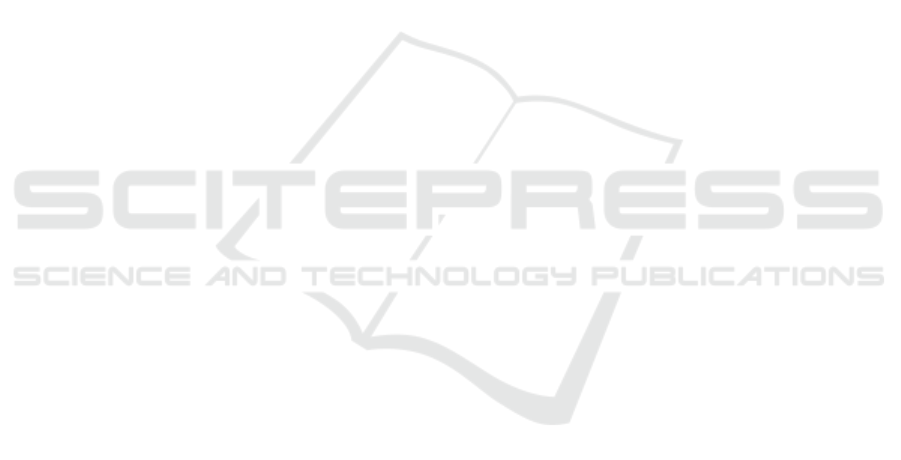
M. (2010). Moa: Massive online analysis. Journal of
Machine Learning Research 11: 1601-1604.
Blackard, J. A., Dean, D. J., and Anderson, C. W. (1998).
Covertype data set.
Bu, L., Alippi, C., and Zhao, D. (2016). A pdf-free change
detection test based on density difference estimation.
IEEE transactions on neural networks and learning
systems, 29(2):324–334.
Ditzler, G., Roveri, M., Alippi, C., and Polikar, R. (2015).
Learning in nonstationary environments: A survey.
IEEE Comp. Int. Mag., 10(4).
Eliades, D. G. and Polycarpou, M. M. (2010). A Fault
Diagnosis and Security Framework for Water Systems.
IEEE Transactions on Control Systems Technology,
18(6):1254–1265.
Elwell, R. and Polikar, R. (2011). Incremental learning of
concept drift in nonstationary environments. IEEE
Transactions on Neural Networks, 22(10):1517–1531.
Frías-Blanco, I., d. Campo-Ávila, J., Ramos-Jiménez, G.,
Morales-Bueno, R., Ortiz-Díaz, A., and Caballero-
Mota, Y. (2015). Online and non-parametric drift de-
tection methods based on hoeffding’s bounds. IEEE
Transactions on Knowledge and Data Engineering,
27(3):810–823.
Gama, J., Medas, P., Castillo, G., and Rodrigues, P. P. (2004).
Learning with drift detection. In Advances in Artificial
Intelligence - SBIA 2004, 17th Brazilian Symposium
on Artificial Intelligence, São Luis, Maranhão, Brazil,
September 29 - October 1, 2004, Proceedings, pages
286–295.
Gama, J. a., Žliobait
˙
e, I., Bifet, A., Pechenizkiy, M., and
Bouchachia, A. (2014). A survey on concept drift
adaptation. ACM Comput. Surv., 46(4):44:1–44:37.
Gonçalves Jr, P. M., de Carvalho Santos, S. G., Barros, R. S.,
and Vieira, D. C. (2014). A comparative study on con-
cept drift detectors. Expert Systems with Applications,
41(18):8144–8156.
Gözüaçık, Ö., Büyükçakır, A., Bonab, H., and Can, F. (2019).
Unsupervised concept drift detection with a discrimi-
native classifier. In Proceedings of the 28th ACM in-
ternational conference on information and knowledge
management, pages 2365–2368.
Gretton, A., Borgwardt, K., Rasch, M., Schölkopf, B., and
Smola, A. (2006). A kernel method for the two-sample-
problem. volume 19.
Hanneke, S., Kanade, V., and Yang, L. (2015). Learning with
a drifting target concept. In Int. Conf. on Alg. Learn.
Theo, pages 149–164. Springer.
