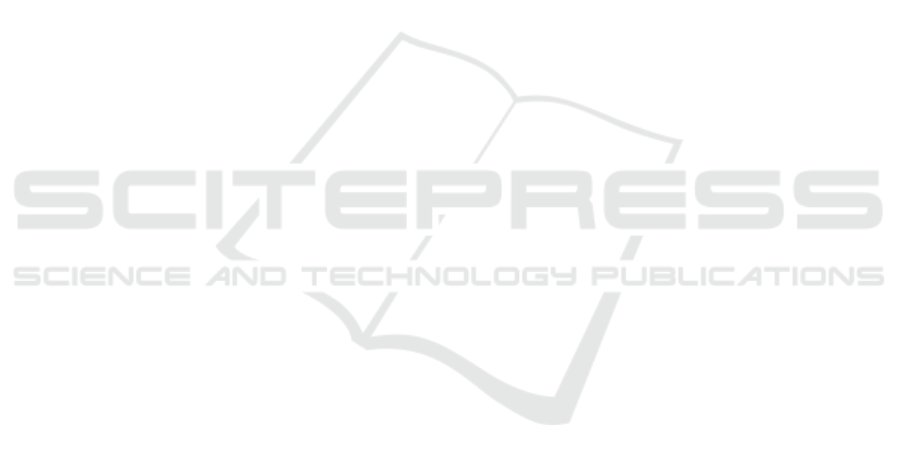
7 CONCLUSION AND FUTURE
SCOPE
In this work, we improved the capabilities of single
image models to accommodate multiple images. This
is possible with simple assumptions of similarities in
underlying content and a modified discriminator ar-
chitecture and objective function. When we consider
two face images that are roughly aligned, but differ
in other aspects like texture, color and light intensi-
ties, our method involves learning a distribution of the
patches that appear from the natural composition of
the input images. The idea extends to multiple im-
ages, assuming that the images are roughly aligned
and the images share similar underlying content lay-
outs. Our method generates diverse set of hundreds
of data samples by training on just two input images.
Future work can focus on improving control over the
style at global and local level.
REFERENCES
Arantes, R. B., Vogiatzis, G., and Faria, D. R. (2020). rcgan:
Learning a generative model for arbitrary size image
generation. In Bebis, G., Yin, Z., Kim, E., Bender,
J., Subr, K., Kwon, B. C., Zhao, J., Kalkofen, D., and
Baciu, G., editors, Advances in Visual Computing.
Chen, X., Zhao, H., Yang, D., Li, Y., Kang, Q., and Lu,
H. (2021). Sa-singan: self-attention for single-image
generation adversarial networks. Machine Vision and
Applications, 32(4):104.
Clou
ˆ
atre, L. and Demers, M. (2019). FIGR: few-shot image
generation with reptile. CoRR, abs/1901.02199.
Ding, G., Han, X., Wang, S., Wu, S., Jin, X., Tu, D., and
Huang, Q. (2022). Attribute group editing for reliable
few-shot image generation. 2022 IEEE CVPR.
Donahue, J. and Simonyan, K. (2019). Large scale adver-
sarial representation learning. CoRR, abs/1907.02544.
Dosovitskiy, A. and Brox, T. (2016). Generating images
with perceptual similarity metrics based on deep net-
works. CoRR, abs/1602.02644.
Gu, Z., Li, W., Huo, J., Wang, L., and Gao, Y. Lofgan:
Fusing local representations for few-shot image gen-
eration. In Proceedings of the IEEE/CVF ICCV.
Gulrajani, I., Ahmed, F., Arjovsky, M., Dumoulin, V., and
Courville, A. C. (2017). Improved training of wasser-
stein gans. CoRR, abs/1704.00028.
Hinz, T., Fisher, M., Wang, O., and Wermter, S. (2020).
Improved techniques for training single-image gans.
CoRR, abs/2003.11512.
