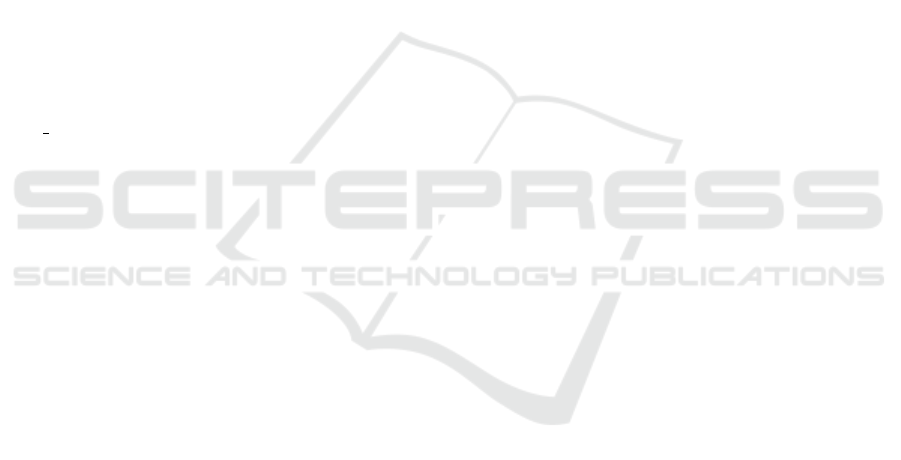
REFERENCES
(2021). What should i know to use eda
data in my experiment? Available on-
line: https://support.empatica.com/hc/en-
us/articles/203621955-What-should-I-know-to-
use-EDA- data-in-my-experiment-. Accessed on 24
November 2022.
Abugabah, A., Nizamuddin, N., and Abuqabbeh, A. (2020).
A review of challenges and barriers implementing rfid
technology in the healthcare sector. Procedia Com-
puter Science, 170:1003–1010. The 11th International
Conference on Ambient Systems, Networks and Tech-
nologies (ANT) / The 3rd International Conference on
Emerging Data and Industry 4.0 (EDI40) / Affiliated
Workshops.
Bacon, S. (2019). Stress, psychiatric disorders, and cardio-
vascular disease. BMJ, 365:l1577.
Bochem, A., Abugabah, A., and Smadi, A. A. (2022). Smart
rfid application in health care: Using rfid technology
for smart inventory and logistic systems in hospitals.
In 2022 45th Jubilee International Convention on In-
formation, Communication and Electronic Technol-
ogy (MIPRO), pages 18–23.
Brun, J. P. Stress: The health epidemic of the 21st century.
Available online: https://www.who.int/occupational\
health/topics/brunpres0307.pdf?ua=1.
Burky, A. (2022). Medical devices are a weak
link in hospital cyber defenses, putting pa-
tients in the crossfire: study. Available on-
line: https://www.fiercehealthcare.com/health-
tech/cyberattack-revolving-doors-medical-devices-
put-patients-crossfire. Accessed on 24 November
2022.
Doynikova, E., Novikova, E., Murenin, I., Kolomeets, M.,
Levshun, D., Tushkanova, O., Levshun, D., Meleshko,
A., and Kotenko, I. (2022). Security Measuring Sys-
tem for IoT Devices, pages 256–275.
D’Souza, A. Your heartbeat may soon be your only
password. Available online: https://www.wired.com/
insights/2014/06/heartbeat-may-soon-password/. Ac-
cessed on 24 November 2022.
Fink, G. (2016). Stress: The health epidemic of the 21st
century. Available online: https://scitechconnect.
elsevier.com/stress-health-epidemic-21st-century/.
Accessed on 24 November 2022.
Hoffman, T. What is heart rate variability (hrv) &
why does it matter?: Firstbeat blog. Avail-
able online: https://www.firstbeat.com/en/blog/
what-is-heart-rate-variability-hrv/. Accessed on 24
November 2022.
Kim, K. H., Bang, S. W., and Kim, S. R. (2006). Emo-
tion recognition system using short-term monitoring
of physiological signals. Medical and Biological En-
gineering and Computing, 42:419–427.
Li, C., Raghunathan, A., and Jha, N. (2011). Hijacking
an insulin pump: Security attacks and defenses for a
diabetes therapy system. In 2011 IEEE 13th Interna-
tional Conference on e-Health Networking, Applica-
tions and Services, HEALTHCOM 2011, 2011 IEEE
13th International Conference on e-Health Network-
ing, Applications and Services, HEALTHCOM 2011,
pages 150–156. 2011 IEEE 13th International Confer-
ence on e-Health Networking, Applications and Ser-
vices, HEALTHCOM 2011 ; Conference date: 13-06-
2011 Through 15-06-2011.
Malik, M., Bigger, J. T., Camm, A. J., E., K. R., Malliani,
A., and Moss, A. J. (1996). Heart rate variability:
standards of measurement, physiological interpreta-
tion, and clinical use. Circulation, 93(5):1043–1065.
Mikuckas, A., Mikuckiene, I., Venckauskas, A., Kazanavi-
cius, E., Lukas, R., and Plauska, I. (2014). Emotion
recognition in human computer interaction systems.
Elektronika ir Elektrotechnika, 20(10):51–56.
Raad, M. W., Deriche, M., and Kanoun, O. (2021). An
rfid-based monitoring and localization system for de-
mentia patients. In 2021 18th International Multi-
Conference on Systems, Signals & Devices (SSD),
pages 1–7.
Radcliffe, J. (2011). Hacking medical devices for fun and
insulin breaking the human scada system. Accessed
on 24 November 2022.
Rajak, M. and Shaw, K. (2021). An extension of technology
acceptance model for mhealth user adoption. Technol-
ogy in Society, 67:101800.
Storm, D. (2011). Black hat: Lethal hack and wireless at-
tack on insulin pumps to kill people. Available online:
https://www.computerworld.com/article/2470689/black-
hat–lethal-hack-and-wireless-attack-on-insulin-
pumps-to-kill-people.html. Accessed on 24 Novem-
ber 2022.
Takahashi, D. (2011). Excuse me while i
turn off your insulin pump. Available
online: https://venturebeat.com/mobile/
excuse-me-while-i-turn-off-your-insulin-pump/.
Accessed on 24 November 2022.
Yaqoob, T., Abbas, H., and Atiquzzaman, M. (2019). Se-
curity vulnerabilities, attacks, countermeasures, and
regulations of networked medical devices—a review.
IEEE Communications Surveys & Tutorials, 21:3723–
3768.
An RFID Based Localization and Mental Stress Recognition System Using Wearable Sensors
331