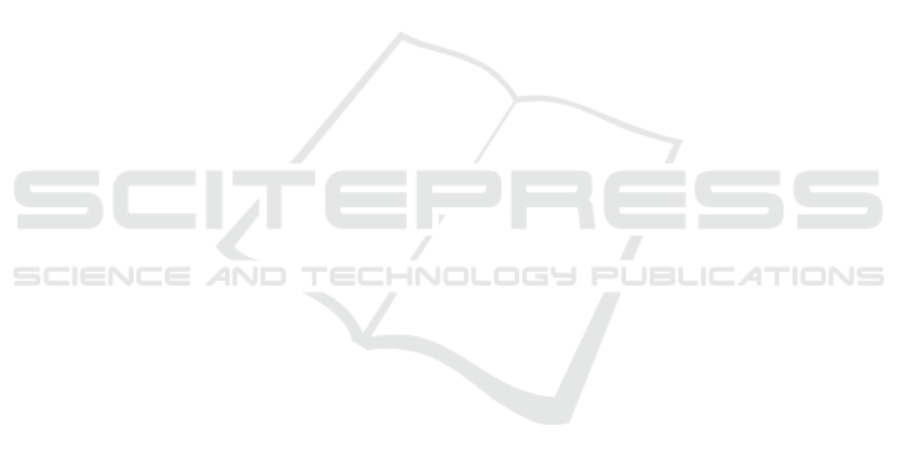
Gao, Q., Chen, Y., Ju, Z., and Liang, Y. (2021). Dynamic
hand gesture recognition based on 3d hand pose esti-
mation for human-robot interaction. IEEE Sensors.
Guzman, A. L. (2018). Human-Machine Communication.
Peter Lang, Bern, Switzerland.
Hans, A. and Hans, E. (2015). Kinesics, haptics and prox-
emics: Aspects of non-verbal communication. IOSR
Journal of Humanities and Social Science (IOSR-
JHSS), 20(2):47–52.
Huang, K.-Y., Wu, C.-H., Hong, Q.-B., Su, M.-H., and
Chen, Y.-H. (2019). Speech emotion recognition us-
ing deep neural network considering verbal and non-
verbal speech sounds. In ICASSP 2019-2019 IEEE In-
ternational Conference on Acoustics, Speech and Sig-
nal Processing (ICASSP), pages 5866–5870. IEEE.
Islam, M. R., Mitu, U. K., Bhuiyan, R. A., and Shin, J.
(2018). Hand gesture feature extraction using deep
convolutional neural network for recognizing ameri-
can sign language. In 4th Int. Conf. on Frontiers of
Signal Processing (ICFSP), pages 115–119.
Key, M. R. (1975). Paralanguage and kinesics (nonverbal
communication).
Kim, J.-H., Choi, S.-J., and Jeong, J.-W. (2019). Watch
& do: A smart iot interaction system with object de-
tection and gaze estimation. IEEE Transactions on
Consumer Electronics, 65(2):195–204.
Kshirsagar, S., Sachdev, S., Singh, N., Tiwari, A., and Sahu,
S. (2020). Iot enabled gesture-controlled home au-
tomation for disabled and elderly. In 2020 Fourth In-
ternational Conference on Computing Methodologies
and Communication (ICCMC), pages 821–826. IEEE.
Luo, W., Cao, J., Ishikawa, K., and Ju, D. (2021). A
human-computer control system based on intelligent
recognition of eye movements and its application in
wheelchair driving. Multimodal Technologies and In-
teraction, 5(9):50.
Lyons, M. J. and Bartneck, C. (2006). HCI and the face.
In CHI’06 Extended Abstracts on Human Factors in
Computing Systems, pages 1671–1674.
Mueller, M., Leuschner, D., Briem, L., Schmidt, M., Kil-
gour, K., Stueker, S., and Waibel, A. (2015). Us-
ing neural networks for data-driven backchannel pre-
diction: A survey on input features and training
techniques. In International conference on human-
computer interaction, pages 329–340. Springer.
