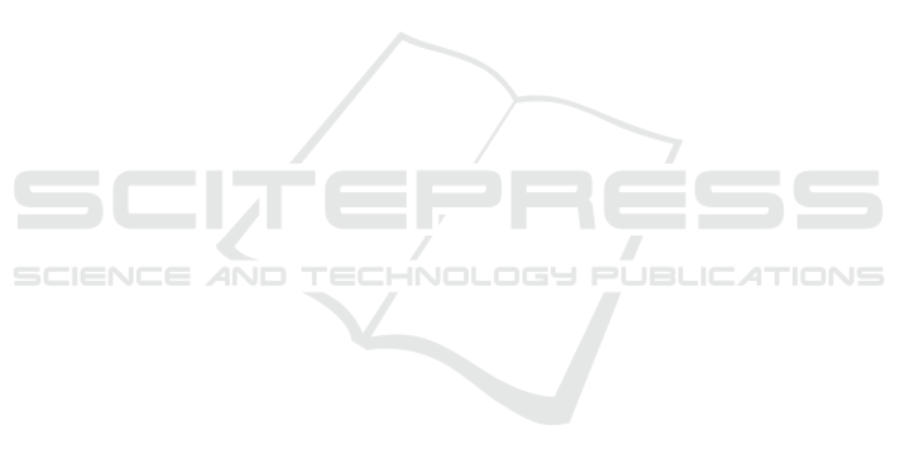
using algorithmic systems. Chaos, Solitons & Frac-
tals, 154:111676.
Huang, J., Li, Y., Suleman, M. T., and Zhang, H. (2021).
Effects of geopolitical risks on gold market return dy-
namics: Evidence from a nonparametric causality-in-
quantiles approach. Defence and Peace Economics,
pages 1–15.
Hundal, B., Grover, S., and Bhatia, J. K. (2013). Herd be-
haviour and gold investment: A perpetual study of re-
tail investors. IOSR Journal of Business and Manage-
ment, 15(4):63–69.
Islam, M. S. and Hossain, E. (2020). Foreign exchange cur-
rency rate prediction using a gru-lstm hybrid network.
Soft Computing Letters, page 100009.
Johnson, T. (2017). The ascendancy of financial economics.
In Ethics in Quantitative Finance, pages 195–220.
Springer.
Kinyua, J. D., Mutigwe, C., Cushing, D. J., and Poggi, M.
(2021). An analysis of the impact of president trump’s
tweets on the djia and s&p 500 using machine learn-
ing and sentiment analysis. Journal of behavioral and
experimental finance, 29:100447.
Liao, S., Chen, J., and Ni, H. (2021). Forex trading volatil-
ity prediction using neuralnetwork models. arXiv
preprint arXiv:2112.01166.
Lim, B., Zohren, S., and Roberts, S. (2019). Population-
based global optimisation methods for learning long-
term dependencies with rnns. arXiv preprint
arXiv:1905.09691.
Madan, I., Saluja, S., and Zhao, A. (2015). Automated
bitcoin trading via machine learning algorithms.
URL: http://cs229. stanford. edu/proj2014/Isaac%
20Madan, 20.
Makala, D. and Li, Z. (2021). Prediction of gold price with
arima and svm. In Journal of Physics: Conference
Series, volume 1767, page 012022. IOP Publishing.
Manjula, K. and Karthikeyan, P. (2019). Gold price pre-
diction using ensemble based machine learning tech-
niques. In 2019 3rd International Conference on
Trends in Electronics and Informatics (ICOEI), pages
1360–1364. IEEE.
McNally, S. (2016). Predicting the price of Bitcoin using
Machine Learning. PhD thesis, Dublin, National Col-
lege of Ireland.
Milke, V., Luca, C., and Wilson, G. (2017). Minimisation
of parameters for optimisation of algorithmic trad-
ing systems. In 2017 International Conference on
Optimization of Electrical and Electronic Equipment
(OPTIM) & 2017 Intl Aegean Conference on Electri-
cal Machines and Power Electronics (ACEMP), pages
1114–1119. IEEE.
Milke, V., Luca, C., Wilson, G., and Fatima, A. (2020).
Using convolutional neural networks and raw data to
model intraday trading market behaviour. In ICAART
(2), pages 224–231.
Nison, S. (2011). Strategies for Profiting with Japanese
Candlestick Charts, volume 132. John Wiley & Sons.
Raimundo, M. S. and Okamoto Jr, J. (2018). Application
of hurst exponent (h) and the r/s analysis in the clas-
sification of forex securities. International Journal of
Modeling and Optimization, 8(2):116–124.
Rundo, F., Trenta, F., di Stallo, A. L., and Battiato, S.
(2019). Grid trading system robot (gtsbot): A novel
mathematical algorithm for trading fx market. Applied
Sciences, 9(9):1796.
Semeyutin, A., Gozgor, G., Lau, C. K. M., and Xu, B.
(2021). Effects of idiosyncratic jumps and co-jumps
on oil, gold, and copper markets. Energy Economics,
104:105660.
Shen, J. (2021). Nine challenges in modern al-
gorithmic trading and controls. arXiv preprint
arXiv:2101.08813.
Siami-Namini, S. and Namin, A. S. (2018). Forecasting
economics and financial time series: Arima vs. lstm.
arXiv preprint arXiv:1803.06386.
U.S. Securities and Exchange Commission (2022).
Day trading. Online: https://www.investor.gov/
introduction-investing/investing-basics/glossary/
day-trading. Accessed: 2022-11-20.
Vargas, M. R., De Lima, B. S., and Evsukoff, A. G. (2017).
Deep learning for stock market prediction from finan-
cial news articles. In 2017 IEEE international con-
ference on computational intelligence and virtual en-
vironments for measurement systems and applications
(CIVEMSA), pages 60–65. IEEE.
Weeraddana, N., Silva, A., and Jayathilake, P. (2018). De-
tection of black regions in the forex market by analyz-
ing high-frequency intraday data. In 2018 18th Inter-
national Conference on Advances in ICT for Emerging
Regions (ICTer), pages 384–391. IEEE.
Weng, F., Chen, Y., Wang, Z., Hou, M., Luo, J., and Tian,
Z. (2020). Gold price forecasting research based on an
improved online extreme learning machine algorithm.
Journal of Ambient Intelligence and Humanized Com-
puting, 11(10):4101–4111.
Zeng, H. and Lu, R. (2022). High-frequency volatility
connectedness and time-frequency correlation among
chinese stock and major commodity markets around
covid-19. Investment Management and Financial In-
novations, 19(2):260–273.
Zhang, W., Yan, K., and Shen, D. (2021). Can the baidu
index predict realized volatility in the chinese stock
market? Financial Innovation, 7(1):1–31.
ICAART 2023 - 15th International Conference on Agents and Artificial Intelligence
892