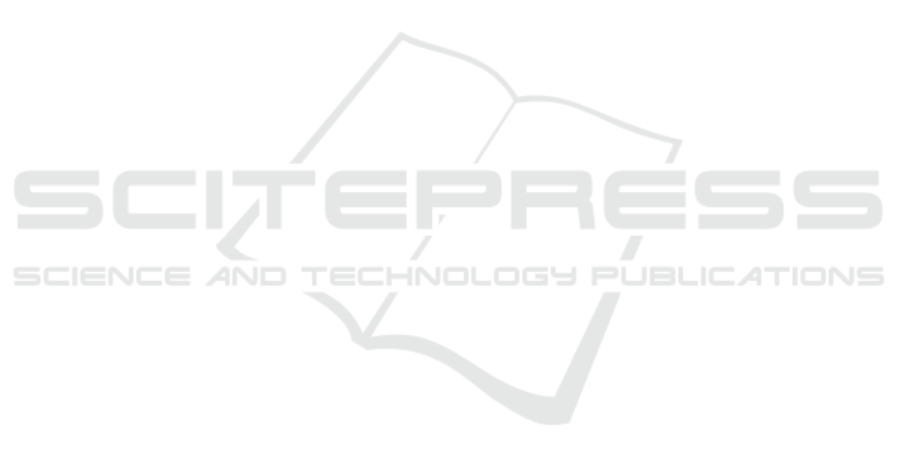
5 CONCLUSIONS
In this work, we performed experiments to analyze
the agreement between measurements obtained with
the same and different models of IMUs. We in-
cluded dedicated inertial sensors as well as a common
model of a smartwatch. Results indicate, that while
the agreement is relatively good, it is not sufficient
to simply substitute a device with another model or
use a heterogeneous setup without additional consid-
eration. However, in some applications, with proper
adaptation, using multiple different sensors could be
a viable solution. More importantly, from a practi-
cal point of view, employing an everyday-use device
such as a smartwatch is just as good (or even better)
as using another model of IMU. Discrepancies be-
tween measurements obtained with different devices
are more significant in dynamic motion. Therefore, in
scenarios such as analyzing fast, sports actions it may
be necessary to calibrate motion analysis methods per
device.
In terms of future work, it would be beneficial to
verify the repeatability of measurements per device,
as well as perform experiments with additional de-
vices. We also consider comparing signals from dif-
ferent sensors as inputs to machine learning meth-
ods for action detection or classification. Such ex-
periments would include multiple subjects. Training
machine learning models on one set of sensors and
testing on another would be a good indication of the
viability of heterogeneous setups in practical scenar-
ios.
ACKNOWLEDGEMENTS
The research presented in this paper was supported
by the National Centre for Research and Develop-
ment (NCBiR) under Grant No. LIDER/37/0198/L-
12/20/NCBR/2021.
REFERENCES
Bavan, L., Surmacz, K., Beard, D., Mellon, S., and Rees, J.
(2019). Adherence monitoring of rehabilitation exer-
cise with inertial sensors: A clinical validation study.
Gait & posture, 70:211–217.
Bevilacqua, A., Huang, B., Argent, R., Caulfield, B., and
Kechadi, T. (2018). Automatic classification of knee
rehabilitation exercises using a single inertial sensor:
A case study. In 2018 IEEE 15th International Con-
ference on Wearable and Implantable Body Sensor
Networks (BSN), pages 21–24. IEEE.
Camomilla, V., Bergamini, E., Fantozzi, S., and Vannozzi,
G. (2018). Trends supporting the in-field use of wear-
able inertial sensors for sport performance evaluation:
A systematic review. Sensors, 18(3):873.
Clemente, F., Badicu, G., Hassan, U., Akyildiz, Z., Pino-
Ortega, J., Silva, R., and Rico-Gonz
´
alez, M. (2022).
Validity and reliability of inertial measurement units
for jump height estimations: a systematic review. Hu-
man Movement, 23(4):1–20.
Giggins, O. M., Sweeney, K. T., and Caulfield, B. (2014).
Rehabilitation exercise assessment using inertial sen-
sors: a cross-sectional analytical study. Journal of
neuroengineering and rehabilitation, 11(1):1–10.
Khediri, S. e., Nasri, N., Samet, M., Wei, A., and Kachouri,
A. (2012). Analysis study of time synchronization
protocols in wireless sensor networks. arXiv preprint
arXiv:1206.1419.
Kobsar, D., Masood, Z., Khan, H., Khalil, N., Kiwan, M. Y.,
Ridd, S., and Tobis, M. (2020). Wearable inertial sen-
sors for gait analysis in adults with osteoarthritis—a
scoping review. Sensors, 20(24):7143.
Lopez-Nava, I. H. and Munoz-Melendez, A. (2016). Wear-
able inertial sensors for human motion analysis: A re-
view. IEEE Sensors Journal, 16(22):7821–7834.
Malawski, F. (2020). Depth versus inertial sensors in real-
time sports analysis: a case study on fencing. IEEE
Sensors Journal, 21(4):5133–5142.
Milosevic, B., Leardini, A., and Farella, E. (2020). Kinect
and wearable inertial sensors for motor rehabilitation
programs at home: state of the art and an experimental
comparison. Biomedical engineering online, 19(1):1–
26.
O’Reilly, M., Caulfield, B., Ward, T., Johnston, W., and Do-
herty, C. (2018). Wearable inertial sensor systems for
lower limb exercise detection and evaluation: a sys-
tematic review. Sports Medicine, 48(5):1221–1246.
Petraglia, F., Scarcella, L., Pedrazzi, G., Brancato, L.,
Puers, R., and Costantino, C. (2019). Inertial sensors
versus standard systems in gait analysis: a systematic
review and meta-analysis. European journal of physi-
cal and rehabilitation medicine, 55(2):265–280.
Ren, H. and Wang, X. (2021). Application of wearable
inertial sensor in optimization of basketball player’s
human motion tracking method. Journal of Ambient
Intelligence and Humanized Computing, pages 1–15.
Roell, M., Mahler, H., Lienhard, J., Gehring, D., Gollhofer,
A., and Roecker, K. (2019). Validation of wearable
sensors during team sport-specific movements in in-
door environments. Sensors, 19(16):3458.
Teufl, W., Lorenz, M., Miezal, M., Taetz, B., Fr
¨
ohlich,
M., and Bleser, G. (2018). Towards inertial sen-
sor based mobile gait analysis: Event-detection and
spatio-temporal parameters. Sensors, 19(1):38.
Tunca, C., Pehlivan, N., Ak, N., Arnrich, B., Salur, G.,
and Ersoy, C. (2017). Inertial sensor-based robust gait
analysis in non-hospital settings for neurological dis-
orders. Sensors, 17(4):825.
Wang, Y., Zhao, Y., Chan, R. H., and Li, W. J. (2018). Vol-
leyball skill assessment using a single wearable mi-
Heterogeneous Inertial Measurement Units in Motion Analysis
357