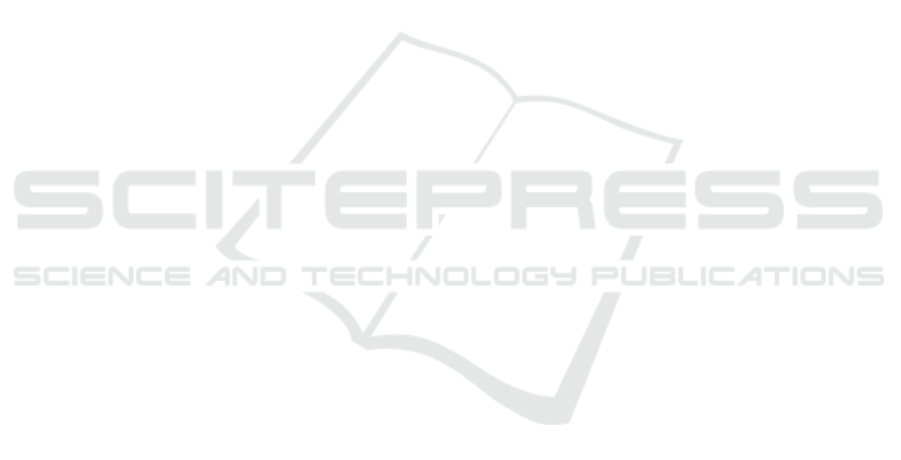
REFERENCES
Almond, R., Grooten, M., Juffe Bignoli, D., and Petersen,
T. (2022). Wwf (2022) living planet report 2022 -
building a nature-positive society. 1
Alshammari, S., Wang, Y.-X., Ramanan, D., and Kong, S.
(2022). Long-tailed recognition via weight balancing.
In CVPR, pages 6897–6907. 2, 3, 5
Andrew, W., Gao, J., Mullan, S., Campbell, N., Dowsey,
A. W., and Burghardt, T. (2021). Visual identification
of individual holstein-friesian cattle via deep metric
learning. Computers and Electronics in Agriculture,
185:106133. 4
Bain, M., Nagrani, A., Schofield, D., Berdugo, S., Bessa, J.,
Owen, J., Hockings, K. J., Matsuzawa, T., Hayashi,
M., Biro, D., et al. (2021). Automated audiovisual
behavior recognition in wild primates. Science ad-
vances, 7(46):eabi4883. 1, 3
Carvalho, S., Wessling, E. G., Abwe, E. E., Almeida-
Warren, K., Arandjelovic, M., Boesch, C., Danquah,
E., Diallo, M. S., Hobaiter, C., Hockings, K., et al.
(2022). Using nonhuman culture in conservation re-
quires careful and concerted action. Conservation Let-
ters, 15(2):e12860. 1
Congdon, J., Hosseini, M., Gading, E., Masousi, M.,
Franke, M., and MacDonald, S. (2022). The future
of artificial intelligence in monitoring animal identifi-
cation, health, and behaviour. 1
Cui, Y., Jia, M., Lin, T.-Y., Song, Y., and Belongie, S.
(2019). Class-balanced loss based on effective num-
ber of samples. In CVPR, pages 9268–9277. 2, 3,
5
Dominoni, D. M., Halfwerk, W., Baird, E., Buxton, R. T.,
Fern
´
andez-Juricic, E., Fristrup, K. M., McKenna,
M. F., Mennitt, D. J., Perkin, E. K., Seymoure, B. M.,
et al. (2020). Why conservation biology can benefit
from sensory ecology. Nature Ecology & Evolution,
4(4):502–511. 1
Du Tran, H. W., Torresani, L., Ray, J., Lecun, Y., and Paluri,
M. (2017). A closer look at spatiotemporal convolu-
tions for action recognition.(2017). OK. 4
Duan, M., Qiu, H., Zhang, Z., and Wu, Y. (2021). Ntu-
densepose: A new benchmark for dense pose action
recognition. In Big Data, pages 3170–3175. IEEE. 3
Feichtenhofer, C., Fan, H., Malik, J., and He, K. (2019).
