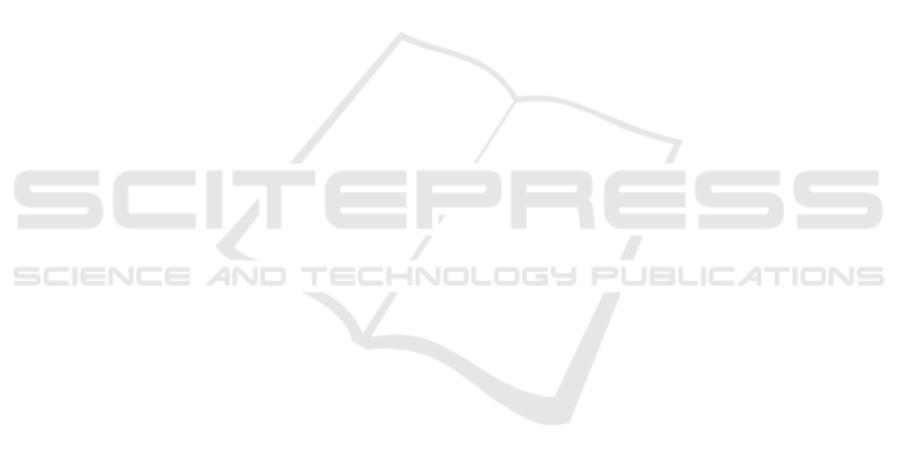
ifying the semantics of sensors, observations, sam-
pling, and actuation. Semantic Web, 10(1):9–32.
Hausknecht, M. and Stone, P. (2015). Deep recurrent q-
learning for partially observable MDPs. In AAAi Fall
Symposium Series.
Horrocks, I., Patel-Schneider, P. F., Boley, H., Tabet, S.,
Grosof, B., Dean, M., et al. (2004). SWRL: A seman-
tic web rule language combining OWL and RuleML.
W3C Member Submission, 21(79):1–31.
Igl, M., Zintgraf, L., Le, T. A., Wood, F., and White-
son, S. (2018). Deep variational reinforcement learn-
ing for POMDPs. In Proceedings of the 35th Inter-
national Conference on Machine Learning (ICML),
pages 2117–2126, Vienna. PMLR.
Kuhnle, A. (2020). Simulation and reinforcement learn-
ing framework for production planning and control of
complex job shop manufacturing systems. Accessed:
2021-06-01.
Kuhnle, A., R
¨
ohrig, N., and Lanza, G. (2019). Autonomous
order dispatching in the semiconductor industry using
reinforcement learning. Procedia CIRP, 79:391–396.
Kulmanov, M., Smaili, F. Z., Gao, X., and Hoehndorf,
R. (2021). Semantic similarity and machine learn-
ing with ontologies. Briefings in Bioinformatics,
22(4):bbaa199.
Le, T. P., Vien, N. A., and Chung, T. (2018). A deep hier-
archical reinforcement learning algorithm in partially
observable markov decision processes. IEEE Access,
6:49089–49102.
Mnih, V., Badia, A. P., Mirza, M., Graves, A., Lillicrap, T.,
Harley, T., Silver, D., and Kavukcuoglu, K. (2016).
Asynchronous methods for deep reinforcement learn-
ing. In International Conference on Machine Learn-
ing, pages 1928–1937. PMLR.
Motta, J. A., Capus, L., and Tourigny, N. (2016). Vence: A
new machine learning method enhanced by ontologi-
cal knowledge to extract summaries. In Proceedings
of the Science and Information Conferences (SAI),
Computing Conference, pages 61–70. IEEE.
Musen, M. A. (2015). The prot
´
eg
´
e project: A look back and
a look forward. AI Matters, 1(4):4–12.
Noy, N. F., McGuinness, D. L., et al. (2001). Ontology
development 101: A guide to creating your first ontol-
ogy. Technical report, Stanford Knowledge Systems
Laboratory.
Oh, J., Chockalingam, V., Lee, H., et al. (2016). Control of
memory, active perception, and action in minecraft.
In International Conference on Machine Learning,
pages 2790–2799. PMLR.
Parisotto, E. and Salakhutdinov, R. (2018). Neural map:
Structured memory for deep reinforcement learning.
In Proceedings of the 6th International Conference on
Learning Representations (ICLR), Vancouver. Open-
Review.net.
Pfitzer, F., Provost, J., Mieth, C., and Liertz, W. (2018).
Event-driven production rescheduling in job shop en-
vironments. In International Conference on Automa-
tion Science and Engineering (CASE), pages 939–
944. IEEE.
Schulman, J., Levine, S., Abbeel, P., Jordan, M., and
Moritz, P. (2015). Trust region policy optimization.
In International Conference on Machine Learning
(ICML), pages 1889–1897. PMLR.
Stankovic, M., Krcadinac, U., Kovanovic, V., and Jo-
vanovic, J. (2009). Intelligent software agents and
multi-agent systems. In Encyclopedia of Information
Science and Technology, Second Edition, pages 2126–
2131. IGI Global.
Sutton, R. S. and Barto, A. G. (2018). Reinforcement learn-
ing: An introduction. MIT Press.
Waschneck, B., Altenm
¨
uller, T., Bauernhansl, T., and Kyek,
A. (2016). Production scheduling in complex job
shops from an industry 4.0 perspective: A review and
challenges in the semiconductor industry. SAMI@
iKNOW, pages 1–12.
Wooldridge, M. (2009). An introduction to multiagent sys-
tems, pages 23–26. John Wiley & Sons.
Xue, X., Wu, X., Jiang, C., Mao, G., and Zhu, H. (2021).
Integrating sensor ontologies with global and local
alignment extractions. Wireless Communications and
Mobile Computing, 2021.
Youn, S. and McLeod, D. (2007). Efficient spam email fil-
tering using adaptive ontology. In Proceedings of the
4th International Conference on Information Technol-
ogy (ITNG), pages 249–254, Las Vegas. IEEE.
Zablith, F., Antoniou, G., d’Aquin, M., Flouris, G., Kondy-
lakis, H., Motta, E., Plexousakis, D., and Sabou,
M. (2015). Ontology evolution: A process-centric
survey. The Knowledge Engineering Review (KER),
30(1):45–75.
Zouaq, A. and Nkambou, R. (2010). A survey of do-
main ontology engineering: Methods and tools. In
Advances in Intelligent Tutoring Systems, pages 103–
119. Springer.
ICAART 2023 - 15th International Conference on Agents and Artificial Intelligence
354