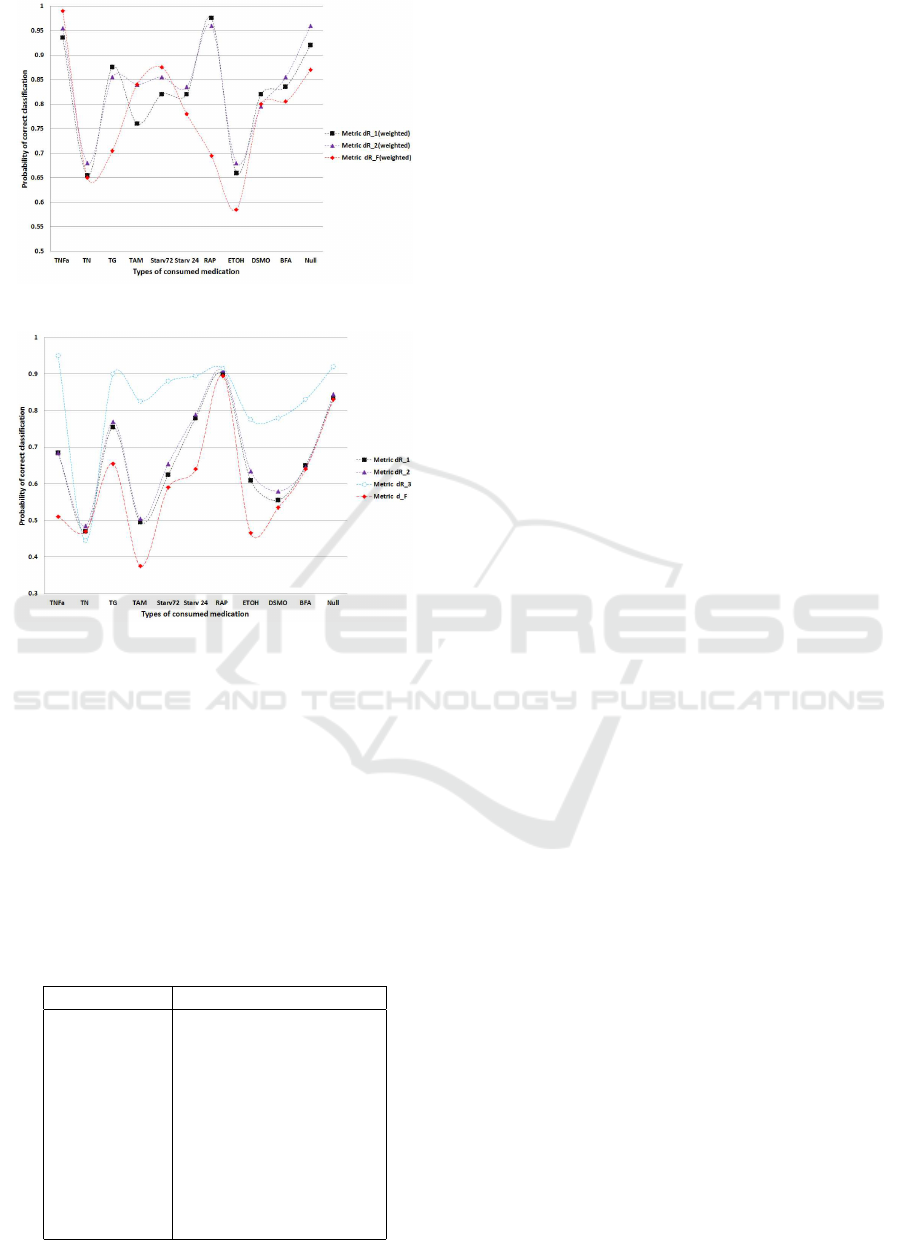
Figure 3: Probability of correct classification - weighted.
Figure 4: Probability of correct classification - non-
weighted.
tance measure between sample covariance matrices
calculated from the HCI real data set. In order to
improve the performance of previously used distance
measures in this paper we use weighted distance mea-
sure as it allows to reduce the probability of clas-
sification errors by increasing the cluster center dis-
tances. We evaluate the performance using dataset
consisting of 11 different classes corresponding to
different treatments. To further improve the classifi-
cation results we perform maximum likelihood based
classification fusion. Our results indicate that the re-
Table 2: Medications and doses.
Treatment ML-fused improvement
DMSO 1%
Ethanol 3%
BFA 1%
Rapamycin 2%
Tamoxifen 2%
Thapsigargin 1%
Tunicamycin 2%
TNFalpha 2%
Starvation24 3%
Starvation72 5%
sults obtained perform similarly to classically used al-
gorithms based on average based classification algo-
rithms. In future work we plan to develop more ef-
ficient computational algorithms and evaluate perfor-
mance as a function of training set size. In addition,
the performance of the proposed algorithm may de-
pend significantly on the algorithm used to construct
a sample covariance set and therefore an effort should
be made to investigate robustness/dependency of the
proposed algorithm on the sampling process.
REFERENCES
Absil, P.-A., Mahony, R., and Sepulchre, R. (2009). Opti-
mization algorithms on matrix manifolds. Princeton
University Press.
Barbaresco, F. (2008). Innovative tools for radar signal pro-
cessing based on Cartans geometry of SPD matrices
& information geometry. Radar Conference, 2008.
RADAR’08. IEEE, pages 1–6.
Debus, B., Parastar, H., Harrington, A., and Kirsanov, D.
(2021). Deep learning in analytical chemistry. Trends
in Analytical Chemistry, 145:116459.
Jahromi, M., Wong, K., and Jeremic, A. (2015). Estimat-
ing Positive Definite Matrices using Frechet Mean. In
Biosignal 2015, pages 2021–2026. INSTIC.
Li, Y., Wong, K., and deBruin, H. (2009). Eeg signal
classification based on a riemannian distance measure.
IEEE TIC-STH, pages 225–230.
Li, Y. and Wong, K. M. (2013). Riemannian distances for
EEG signal classification by power spectral density.
IEEE journal of selected selected topics in signal pro-
cessing.
Liu, B., Jeremic, A., and Wong, K. (2007). Blind adaptive
algorithm for M-ary distributed detection. In IEEE In-
ternational Conference on Acoustics, Speech and Sig-
nal Processing, 2007. ICASSP 2007, volume 2.
Liu, B., Jeremic, A., and Wong, K. (2014). Optimal dis-
tributed detection of multiple hypotheses using blind
algorithm. IEEE Trand. on Aerospace and Electronic
Systems, 50:1190–1203.
Moakher, M. (2005). A differential geometric approach
to the geometric mean of symmetric positive-definite
matrices. SIAM Journal on Matrix Analysis and Ap-
plications, 26(3):735–747.
Shawe-Taylor, J. and Cristianini, N. (2004). Kernel methods
for pattern analysis. Cambridge university press.
Varshney, P. (1986). Optimal data fusion in multiple sen-
sor detection systems. IEEE Trans. on Aerospace and
Electronic Systems, pages 98–101.
Classification of HCI Datasets Using Information Fusion and Weighted Frechet Distance
343