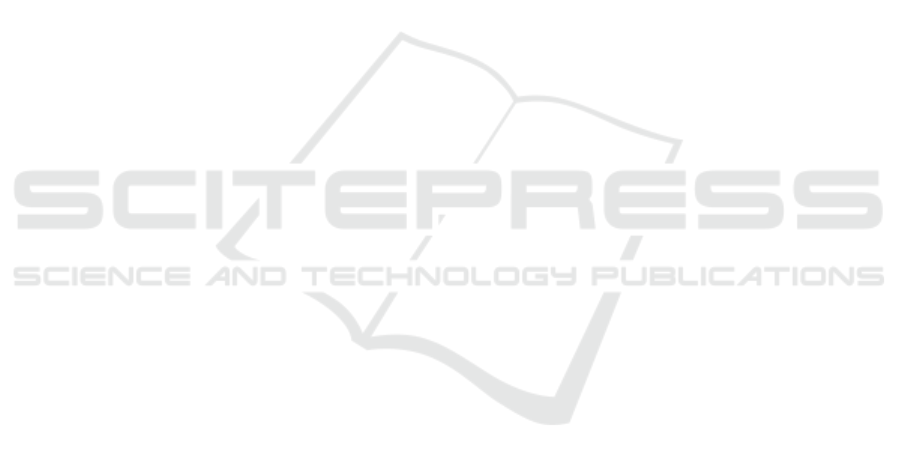
with type 1 diabetes. Journal of Personalized Medicine,
12(8):1262.
Bowyer, K. W., Chawla, N. V., Hall, L. O., and Kegelmeyer,
W. P. (2011). SMOTE: synthetic minority over-sampling
technique. CoRR, abs/1106.1813.
Cengiz, E. and Tamborlane, W. V. (2009). A tale of two com-
partments: Interstitial versus blood glucose monitoring.
Diabetes Technology & Therapeutics, 11(S1):11–16.
Dua, D. and Graff, C. (2017). UCI machine learning reposit-
ory.
Gu, Q., Cai, Z., Zhu, L., and Huang, B. (2008). Data mining
on imbalanced data sets. In 2008 International Confer-
ence on Advanced Computer Theory and Engineering,
pages 1020–1024. IEEE.
Han, H., Wang, W.-Y., and Mao, B.-H. (2005). Borderline-
smote: A new over-sampling method in imbalanced data
sets learning. In Huang, D.-S., Zhang, X.-P., and Huang,
G.-B., editors, Advances in Intelligent Computing, pages
878–887, Berlin, Heidelberg. Springer Berlin Heidelberg.
Hart, P. (1968). The condensed nearest neighbor rule
(corresp.). IEEE Transactions on Information Theory,
14(3):515–516.
He, H., Bai, Y., Garcia, E. A., and Li, S. (2008). ADASYN:
Adaptive synthetic sampling approach for imbalanced
learning. In 2008 IEEE International Joint Conference
on Neural Networks (IEEE World Congress on Computa-
tional Intelligence), pages 1322–1328.
International Diabetes Federation (2019). IDF Diabetes
Atlas, 9th edn. Accessed on: 19/07/2021.
Kubat, M., Matwin, S., et al. (1997). Addressing the curse of
imbalanced training sets: one-sided selection. In ICML,
volume 97, pages 179–186. Nashville, USA.
Laurikkala, J. (2001). Improving identification of difficult
small classes by balancing class distribution. In Quaglini,
S., Barahona, P., and Andreassen, S., editors, Artificial
Intelligence in Medicine, pages 63–66, Berlin, Heidelberg.
Springer Berlin Heidelberg.
Li, D., Liu, C., and Hu, S. C. (2010). A learning method
for the class imbalance problem with medical data sets.
Computers in Biology and Medicine, 40(5):509–518.
Machado, D., Costa, V. S., and Brandão, P. (2022). Im-
pact of the glycaemic sampling method in diabetes data
mining. In 2022 IEEE Symposium on Computers and
Communications (ISCC), pages 1–6.
Mani, I. and Zhang, I. (2003). kNN approach to unbalanced
data distributions: a case study involving information
extraction. In Proceedings of workshop on learning from
imbalanced datasets, volume 126. ICML United States.
Marling, C. and Bunescu, R. (2020). The OhioT1DM data-
set for blood glucose level prediction: Update 2020. In
CEUR workshop proceedings, volume 2675, page 71.
NIH Public Access.
Mayo, M., Chepulis, L., and Paul, R. G. (2019). Glycemic-
aware metrics and oversampling techniques for predicting
blood glucose levels using machine learning. PLOS ONE,
14(12):e0225613.
Medtronic Diabetes (2014). Why sensor glucose does not
equal blood glucose. Accessed on: 29/03/2022.
Mouri, M. and Badireddy, M. (2021). Hyperglycemia. Stat-
Pearls [Internet]. [Updated 2021 May 10].
Nguyen, H. M., Cooper, E. W., and Kamei, K. (2011). Bor-
derline over-sampling for imbalanced data classification.
International Journal of Knowledge Engineering and Soft
Data Paradigms, 3(1):4–21.
Raval, K. M. (2012). Data mining techniques. International
Journal of Advanced Research in Computer Science and
Software Engineering, 2(10).
Seery, C. (2019a). Diabetes complications. guide on dia-
betes.co.uk. Accessed on: 20/07/2021.
Seery, C. (2019b). Short term complications. guide on
diabetes.co.uk. Accessed on: 20/07/2021.
Shukla, D., Patel, S. B., and Sen, A. K. (2014). A literature
review in health informatics using data mining techniques.
International Journal of Software and Hardware Research
in Engineering, 2(2):123–129.
Siegmund, T., Heinemann, L., Kolassa, R., and Thomas,
A. (2017). Discrepancies between blood glucose and in-
terstitial glucose—technological artifacts or physiology:
Implications for selection of the appropriate therapeutic
target. Journal of Diabetes Science and Technology,
11(4):766–772.
Tomek, I. et al. (1976). Two modifications of cnn. IEEE
Transactions on Systems, Man, and Cybernetics, SMC-
6(11):769–772.
Wilson, D. L. (1972). Asymptotic properties of nearest
neighbor rules using edited data. IEEE Transactions on
Systems, Man, and Cybernetics, SMC-2(3):408–421.
HEALTHINF 2023 - 16th International Conference on Health Informatics
198