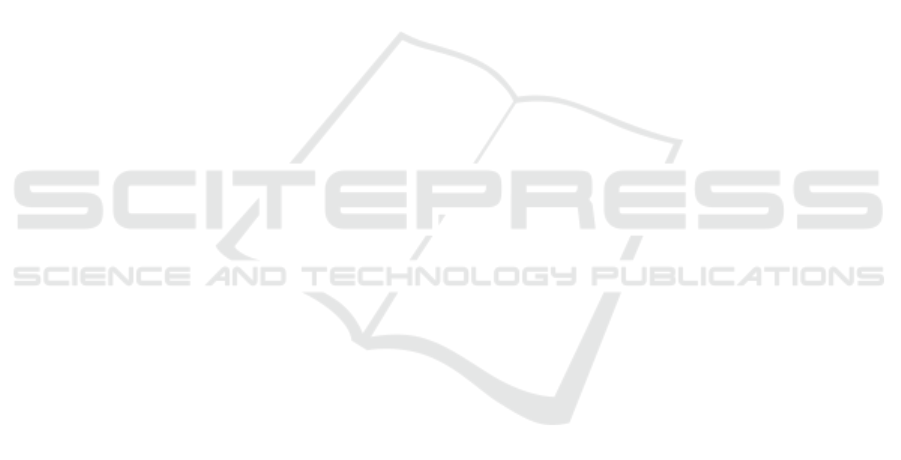
the IEEE conference on computer vision and pattern
recognition, pages 770–778.
Hosgurmath, S., Mallappa, V. V., Patil, N. B., and Petli,
V. (2022). Effective face recognition using dual lin-
ear collaborative discriminant regression classifica-
tion algorithm. Multimedia Tools and Applications,
81(5):6899–6922.
Hu, Y., Xu, Y., Zhuang, H., Weng, Z., and Lin, Z. (2022).
Machine learning techniques and systems for mask-
face detection—survey and a new ood-mask
approach. Applied Sciences, 12(18).
Ittahir, S., Idbeaa, T., and Ogorban, H. (2022). The system
for estimating the number of people in digital images
based on skin color face detection algorithm. AlQalam
Journal of Medical and Applied Sciences, pages 215–
225.
Kanade, T., Cohn, J. F., and Tian, Y. (2000). Comprehen-
sive database for facial expression analysis. In IEEE
Int Conference on Automatic Face and Gesture Recog-
nition, pages 46–53.
Kar, N. B., Babu, K. S., and Bakshi, S. (2022). Facial
expression recognition system based on variational
mode decomposition and whale optimized kelm. Im-
age and Vision Computing, page 104445.
Khanam, R., Johri, P., and Div
´
an, M. J. (2022). Human
Skin Color Detection Technique Using Different Color
Models, pages 261–279.
Lakshmi, D. and Ponnusamy, R. (2021). Facial emotion
recognition using modified hog and lbp features with
deep stacked autoencoders. Microprocessors and Mi-
crosystems, 82:103834.
Li, Z.-J. (2022). A method of improving accuracy in ex-
pression recognition. European Journal of Electrical
Engineering and Computer Science, 6(3):27–30.
Lucey, P., Cohn, J. F., Kanade, T., Saragih, J., Ambadar,
Z., and Matthews, I. (2010). The extended cohn-
kanade dataset (ck+): A complete dataset for action
unit and emotion-specified expression. In 2010 IEEE
conference on computer vision and pattern recogni-
tion, pages 94–101.
Lyons, M., Kamachi, M., and Gyoba, J. The japanese fe-
male facial expression (jaffe) dataset.
Nan, F., Jing, W., Tian, F., Zhang, J., Chao, K.-M., Hong,
Z., and Zheng, Q. (2022). Feature super-resolution
based Facial Expression Recognition for multi-scale
low-resolution images. Knowledge-Based Systems,
236:107678.
Nugroho, H. A., Goratama, R. D., and Frannita, E. L.