Harries, M., cse tr, U. N., and Wales, N. S. (1999). Splice-2
comparative evaluation: Electricity pricing. Technical
report.
Hinder, F., Artelt, A., and Hammer, B. (2019). A probability
theoretic approach to drifting data in continuous time
domains. arXiv preprint arXiv:1912.01969.
Hinder, F., Artelt, A., and Hammer, B. (2020). Towards
non-parametric drift detection via dynamic adapting
window independence drift detection (dawidd). In
ICML.
Hinder, F., Brinkrolf, J., Vaquet, V., and Hammer, B. (2021).
A shape-based method for concept drift detection and
signal denoising. In 2021 IEEE Symposium Series
on Computational Intelligence (SSCI), pages 01–08.
IEEE.
Hinder, F., Vaquet, V., and Hammer, B. (2022). Suitability of
different metric choices for concept drift detection. In
International Symposium on Intelligent Data Analysis,
pages 157–170. Springer.
Hu, H., Kantardzic, M., and Sethi, T. S. (2020). No free
lunch theorem for concept drift detection in streaming
data classification: A review. WIREs Data Mining and
Knowledge Discovery, 10(2):e1327.
Kosina, P. and Gama, J. (2013). Very fast decision rules
for classification in data streams. Data Mining and
Knowledge Discovery, 29:168–202.
Losing, V., Hammer, B., and Wersing, H. (2015). Interactive
online learning for obstacle classification on a mobile
robot. In 2015 international joint conference on neural
networks (ijcnn), pages 1–8. IEEE.
Lu, J., Liu, A., Dong, F., Gu, F., Gama, J., and Zhang, G.
(2018). Learning under concept drift: A review. IEEE
Transactions on Knowledge and Data Engineering,
31(12):2346–2363.
Manapragada, C., Webb, G. I., and Salehi, M. (2018). Ex-
tremely fast decision tree. In Proceedings of the 24th
ACM SIGKDD International Conference on Knowl-
edge Discovery & Data Mining, pages 1953–1962.
Mohri, M. and Muñoz Medina, A. (2012). New analysis and
algorithm for learning with drifting distributions. In Int.
Conf. on Alg. Learn. Theo, pages 124–138. Springer.
Montiel, J., Read, J., Bifet, A., and Abdessalem, T. (2018).
Scikit-multiflow: A multi-output streaming framework.
Journal of Machine Learning Research, 19(72):1–5.
Page, E. S. (1954). Continuous inspection schemes.
Biometrika, 41(1-2):100–115.
Pedregosa, F., Varoquaux, G., Gramfort, A., Michel, V.,
Thirion, B., Grisel, O., Blondel, M., Prettenhofer, P.,
Weiss, R., Dubourg, V., Vanderplas, J., Passos, A.,
Cournapeau, D., Brucher, M., Perrot, M., and Duch-
esnay, E. (2011). Scikit-learn: Machine learning
in Python. Journal of Machine Learning Research,
12:2825–2830.
Raab, C., Heusinger, M., and Schleif, F.-M. (2019). Reac-
tive soft prototype computing for frequent reoccurring
concept drift. In ESANN.
Shah, R. D. and Peters, J. (2020). The hardness of condi-
tional independence testing and the generalised covari-
ance measure. The Annals of Statistics, 48(3):1514–
1538.
Shalev-Shwartz, S. and Ben-David, S. (2014). Understand-
ing machine learning: From theory to algorithms.
Cambridge university press.
Street, W. N. and Kim, Y. (2001). A streaming ensemble
algorithm (SEA) for large-scale classification. In Pro-
ceedings of the seventh ACM SIGKDD international
conference on Knowledge discovery and data mining,
San Francisco, CA, USA, August 26-29, 2001, pages
377–382.
Vaquet, V., Menz, P., Seiffert, U., and Hammer, B. (2022).
Investigating intensity and transversal drift in hyper-
spectral imaging data. Neurocomputing, 505:68–79.
Webb, G. I., Lee, L. K., Petitjean, F., and Goethals, B. (2017).
Understanding concept drift. CoRR, abs/1704.00362.
On the Hardness and Necessity of Supervised Concept Drift Detection
175