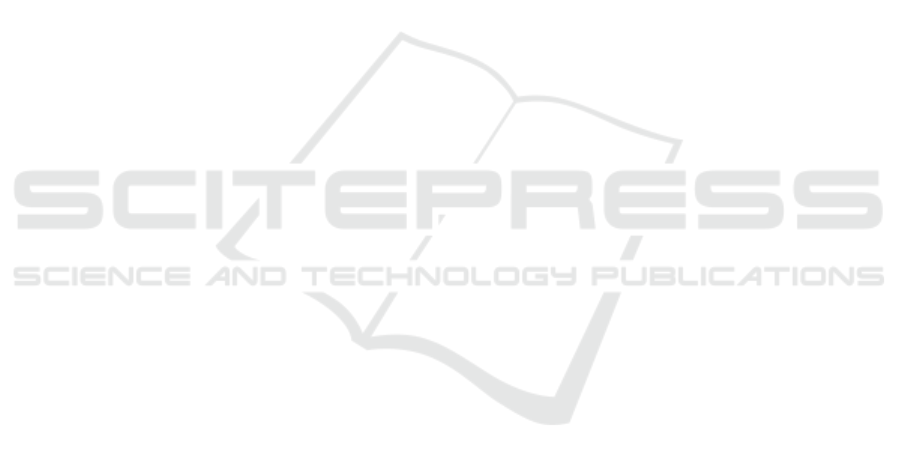
signals recorded during swallowing events. The re-
sults of utilizing only the suprahyoid or infrahyoid
muscles did not differ statistically; however, there
were statistical differences between the various re-
gressors. RF, then SVM regressors were the best ones
using the Mean Absolute Value feature in estimating
the fluid volume with the lowest error. Furthermore,
there is an indication that regressor performance is
feature dependent. This outcome is a step forward
in using sEMG for hydration monitoring. Further re-
search is needed to investigate the use of single EMG
channels to record and estimate the fluid data and
whether two channels work better for regression and
the other are better for classification.
REFERENCES
El-Sharkawy, A. M., Sahota, O., Maughan, R. J., & Lobo,
D. N. (2014). The pathophysiology of fluid and elec-
trolyte balance in the older adult surgical patient. Clin-
ical Nutrition, 33(1), 6-13.
Dehydration risks for seniors. Cleveland Clinic. (2021,
November 10). Re- trieved December 30, 2021,
from https://health.clevel and clinic.org/drink- up-
dehydration- is-an-often-overlooked-health-risk-for-
seniors/
Malvuccio, C., & Kamavuako, E. N. (2021, March). De-
tection of Swallowing Events and Fluid Intake Vol-
ume Estimation from Surface Electromyography Sig-
nals. In 2020 IEEE-EMBS Conference on Biomedical
Engineering and Sciences (IECBES) (pp. 245-250).
IEEE.
Asogan, H., & Raoof, A. (2021). Education and training as
key drivers for improving the quality of fluid balance
charts: findings from a quality improvement project.
BMJ Open Quality, 10(3), e001137.
Cohen, R., Fernie, G., & Roshan Fekr, A. (2021). Fluid in-
take monitoring systems for the elderly: a review of
the literature. Nutrients, 13(6), 2092.
Wellnitz, A., Wolff, J. P., Haubelt, C., & Kirste, T. (2019,
September). Fluid intake recognition using inertial
sensors. In Proceedings of the 6th international Work-
shop on Sensor-based Activity Recognition and Inter-
action (pp. 1-7).
Dong, B., & Biswas, S. (2017). Meal-time and duration
monitoring using wearable sensors. Biomedical Sig-
nal Processing and Control, 32, 97-109.
Cheng, J., Amft, O., & Lukowicz, P. (2010, May). Ac-
tive capacitive sensing: Exploring a new wearable
sensing modality for activity recognition. In Interna-
tional conference on pervasive computing (pp. 319-
336). Springer, Berlin, Heidelberg.
Cheng, J., Zhou, B., Kunze, K., Rheinl
¨
ander, C. C., Wille,
S., Wehn, N., ... & Lukowicz, P. (2013, September).
Activity recognition and nutrition monitoring in ev-
ery day situations with a textile capacitive neckband.
In Proceedings of the 2013 ACM conference on Per-
vasive and ubiquitous computing adjunct publication
(pp. 155-158).
Zhang, R., Freund, M., Amft, O., Cheng, J., Zhou, B.,
Lukowicz, P., ... & Chabrecek, P. (2016, September).
A generic sensor fabric for multi-modal swallowing
sensing in regular upper-body shirts. In Proceedings
of the 2016 ACM International Symposium on Wear-
able Computers (pp. 46-47).
Amft, O., & Troster, G. (2006, November). Methods for de-
tection and classification of normal swallowing from
muscle activation and sound. In 2006 Pervasive Health
Conference and Workshops (pp. 1-10). IEEE.
Amft, O., & Tr
¨
oster, G. (2008). Recognition of dietary ac-
tivity events using on-body sensors. Artificial intelli-
gence in medicine, 42(2), 121-136.
Moreau–Gaudry, A., Sabil, A., Benchetrit, G., & Franco,
A. (2005). Use of respiratory inductance plethysmog-
raphy for the detection of swallowing in the elderly.
Dysphagia, 20(4), 297-302.
Dong, B., & Biswas, S. (2012, August). Swallow moni-
toring through apnea detection in breathing signal. In
2012 Annual International Conference of the IEEE
Engineering in Medicine and Biology Society (pp.
6341-6344). IEEE.
Dong, B., & Biswas, S. (2013, May). Liquid intake monitor-
ing through breathing signal using machine learning.
In Sensing Technologies for Global Health, Military
Medicine, and Environmental Monitoring III (Vol.
8723, pp. 141-148). SPIE.
Dong, B., & Biswas, S. (2016). Analyzing breathing signals
and swallow sequence locality for solid food intake
monitoring. Journal of medical and biological engi-
neering, 36(6), 765-775.
Tatulli, E., Fontecave-Jallon, J., Calabrese, P., & Gumery,
P. Y. (2020). Respiratory Inductance Plethysmogra-
phy for Automated Swallowing Detection. Interna-
tional Journal of E-Health and Medical Communica-
tions (IJEHMC), 11(2), 64-77.
Nakafuji, H., Imura, M., Uranishi, Y., Yoshimoto, S., & Os-
hiro, O. (2014). Estimation of amount of swallowed
water by analysis of swallowing sounds. Biomedical
Engineering, 52(Supplement), O-11.
Kobayashi, Y., & Mineno, H. (2014, October). Fluid in-
take recognition for nursing care support by leverag-
ing swallowing sound. In 2014 IEEE 3rd Global Con-
ference on Consumer Electronics (GCCE) (pp. 620-
621). IEEE.
Amft, O., & Troster, G. (2006, November). Methods for de-
tection and classification of normal swallowing from
muscle activation and sound. In 2006 Pervasive Health
Conference and Workshops (pp. 1-10). IEEE.
Negi, S., Kumar, Y., & Mishra, V. M. (2016, September).
Feature extraction and classification for EMG signals
using linear discriminant analysis. In 2016 2nd In-
ternational Conference on Advances in Computing,
Communication, & Automation (ICACCA)(Fall) (pp.
1-6). IEEE.
Spiewak, C., Islam, M., Zaman, A., & Rahman, M.
H. (2018). A comprehensive study on EMG feature
extraction and classifiers. Open Access Journal of
Biomedical Engineering and Biosciences, 1(1), 1-10.
Estimation of Fluid Intake Volume from Surface Electromyography Signals: A Comparative Study of Seven Regression Techniques
123