(2021). Face recognition in four types of colour space:
a performance analysis. In Materials Science and En-
gineering, volume 1088, page 012010.
Putra, I., Wiastini, N., Wibawa, K. S., and Putra, I. M. S.
(2020). Identification of skin disease using k-means
clustering, discrete wavelet transform, color moments
and support vector machine. Int J. Mach. Learn. Com-
put, 10(5):700–706.
Rahman, M. A., Purnama, I. K. E., and Purnomo, M. H.
(2014). Simple method of human skin detection using
hsv and ycbcr color spaces. In 2014 Int Conference
on Intelligent Autonomous Agents, Networks and Sys-
tems, pages 58–61.
Ruan, D., Mo, R., Yan, Y., Chen, S., Xue, J.-H., and Wang,
H. (2022). Adaptive deep disturbance-disentangled
learning for facial expression recognition. Int Journal
of Computer Vision, 130(2):455–477.
Sadeghi, H. and Raie, A.-A. (2022). Histnet: Histogram-
based convolutional neural network with chi-squared
deep metric learning for facial expression recognition.
Information Sciences, 608:472–488.
Saurav, S., Gidde, P., Saini, R., and Singh, S. (2022). Dual
integrated convolutional neural network for real-time
facial expression recognition in the wild. The Visual
Computer, 38(3):1083–1096.
Siam, A. I., Soliman, N. F., Algarni, A. D., El-Samie,
A., Fathi, E., and Sedik, A. (2022). Deploying ma-
chine learning techniques for human emotion detec-
tion. Computational Intelligence and Neuroscience,
2022:8032673.
Sidhom, O., Ghazouani, H., and Barhoumi, W. (2023).
Subject-dependent selection of geometrical features
for spontaneous emotion recognition. Multimedia
Tools and Applications, 82(2):2635–2661.
Simonyan, K. and Zisserman, A. (2014). Very deep con-
volutional networks for large-scale image recognition.
arXiv preprint arXiv:1409.1556.
Szegedy, C., Liu, W., Jia, Y., Sermanet, P., Reed, S.,
Anguelov, D., Erhan, D., Vanhoucke, V., and Rabi-
novich, A. (2015). Going deeper with convolutions.
In Proceedings of the IEEE conference on computer
vision and pattern recognition, pages 1–9.
Terrillon, J.-C., Shirazi, M. N., Fukamachi, H., and Aka-
matsu, S. (2000). Comparative performance of differ-
ent skin chrominance models and chrominance spaces
for the automatic detection of human faces in color
images. In 4th IEEE Int Conference on Automatic
Face and Gesture Recognition, pages 54–61.
Vansh, V., Chandrasekhar, K., Anil, C. R., and Sahu, S. S.
(2020). Improved face detection using ycbcr and
adaboost. In Behera, H. S., Nayak, J., Naik, B.,
and Pelusi, D., editors, Computational Intelligence in
Data Mining.
Viola, P. and Jones, M. (2001). Rapid object detection using
a boosted cascade of simple features. In Proceedings
of the 2001 conference on computer vision and pattern
recognition. CVPR 2001, volume 1, pages I–I.
Yamada, Y., Inagawa, T., Hirabayashi, N., and Sumiyoshi,
T. (2022). Emotion recognition deficits in psychiatric
disorders as a target of non-invasive neuromodulation:
A systematic review. Clinical EEG and Neuroscience,
53(6):506–512.
Yan, H., Liu, Y., Wang, X., Li, M., and Li, H. (2021). A face
detection method based on skin color features and ad-
aboost algorithm. In Journal of Physics: Conference
Series, volume 1748, page 042015.
Zhu, Q., Mao, Q., Jia, H., Noi, O. E. N., and Tu, J. (2022).
Convolutional relation network for facial expression
recognition in the wild with few-shot learning. Expert
Systems with Applications, 189:116046.
YCbCr Color Space as an Effective Solution to the Problem of Low Emotion Recognition Rate of Facial Expressions In-The-Wild
829