Slowfast networks for video recognition. In ICCV,
pages 6202–6211. 2
Hayakawa, J. and Dariush, B. (2020). Recognition and
3d localization of pedestrian actions from monocular
video. In ITSC, pages 1–7. IEEE. 3
Hermans, A., Beyer, L., and Leibe, B. (2017). In defense
of the triplet loss for person re-identification. arXiv
preprint arXiv:1703.07737. 1, 4
Hong, J., Cho, B., Hong, Y. W., and Byun, H. (2019).
Contextual action cues from camera sensor for multi-
stream action recognition. Sensors, 19(6):1382. 3
IUCN (2022). Iucn red list of threatened species version
2022.1. 1
Kalfaoglu, M. E., Kalkan, S., and Alatan, A. A. (2020).
Late temporal modeling in 3d cnn architectures with
bert for action recognition. In ECCV, pages 731–747.
Springer. 2
Kang, B., Xie, S., Rohrbach, M., Yan, Z., Gordo, A., Feng,
J., and Kalantidis, Y. (2019). Decoupling representa-
tion and classifier for long-tailed recognition. arXiv
preprint arXiv:1910.09217. 3
Karaderi, T., Burghardt, T., Hsiang, A. Y., Ramaer, J., and
Schmidt, D. N. (2022). Visual microfossil identifica-
tion via deep metric learning. In ICPRAI, pages 34–
46. Springer. 1
Kay, W., Carreira, J., Simonyan, K., Zhang, B., Hillier, C.,
Vijayanarasimhan, S., Viola, F., Green, T., Back, T.,
Natsev, P., et al. (2017). The kinetics human action
video dataset. arXiv preprint arXiv:1705.06950. 5
K
¨
uhl, H. S. and Burghardt, T. (2013). Animal biomet-
rics: quantifying and detecting phenotypic appear-
ance. TREE, 28(7):432–441. 1
Le, V.-T., Tran-Trung, K., and Hoang, V. T. (2022). A com-
prehensive review of recent deep learning techniques
for human activity recognition. Computational Intel-
ligence and Neuroscience, 2022. 2
Li, Y., Lu, Z., Xiong, X., and Huang, J. (2022). Perf-net:
Pose empowered rgb-flow net. In WACV, pages 513–
522. 3
Lin, J., Gan, C., and Han, S. (2019). Tsm: Temporal shift
module for efficient video understanding. In ICCV,
pages 7083–7093. 2
Liu, Z., Miao, Z., Zhan, X., Wang, J., Gong, B., and Yu,
S. X. (2019). Large-scale long-tailed recognition in
an open world. In CVPR, pages 2537–2546. 3
Majd, M. and Safabakhsh, R. (2020). Correlational con-
volutional lstm for human action recognition. Neuro-
computing, 396:224–229. 2
Menon, A. K., Jayasumana, S., Rawat, A. S., Jain, H., Veit,
A., and Kumar, S. (2020). Long-tail learning via logit
adjustment. arXiv preprint arXiv:2007.07314. 2, 3, 5
Musgrave, K., Belongie, S., and Lim, S.-N. (2020). Pytorch
metric learning. 1
Nishida, T., Kano, T., Goodall, J., McGrew, W. C., and
Nakamura, M. (1999). Ethogram and ethnography
of mahale chimpanzees. Anthropological Science,
107(2):141–188. 1
Pan, Y., Xu, J., Wang, M., Ye, J., Wang, F., Bai, K., and
Xu, Z. (2019). Compressing recurrent neural networks
with tensor ring for action recognition. In AAAI, vol-
ume 33, pages 4683–4690. 2
Sakib, F. and Burghardt, T. (2020). Visual recognition of
great ape behaviours in the wild. VAIB. 1, 2, 3, 4, 5
Sanakoyeu, A., Khalidov, V., McCarthy, M. S., Vedaldi, A.,
and Neverova, N. (2020). Transferring dense pose to
proximal animal classes. In CVPR, pages 5233–5242.
2, 4
Shaikh, M. B. and Chai, D. (2021). Rgb-d data-based action
recognition: A review. Sensors, 21(12):4246. 2
Sharir, G., Noy, A., and Zelnik-Manor, L. (2021). An image
is worth 16x16 words, what is a video worth? arXiv
preprint arXiv:2103.13915. 2
Simonyan, K. and Zisserman, A. (2014). Two-stream con-
volutional networks for action recognition in videos.
NeurIPS, 27. 2, 3
Triple-stream Deep Metric Learning of Great Ape Behavioural Actions
301