M
¨
uller, P., Huang, M. X., Zhang, X., and Bulling, A.
(2018). Robust eye contact detection in natural
multi-person interactions using gaze and speaking be-
haviour. In Proceedings of the 2018 ACM Symposium
on Eye Tracking Research & Applications, pages 1–
10.
Naqvi, R. A., Arsalan, M., Batchuluun, G., Yoon, H. S., and
Park, K. R. (2018). Deep learning-based gaze detec-
tion system for automobile drivers using a nir camera
sensor. Sensors, 18(2):456.
Oh, Y.-H., See, J., Le Ngo, A. C., Phan, R. C.-W., and
Baskaran, V. M. (2018). A survey of automatic fa-
cial micro-expression analysis: databases, methods,
and challenges. Frontiers in psychology, 9:1128.
Pirjol, F. and Ionesc, D.-E. (2019). Communication,
chronemics, silence.
Rafael, S. (2021). The contribution of early multimodal data
fusion for subjectivity in HCI. In 2021 16th Iberian
Conference on Information Systems and Technologies
(CISTI), pages 1–6. IEEE.
Rankin, K. P., Salazar, A., Gorno-Tempini, M. L., Soll-
berger, M., Wilson, S. M., Pavlic, D., Stanley, C. M.,
Glenn, S., Weiner, M. W., and Miller, B. L. (2009).
Detecting sarcasm from paralinguistic cues: anatomic
and cognitive correlates in neurodegenerative disease.
Neuroimage, 47(4):2005–2015.
Seaborn, K., Miyake, N. P., Pennefather, P., and Otake-
Matsuura, M. (2021). Voice in human–agent inter-
action: A survey. ACM Computing Surveys (CSUR),
54(4):1–43.
Steinberg, S. (2007). An introduction to communication
studies. Juta and Company Ltd.
Stephanidis, C., Salvendy, G., Antona, M., Chen, J. Y.,
Dong, J., Duffy, V. G., Fang, X., Fidopiastis, C.,
Fragomeni, G., Fu, L. P., et al. (2019). Seven HCI
grand challenges. International Journal of Human–
Computer Interaction, 35(14):1229–1269.
Strazdas, D., Hintz, J., Khalifa, A., Abdelrahman, A. A.,
Hempel, T., and Al-Hamadi, A. (2022). Robot sys-
tem assistant (RoSA): Towards intuitive multi-modal
and multi-device human-robot interaction. Sensors,
22(3):923.
Su, Z., Zhang, X., Kimura, N., and Rekimoto, J. (2021).
Gaze+ lip: Rapid, precise and expressive interactions
combining gaze input and silent speech commands for
hands-free smart tv control. In ACM Symposium on
Eye Tracking Research and Applications, pages 1–6.
Weisser, A., Miles, K., Richardson, M. J., and Buchholz,
J. M. (2021). Conversational distance adaptation in
noise and its effect on signal-to-noise ratio in realistic
listening environments. The Journal of the Acoustical
Society of America, 149(4):2896–2907.
Wimmer, M., MacDonald, B. A., Jayamuni, D., and Yadav,
A. (2008). Facial expression recognition for human-
robot interaction–a prototype. In International Work-
shop on Robot Vision, pages 139–152. Springer.
Xia, Z., Hong, X., Gao, X., Feng, X., and Zhao, G. (2019).
Spatiotemporal recurrent convolutional networks for
recognizing spontaneous micro-expressions. IEEE
Transactions on Multimedia, 22(3):626–640.
Xie, B., Sidulova, M., and Park, C. H. (2021). Robust
multimodal emotion recognition from conversation
with transformer-based crossmodality fusion. Sen-
sors, 21(14):4913.
Yongda, D., Fang, L., and Huang, X. (2018). Research on
multimodal human-robot interaction based on speech
and gesture. Computers & Electrical Engineering,
72:443–454.
BIOSIGNALS 2023 - 16th International Conference on Bio-inspired Systems and Signal Processing
350