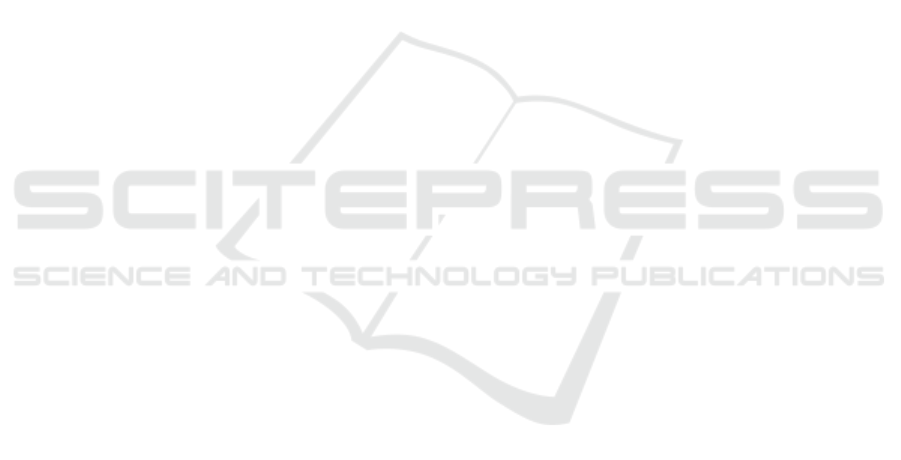
6 CONCLUSION
In this paper, we propose Farsighter, an multi-step un-
certainty exploration framework in DRL and we can
explicitly adjust the number of future steps to bal-
ance the Q-estimation bias-variance trade-off. Far-
sighter helps to alleviate the sparse reward and un-
certainty vanishing problem. Moreover, it avoids the
uncertainty to be too large in the uncertainty propaga-
tion methods. It outperforms SOTA on a wide range
of RL tasks with high/low-dimensional states, dis-
crete/continuous actions, and sparse/dense rewards,
including high-dimensional Atari games and contin-
uous control robotic manipulation tasks.
ACKNOWLEDGEMENTS
The work was partially supported through grant
USDA/NIFA 2020-67021-32855, and by NSF
through IIS-1838207, CNS 1901218, OIA-2134901.
REFERENCES
Antos, A., Szepesv
´
ari, C., and Munos, R. (2008). Learn-
ing near-optimal policies with bellman-residual mini-
mization based fitted policy iteration and a single sam-
ple path. Machine Learning, 71(1):89–129.
Azizzadenesheli, K., Brunskill, E., and Anandkumar, A.
(2018). Efficient exploration through bayesian deep
q-networks. In 2018 Information Theory and Appli-
cations Workshop (ITA), pages 1–9. IEEE.
Bai, C., Wang, L., Han, L., Hao, J., Garg, A., Liu, P., and
Wang, Z. (2021). Principled exploration via optimistic
bootstrapping and backward induction. In Interna-
tional Conference on Machine Learning, pages 577–
587. PMLR.
Bellemare, M. G., Naddaf, Y., Veness, J., and Bowling, M.
(2013). The arcade learning environment: An evalua-
tion platform for general agents. Journal of Artificial
Intelligence Research, 47:253–279.
Blei, D. M., Kucukelbir, A., and McAuliffe, J. D.
(2017). Variational inference: A review for statisti-
cians. Journal of the American statistical Association,
112(518):859–877.
Ecoffet, A., Huizinga, J., Lehman, J., Stanley, K. O.,
and Clune, J. (2019). Go-explore: a new ap-
proach for hard-exploration problems. arXiv preprint
arXiv:1901.10995.
Gu, S., Lillicrap, T., Sutskever, I., and Levine, S. (2016).
Continuous deep q-learning with model-based accel-
eration. In International conference on machine learn-
ing, pages 2829–2838. PMLR.
Janz, D., Hron, J., Mazur, P., Hofmann, K., Hern
´
andez-
Lobato, J. M., and Tschiatschek, S. (2019). Successor
uncertainties: exploration and uncertainty in temporal
difference learning. Advances in Neural Information
Processing Systems, 32.
Kononenko, I. (1989). Bayesian neural networks. Biologi-
cal Cybernetics, 61(5):361–370.
Liu, Y., Chen, J., and Chen, H. (2018). Less is more:
Culling the training set to improve robustness of deep
neural networks. In International Conference on De-
cision and Game Theory for Security, pages 102–114.
Springer.
Liu, Y., Ding, J., and Liu, X. (2020a). A constrained rein-
forcement learning based approach for network slic-
ing. In 2020 IEEE 28th International Conference on
Network Protocols (ICNP), pages 1–6. IEEE.
Liu, Y., Ding, J., and Liu, X. (2020b). Ipo: Interior-point
policy optimization under constraints. In Proceedings
of the AAAI Conference on Artificial Intelligence, vol-
ume 34, pages 4940–4947.
Liu, Y., Ding, J., and Liu, X. (2021a). Resource alloca-
tion method for network slicing using constrained re-
inforcement learning. In 2021 IFIP Networking Con-
ference (IFIP Networking), pages 1–3. IEEE.
Liu, Y., Ding, J., Zhang, Z.-L., and Liu, X. (2021b).
Clara: A constrained reinforcement learning based re-
source allocation framework for network slicing. In
2021 IEEE International Conference on Big Data (Big
Data), pages 1427–1437. IEEE.
Liu, Y., Halev, A., and Liu, X. (2021c). Policy learning
with constraints in model-free reinforcement learning:
A survey. In The 30th International Joint Conference
on Artificial Intelligence (IJCAI).
Liu, Y. and Liu, X. (2021). Cts2: Time series smooth-
ing with constrained reinforcement learning. In Asian
Conference on Machine Learning, pages 363–378.
PMLR.
Liu, Y. and Liu, X. (2023a). Adventurer: Exploration with
bigan for deep reinforcement learning. Applied Intel-
ligence.
Liu, Y. and Liu, X. (2023b). Constrained reinforcement
learning for autonomous farming: Challenges and op-
portunities. In AI for Agriculture and Food Systems.
Lu, L., Liu, L., Hussain, M. J., and Liu, Y. (2017). I sense
you by breath: Speaker recognition via breath biomet-
rics. IEEE Transactions on Dependable and Secure
Computing, 17(2):306–319.
Lu, L. and Liu, Y. (2015). Safeguard: User reauthen-
tication on smartphones via behavioral biometrics.
IEEE Transactions on Computational Social Systems,
2(3):53–64.
Metelli, A. M., Likmeta, A., and Restelli, M. (2019).
Propagating uncertainty in reinforcement learning via
wasserstein barycenters. Advances in Neural Informa-
tion Processing Systems, 32.
Mnih, V., Badia, A. P., Mirza, M., Graves, A., Lillicrap, T.,
Harley, T., Silver, D., and Kavukcuoglu, K. (2016).
Asynchronous methods for deep reinforcement learn-
ing. In International conference on machine learning,
pages 1928–1937. PMLR.
Mnih, V., Kavukcuoglu, K., Silver, D., Rusu, A. A., Veness,
J., Bellemare, M. G., Graves, A., Riedmiller, M., Fid-
jeland, A. K., Ostrovski, G., et al. (2015). Human-
ICAART 2023 - 15th International Conference on Agents and Artificial Intelligence
388