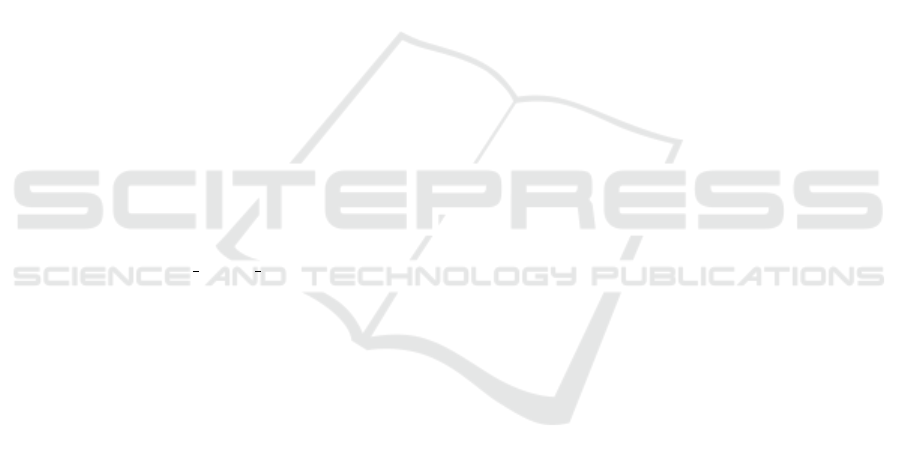
5 CONCLUSIONS
In this paper, ‘You Can Dance’ , an automatic frame-
work for generating music-conditioned dances on real
3D scans, is proposed. The system is composed of
two main modules. The first one generates dance
motions that are coherent with a given music. The
second translates the generated motion dances to real
3D scans using SMPL body model fitting. The fit-
ting module has also been used to generate dancing
3D scans by translating the dance motions of AIST++
dataset to the real 3D scans of the 3DBodyTex.v2
dataset. A human-based evaluation study was con-
ducted to assess the quality of the animated 3D scans
when using dance motions performed by experts and
generated by the music-conditioned dance module.
The proposed framework achieved plausible qualita-
tive results, but had some limitations that are mainly
due to inaccurate 3D scan fitting and unrealistic gen-
erated dance motions. Nevertheless, the authors be-
lieve that this first attempt towards music-conditioned
dance animation of 3D scans might open the doors for
the community to investigate it further.
ACKNOWLEDGEMENTS
This work was funded by the National Research
Fund (FNR), Luxembourg in the context of the PSP-
F2018/12856451/Smart Schoul 2025 project and by
the Esch22 project entitled Sound of data. The au-
thors are grateful to the contributors of the open
source libraries used in this work.
REFERENCES
Aksan, E., Kaufmann, M., Cao, P., and Hilliges, O. (2021).
A spatio-temporal transformer for 3d human motion
prediction. In 2021 International Conference on 3D
Vision (3DV), pages 565–574. IEEE.
Dodik, A., Sell
´
an, S., Kim, T., and Phillips, A. (2022). Sex
and gender in the computer graphics literature. ACM
SIGGRAPH Talks.
Hernandez-Olivan, C. and Beltran, J. R. (2021). Music
composition with deep learning: A review. arXiv
preprint arXiv:2108.12290.
Huang, R., Hu, H., Wu, W., Sawada, K., Zhang, M., and
Jiang, D. (2020). Dance revolution: Long-term dance
generation with music via curriculum learning. arXiv
preprint arXiv:2006.06119.
Huang, Y., Zhang, J., Liu, S., Bao, Q., Zeng, D., Chen,
Z., and Liu, W. (2022). Genre-conditioned long-term
3d dance generation driven by music. In ICASSP
2022-2022 IEEE International Conference on Acous-
tics, Speech and Signal Processing (ICASSP), pages
4858–4862. IEEE.
K
¨
arki, K. (2021). Vocaloid liveness? hatsune miku and the
live production of japanese virtual idol concerts. In
Researching Live Music, pages 127–140. Focal Press.
Lee, H.-Y., Yang, X., Liu, M.-Y., Wang, T.-C., Lu, Y.-D.,
Yang, M.-H., and Kautz, J. (2019). Dancing to music.
Advances in neural information processing systems,
32.
Li, R., Yang, S., Ross, D. A., and Kanazawa, A. (2021).
Learn to dance with aist++: Music conditioned 3d
dance generation.
Loper, M., Mahmood, N., Romero, J., Pons-Moll, G., and
Black, M. J. (2015). SMPL: A skinned multi-person
linear model. ACM Trans. Graphics (Proc. SIG-
GRAPH Asia), 34(6):248:1–248:16.
Pavlakos, G., Choutas, V., Ghorbani, N., Bolkart, T., Os-
man, A. A. A., Tzionas, D., and Black, M. J. (2019).
Expressive body capture: 3D hands, face, and body
from a single image. In Proceedings IEEE Conf. on
Computer Vision and Pattern Recognition (CVPR).
Pu, J. and Shan, Y. (2022). Music-driven dance regeneration
with controllable key pose constraints. arXiv preprint
arXiv:2207.03682.
Ramesh, A., Dhariwal, P., Nichol, A., Chu, C., and
Chen, M. (2022). Hierarchical text-conditional im-
age generation with clip latents. arXiv preprint
arXiv:2204.06125.
Saint, A., Ahmed, E., Cherenkova, K., Gusev, G., Aouada,
D., Ottersten, B., et al. (2018). 3dbodytex: Textured
3d body dataset. In 2018 International Conference on
3D Vision (3DV), pages 495–504. IEEE.
Saint, A., Kacem, A., Cherenkova, K., and Aouada, D.
(2020a). 3dbooster: 3d body shape and texture re-
covery. In European Conference on Computer Vision,
pages 726–740. Springer.
Saint, A., Kacem, A., Cherenkova, K., Papadopoulos, K.,
Chibane, J., Pons-Moll, G., Gusev, G., Fofi, D.,
Aouada, D., and Ottersten, B. (2020b). Sharp 2020:
The 1st shape recovery from partial textured 3d scans
challenge results. In European Conference on Com-
puter Vision, pages 741–755. Springer.
Saint, A., Rahman Shabayek, A. E., Cherenkova, K., Gu-
sev, G., Aouada, D., and Ottersten, B. (2019). Body-
fitr: Robust automatic 3d human body fitting. In 2019
IEEE International Conference on Image Processing
(ICIP).
Siyao, L., Yu, W., Gu, T., Lin, C., Wang, Q., Qian, C., Loy,
C. C., and Liu, Z. (2022). Bailando: 3d dance gener-
ation by actor-critic gpt with choreographic memory.
In Proceedings of the IEEE/CVF Conference on Com-
puter Vision and Pattern Recognition (CVPR), pages
11050–11059.
Sun, G., Wong, Y., Cheng, Z., Kankanhalli, M. S., Geng,
W., and Li, X. (2020). Deepdance: music-to-dance
motion choreography with adversarial learning. IEEE
Transactions on Multimedia, 23:497–509.
Tang, T., Jia, J., and Mao, H. (2018a). Dance with
melody: An lstm-autoencoder approach to music-
oriented dance synthesis. In 2018 ACM Multimedia
You Can Dance! Generating Music-Conditioned Dances on Real 3D Scans
465