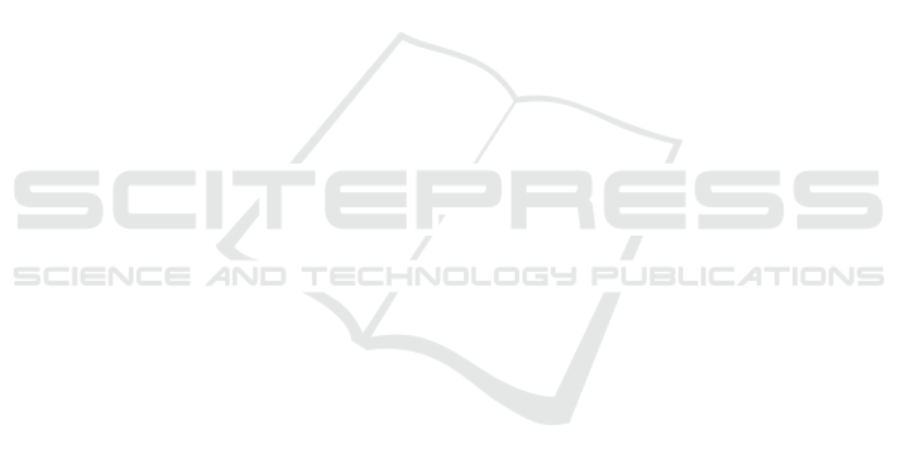
REFERENCES
Abdel-Fattah, M., Kohler, H., Rotenberger, P., and Scholer,
L. (2020). A review of the holonic architecture for
the smart grids and the self-healing application. pages
1–6.
Amin, S. M. and Wollenberg, B. F. (2005). Toward a smart
grid: power delivery for the 21st century. IEEE power
and energy magazine, 3(5):34–41.
Ansari, J., Kazemi, A., and Gholami, A. (2015). Holonic
structure: A state-of-the-art control architecture based
on multi-agent systems for optimal reactive power dis-
patch in smart grids. IET Generation, Transmission &
Distribution, 9.
Brockway, P. E., Owen, A., Brand-Correa, L. I., and Hardt,
L. (2019). Estimation of global final-stage energy-
return-on-investment for fossil fuels with compari-
son to renewable energy sources. Nature Energy,
4(7):612–621.
Dudek, G., Pełka, P., and Smyl, S. (2021). A hybrid resid-
ual dilated lstm and exponential smoothing model for
midterm electric load forecasting. IEEE Transactions
on Neural Networks and Learning Systems.
Espe, E., Potdar, V., and Chang, E. (2018). Prosumer com-
munities and relationships in smart grids: A litera-
ture review, evolution and future directions. Energies,
11(10).
Ferreira, A.,
ˆ
Angela Ferreira, Cardin, O., and Leit
˜
ao, P.
(2015). Extension of holonic paradigm to smart grids.
IFAC-PapersOnLine, 48(3):1099–1104. 15th IFAC
Symposium onInformation Control Problems inMan-
ufacturing.
Gerber, C., Siekmann, J., and Vierke, G. (1999). Holonic
multi-agent systems.
Ghorbani, S. and Unland, R. (2016). A holonic multi-agent
control system for networks of micro-grids. In Klusch,
M., Unland, R., Shehory, O., Pokahr, A., and Ahrndt,
S., editors, Multiagent System Technologies, pages
231–238, Cham. Springer International Publishing.
Green, R. C., Wang, L., and Alam, M. (2011). The im-
pact of plug-in hybrid electric vehicles on distribution
networks: A review and outlook. Renewable and Sus-
tainable Energy Reviews, 15(1):544–553.
Hannan, M., Mollik, M., Al-Shetwi, A. Q., Rahman, S.,
Mansor, M., Begum, R., Muttaqi, K., and Dong, Z.
(2022). Vehicle to grid connected technologies and
charging strategies: Operation, control, issues and
recommendations. Journal of Cleaner Production,
339:130587.
Howell, S., Rezgui, Y., Hippolyte, J.-L., Jayan, B., and Li,
H. (2017). Towards the next generation of smart grids:
Semantic and holonic multi-agent management of dis-
tributed energy resources. Renewable and Sustainable
Energy Reviews, 77:193–214.
Huang, Y., Hasan, N., Deng, C., and Bao, Y. (2022). Mul-
tivariate empirical mode decomposition based hybrid
model for day-ahead peak load forecasting. Energy,
239:122245.
Kanakadhurga, D. and Prabaharan, N. (2022). Demand side
management in microgrid: A critical review of key
issues and recent trends. Renewable and Sustainable
Energy Reviews, 156:111915.
Koestler, A. (1967). The Ghost in the Machine. Macmillan.
Liu, C., Chau, K. T., Wu, D., and Gao, S. (2013). Opportu-
nities and challenges of vehicle-to-home, vehicle-to-
vehicle, and vehicle-to-grid technologies. Proceed-
ings of the IEEE, 101(11):2409–2427.
Mar
´
ık, V., Lastra, J. M., and Skobelev, P. (2013). Indus-
trial applications of holonic and multi-agent systems.
In Proceedings of Ll” DEXA International Workshop.
Springer.
Mella, P. (2009). The Holonic Revolution Holons, Hol-
archies and Holonic Networks. The Ghost in the Pro-
duction Machine.
Negeri, E., Baken, N., and Popov, M. (2013). Holonic archi-
tecture of the smart grid. Smart Grid and Renewable
Energy, 04:202–212.
Pallonetto, F., Jin, C., and Mangina, E. (2022). Forecast
electricity demand in commercial building with ma-
chine learning models to enable demand response pro-
grams. Energy and AI, 7:100121.
Ramchurn, S., Vytelingum, P., Rogers, A., and Jennings,
N. (2012). Putting the ’Smarts’ into the Smart Grid:
A Grand Challenge for Artificial Intelligence. Com-
munications of The ACM - CACM, 55:86–97.
Spencer, T., Berghmans, N., and Sartor, O. (2017). Coal
transitions in China’s power sector: A plant-level as-
sessment of stranded assets and retirement pathways.
Coal transitions, 12/17:21.
Taleb, I., Guerard, G., Fauberteau, F., and Nguyen, N.
(2022). A flexible deep learning method for energy
forecasting. Energies, 15(11).
UNFCCC (2021). The Paris Agreement | UN-
FCCC. https://unfccc.int/process-and-meetings/the-
paris-agreement/the-paris-agreement.
Wallis, A., Hauke, S., Egert, R., and M
¨
uhlh
¨
auser, M.
(2020). A framework for strategy selection of atomic
entities in the holonic smart grid.
ICAART 2023 - 15th International Conference on Agents and Artificial Intelligence
134