal Processing and Control,
70:102954.
Keskar, N. S., Mudigere, D., Nocedal, J., Smelyanskiy, M.,
and Tang, P. T. P. (2016). On large-batch training for
deep learning: Generalization gap and sharp minima.
arXiv preprint arXiv:1609.04836.
Lee, R. S., Gimenez, F., Hoogi, A., Miyake, K. K., Gorovoy,
M., and Rubin, D. L. (2017). A curated mammogra-
phy data set for use in computer-aided detection and
diagnosis research. Scientific data, 4(1):1–9.
Mahmood, T., Li, J., Pei, Y., Akhtar, F., Rehman, M. U.,
and Wasti, S. H. (2022). Breast lesions classifica-
tions of mammographic images using a deep convo-
lutional neural network-based approach. Plos one,
17(1):e0263126.
Otsu, N. (1979). A threshold selection method from gray-
level histograms. IEEE transactions on systems, man,
and cybernetics, 9(1):62–66.
Petrini, D. G., Shimizu, C., Roela, R. A., Valente, G. V.,
Folgueira, M. A. A. K., and Kim, H. Y. (2022).
Breast cancer diagnosis in two-view mammography
using end-to-end trained efficientnet-based convolu-
tional network. Ieee Access, 10:77723–77731.
Shen, L., Margolies, L. R., Rothstein, J. H., Fluder, E.,
McBride, R., and Sieh, W. (2019). Deep learning to
improve breast cancer detection on screening mam-
mography. Scientific reports, 9(1):1–12.
Soulami, K. B., Kaabouch, N., Saidi, M. N., and Tamtaoui,
A. (2021). Breast cancer: One-stage automated detec-
tion, segmentation, and classification of digital mam-
mograms using unet model based-semantic segmen-
tation. Biomedical Signal Processing and Control,
66:102481.
Yu, X., Pang, W., Xu, Q., and Liang, M. (2020). Mammo-
graphic image classification with deep fusion learn-
ing. Scientific Reports, 10(1):1–11.
Zahoor, S., Shoaib, U., and Lali, I. U. (2022). Breast can-
cer mammograms classification using deep neural net-
work and entropy-controlled whale optimization algo-
rithm. Diagnostics, 12(2):557.
ICAART 2023 - 15th International Conference on Agents and Artificial Intelligence
876