Hong, Y., Niu, L., Zhang, J., and Zhang, L. (2020a). Match-
inggan: Matching-based few-shot image generation.
CoRR, abs/2003.03497.
Hong, Y., Niu, L., Zhang, J., and Zhang, L. (2022). Delta-
gan: Towards diverse few-shot image generation with
sample-specific delta. In ECCV.
Hong, Y., Niu, L., Zhang, J., Zhao, W., Fu, C., and Zhang,
L. (2020b). F2GAN: fusing-and-filling GAN for few-
shot image generation. CoRR, abs/2008.01999.
Karras, T., Laine, S., Aittala, M., Hellsten, J., Lehtinen, J.,
and Aila, T. (2020). Analyzing and improving the im-
age quality of StyleGAN. In Proc. CVPR.
Kong, C., Kim, J., Han, D., and Kwak, N. (2021). Smooth-
ing the generative latent space with mixup-based dis-
tance learning. CoRR, abs/2111.11672.
Kumar, R., Dabral, R., and Sivakumar, G. (2021).
Learning unsupervised cross-domain image-to-image
translation using a shared discriminator. CoRR,
abs/2102.04699.
Liang, W., Liu, Z., and Liu, C. (2020). DAWSON: A do-
main adaptive few shot generation framework. CoRR,
abs/2001.00576.
Liu, B., Zhu, Y., Song, K., and Elgammal, A. (2021).
Towards faster and stabilized GAN training for
high-fidelity few-shot image synthesis. CoRR,
abs/2101.04775.
Liu, M.-Y., Huang, X., Mallya, A., Karras, T., Aila, T.,
Lehtinen, J., and Kautz., J. (2019). Few-shot un-
sueprvised image-to-image translation. In arxiv.
Liu, Z., Luo, P., Wang, X., and Tang, X. (2015). Deep learn-
ing face attributes in the wild. In In ICCV.
Ojha, U., Li, Y., Lu, J., Efros, A. A., Lee, Y. J., Shecht-
man, E., and Zhang, R. (2021). Few-shot image
generation via cross-domain correspondence. CoRR,
abs/2104.06820.
Ruiz, N., Theobald, B., Ranjan, A., Abdelaziz, A. H., and
Apostoloff, N. (2020). Morphgan: One-shot face syn-
thesis GAN for detecting recognition bias. CoRR,
abs/2012.05225.
Shaham, T. R., Dekel, T., and Michaeli, T. (2019). Singan:
Learning a generative model from a single natural im-
age. CoRR, abs/1905.01164.
Shocher, A., Bagon, S., Isola, P., and Irani, M. (2019). In-
gan: Capturing and retargeting the ”dna” of a natural
image. In The IEEE ICCV.
Shocher, A., Cohen, N., and Irani, M. (2017). ”zero-shot”
super-resolution using deep internal learning.
Sushko, V., Gall, J., and Khoreva, A. (2021). One-shot
GAN: learning to generate samples from single im-
ages and videos. CoRR, abs/2103.13389.
Tritrong, N., Rewatbowornwong, P., and Suwajanakorn, S.
(2021). Repurposing gans for one-shot semantic part
segmentation. In IEEE CVPR.
Tundia, C., Kumar, R., Damani, O. P., and Sivakumar,
G. (2021). The MIS check-dam dataset for object
detection and instance segmentation tasks. CoRR,
abs/2111.15613.
Yang, W., Zhang, X., Tian, Y., Wang, W., Xue, J.-H., and
Liao, Q. (2019). Deep learning for single image super-
resolution: A brief review. IEEE Transactions on Mul-
timedia, 21(12):3106–3121.
Zhao, S., Liu, Z., Lin, J., Zhu, J., and Han, S. (2020). Dif-
ferentiable augmentation for data-efficient GAN train-
ing. CoRR, abs/2006.10738.
DEff-GAN: Diverse Attribute Transfer for Few-Shot Image Synthesis
877