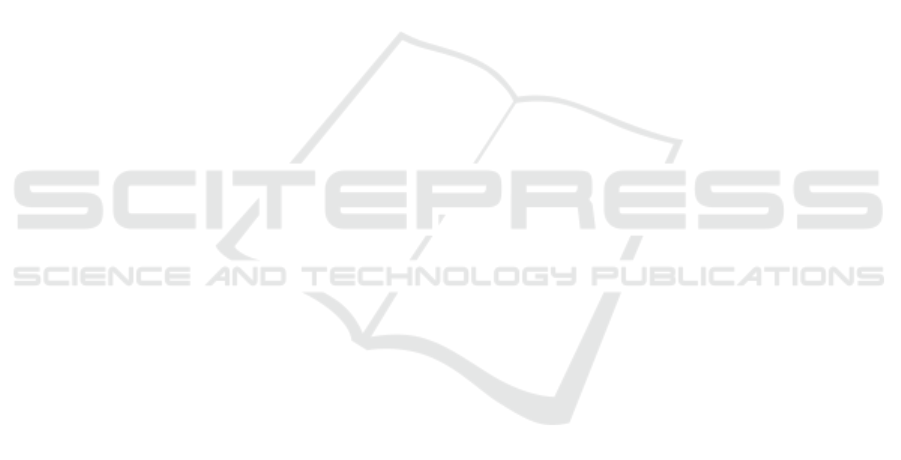
a novel analysis that includes experimentation with
simulation and track driving. The second and third
outcomes can be concurrently utilised in enhancing
the simulator techniques to train road users for a safer
traffic environment through the functional develop-
ment of the proposed drivers’ behaviour monitoring
system.
The outcome of this study is encouraging in terms
of explanation methods that require further research.
The lack of prescribed evaluation metrics in the lit-
erature led to the use of different borrowed metrics
from different concepts. However, the results showed
promising possibilities to enhance and modify them
for future works on the evaluation of explanation
methods. Another possible research direction would
be to improve the feature attribution methods to pro-
duce more insightful explanations.
ACKNOWLEDGEMENTS
This study was performed as a part of the project
SIMUSAFE funded by the European Union’s Hori-
zon 2020 research and innovation programme under
grant agreement N. 723386.
REFERENCES
Abadi, M. L. and Boubezoul, A. (2021). Deep neural net-
works for classification of riding patterns: with a focus
on explainability. In Proceedings of the 29th Euro-
pean Symposium on Artificial Neural Networks, Com-
putational Intelligence and Machine Learning.
Antwarg, L., Miller, R. M., Shapira, B., and Rokach, L.
(2021). Explaining anomalies detected by autoen-
coders using shapley additive explanations. Expert
Systems with Applications, 186:115736.
Barua, S., Ahmed, M. U., Ahlstrom, C., Begum, S., and
Funk, P. (2017). Automated EEG Artifact Handling
with Application in Driver Monitoring. IEEE Journal
of Biomedical and Health Informatics, 22(5):1350.
Busa-Fekete, R., Szarvas, G., Elteto, T., and K
´
egl, B.
(2012). An Apple-to-Apple Comparison of Learning-
to-Rank Algorithms in terms of Normalized Dis-
counted Cumulative Gain. In ECAI 2012-20th Euro-
pean Conference on Artificial Intelligence: Preference
Learning: Problems and Applications in AI Work-
shop, volume 242. Ios Press.
Chawla, N. V., Bowyer, K. W., Hall, L. O., and Kegelmeyer,
W. P. (2002). SMOTE: Synthetic Minority Over-
Sampling Technique. Journal of Artificial Intelligence
Research, 16:321–357.
Corcoran, A. W., Alday, P. M., Schlesewsky, M., and
Bornkessel-Schlesewsky, I. (2018). Toward a Re-
liable, Automated Method of Individual Alpha Fre-
quency Quantification. Psychophysiology, 55(7).
Islam, M. R., Ahmed, M. U., Barua, S., and Begum, S.
(2022). A Systematic Review of Explainable Artifi-
cial Intelligence in terms of Different Application Do-
mains and Tasks. Applied Sciences, 12(3):1353.
Islam, M. R., Barua, S., Ahmed, M. U., Begum, S., Aric
`
o,
P., Borghini, G., and Di Flumeri, G. (2020). A Novel
Mutual Information based Feature Set for Drivers’
Mental Workload Evaluation using Machine Learn-
ing. Brain Sciences, 10(8):551.
Letzgus, S., Wagner, P., Lederer, J., Samek, W., M
¨
uller,
K.-R., and Montavon, G. (2022). Toward Explain-
able Artificial Intelligence for Regression Models: A
Methodological Perspective. IEEE Signal Processing
Magazine, 39(4):40–58.
Liu, Y., Khandagale, S., White, C., and Neiswanger, W.
(2021). Synthetic benchmarks for scientific research
in explainable machine learning. arXiv preprint
arXiv:2106.12543.
Lundberg, S. M. and Lee, S.-I. (2017). A Unified Approach
to Interpreting Model Predictions. Advances in neural
information processing systems, 30.
Oostenveld, R., Fries, P., Maris, E., and Schoffelen, J.-M.
(2011). FieldTrip: Open Source Software for Ad-
vanced Analysis of MEG, EEG, and Invasive Electro-
physiological Data. Computational Intelligence and
Neuroscience, 2011.
Pedregosa, F., Varoquaux, G., Gramfort, A., Michel, V.,
Thirion, B., Grisel, O., Blondel, M., Prettenhofer, P.,
Weiss, R., and Dubourg, V. (2011). Scikit-learn: Ma-
chine Learning in Python. The Journal of Machine
Learning Research, 12:2825–2830.
Ribeiro, M. T., Singh, S., and Guestrin, C. (2016). ”Why
should I trust you?” Explaining the Predictions of any
Classifier. In Proceedings of the 22nd ACM SIGKDD
international conference on knowledge discovery and
data mining, pages 1135–1144.
Sætren, G. B., Lindheim, C., Skogstad, M. R., Andreas Ped-
ersen, P., Robertsen, R., Lødemel, S., and Haukeberg,
P. J. (2019). Simulator versus Traditional Training: A
Comparative Study of Night Driving Training. In Pro-
ceedings of the Human Factors and Ergonomics So-
ciety Annual Meeting, volume 63, pages 1669–1673.
SAGE Publications Sage CA: Los Angeles, CA.
Serradilla, O., Zugasti, E., Ramirez de Okariz, J., Ro-
driguez, J., and Zurutuza, U. (2021). Adaptable and
explainable predictive maintenance: semi-supervised
deep learning for anomaly detection and diagnosis in
press machine data. Applied Sciences, 11(16):7376.
Sokolova, M. and Lapalme, G. (2009). A Systematic Analy-
sis of Performance Measures for Classification Tasks.
Information processing & management, 45(4):427.
Voigt, P. and Von dem Bussche, A. (2017). The EU Gen-
eral Data Protection Regulation (GDPR). A Practical
Guide, 1st Ed., Cham: Springer International Pub-
lishing, 10:3152676.
Wilcoxon, F. (1992). Individual Comparisons by Ranking
Methods. In Breakthroughs in statistics, pages 196–
202. Springer.
Wu, S.-L., Tung, H.-Y., and Hsu, Y.-L. (2020). Deep
Learning for Automatic Quality Grading of Mangoes:
Interpretable Machine Learning for Modelling and Explaining Car Drivers’ Behaviour: An Exploratory Analysis on Heterogeneous Data
403