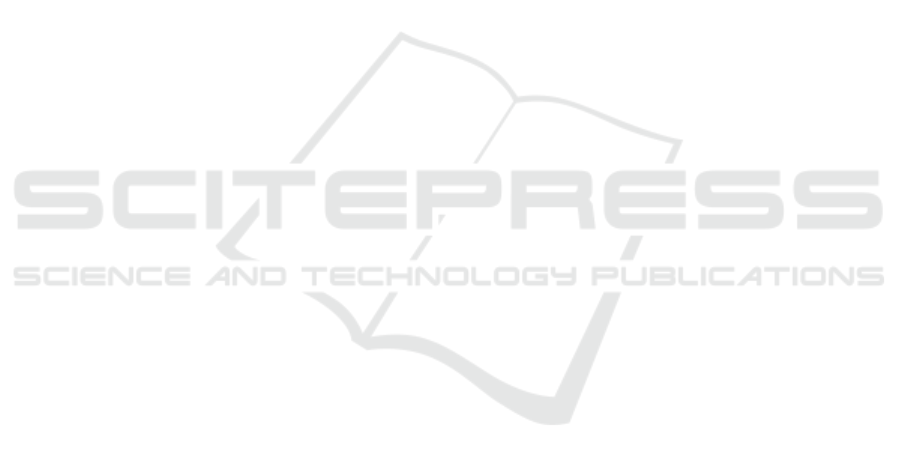
hend and confirm the system’s decision.
In the current study, blood vessel segments that
form a loop or overlap were not considered when cal-
culating the curvature index of the vessels. In addi-
tion, the present method is trained and evaluated with
a small number of retinal image datasets for three lev-
els of ROP-Plus disease classification. Therefore, in
the future, we may enhance the proposed techniques
to address overlapping problems with more retinal
image datasets and additional disease classification
levels.
REFERENCES
Chiang, M. F., Quinn, G. E., Fielder, A. R., Ostmo, S. R.,
Chan, R. P., Berrocal, A., Binenbaum, G., Blair, M.,
Campbell, J. P., Capone Jr, A., et al. (2021). Inter-
national classification of retinopathy of prematurity.
Ophthalmology, 128(10):e51–e68.
Doi, K. (2007). Computer-aided diagnosis in medical imag-
ing: historical review, current status and future po-
tential. Computerized medical imaging and graphics,
31(4-5):198–211.
Grisan, E., Foracchia, M., and Ruggeri, A. (2008). A
novel method for the automatic grading of retinal ves-
sel tortuosity. IEEE transactions on medical imaging,
27(3):310–319.
Hart, W. E., Goldbaum, M., Cote, B., Kube, P., and Nelson,
M. R. (1997). Automated measurement of retinal vas-
cular tortuosity. In Proceedings of the AMIA Annual
Fall Symposium, page 459. American Medical Infor-
matics Association.
Heneghan, C., Flynn, J., O’Keefe, M., and Cahill, M.
(2002). Characterization of changes in blood ves-
sel width and tortuosity in retinopathy of prematu-
rity using image analysis. Medical image analysis,
6(4):407–429.
Isola, P., Zhu, J.-Y., Zhou, T., and Efros, A. A. (2018).
Image-to-image translation with conditional adversar-
ial networks.
Jocher, G., Stoken, A., Borovec, J., NanoCode012, Chaura-
sia, A., TaoXie, Changyu, L., V, A., Laughing,
tkianai, yxNONG, Hogan, A., lorenzomammana,
AlexWang1900, Hajek, J., Diaconu, L., Marc, Kwon,
Y., oleg, wanghaoyang0106, Defretin, Y., Lohia, A.,
ml5ah, Milanko, B., Fineran, B., Khromov, D., Yiwei,
D., Doug, Durgesh, and Ingham, F. (2021). ultralyt-
ics/yolov5: v5.0 - YOLOv5-P6 1280 models, AWS,
Supervise.ly and YouTube integrations.
Kumar, V., Patel, H., Azad, S., Paul, K., Surve, A., and
Chawla, R. (2022). Dl-assisted rop screening tech-
nique. In Gehin, C., Wacogne, B., Douplik, A.,
Lorenz, R., Bracken, B., Pesquita, C., Fred, A., and
Gamboa, H., editors, Biomedical Engineering Systems
and Technologies, pages 236–258, Cham. Springer In-
ternational Publishing.
Kumar, V., Patel, H., Paul, K., Surve, A., Azad, S., and
Chawla, R. (2021). Deep learning assisted retinopathy
of prematurity screening technique. In HEALTHINF,
pages 234–243.
Kumar., V., Patel., H., Paul., K., Surve., A., Azad., S., and
Chawla., R. (2022). Improved blood vessels segmen-
tation of retinal image of infants. In Proceedings of the
15th International Joint Conference on Biomedical
Engineering Systems and Technologies - HEALTH-
INF,, pages 142–153. INSTICC, SciTePress.
MathWorks (1994-2023). Morphological operations on
binary images - matlab bwmorph. https://www.
mathworks.com/help/images/ref/bwmorph.html. (Ac-
cessed on 01/07/2023).
Oloumi, F., Rangayyan, R. M., and Ells, A. L. (2016).
Computer-aided diagnosis of retinopathy in retinal
fundus images of preterm infants via quantification
of vascular tortuosity. Journal of Medical Imaging,
3(4):044505.
Organization, W. H. et al. (2019). World report on vision.
Technical report, Geneva: World Health Organization.
Razzak, M. I., Naz, S., and Zaib, A. (2018). Deep learning
for medical image processing: Overview, challenges
and the future. Classification in BioApps, pages 323–
350.
Reid, J. E. and Eaton, E. (2019). Artificial intelligence for
pediatric ophthalmology. Current opinion in ophthal-
mology, 30(5):337–346.
Ronneberger, O., Fischer, P., and Brox, T. (2015). U-net:
Convolutional networks for biomedical image seg-
mentation.
Scruggs, B. A., Chan, R. P., Kalpathy-Cramer, J., Chiang,
M. F., and Campbell, J. P. (2020). Artificial intelli-
gence in retinopathy of prematurity diagnosis. Trans-
lational Vision Science & Technology, 9(2):5–5.
Sukkaew, L., Uyyanonvara, B., Makhanov, S. S., Bar-
man, S., and Pangputhipong, P. (2008). Automatic
tortuosity-based retinopathy of prematurity screening
system. IEICE transactions on information and sys-
tems, 91(12):2868–2874.
Tian, P., Guo, Y., Kalpathy-Cramer, J., Ostmo, S., Camp-
bell, J. P., Chiang, M. F., Dy, J., Erdogmus, D., and
Ioannidis, S. (2019). A severity score for retinopa-
thy of prematurity. In Proceedings of the 25th
ACM SIGKDD International Conference on Knowl-
edge Discovery & Data Mining, KDD ’19, page
1809–1819, New York, NY, USA. Association for
Computing Machinery.
Ting, D. S. W., Pasquale, L. R., Peng, L., Campbell, J. P.,
Lee, A. Y., Raman, R., Tan, G. S. W., Schmetterer, L.,
Keane, P. A., and Wong, T. Y. (2019). Artificial intel-
ligence and deep learning in ophthalmology. British
Journal of Ophthalmology, 103(2):167–175.
Wang, G., Li, M., Yun, Z., Duan, Z., Ma, K., Luo, Z., Xiao,
P., and Yuan, J. (2021). A novel multiple subdivision-
based algorithm for quantitative assessment of reti-
nal vascular tortuosity. Experimental Biology and
Medicine, 246(20):2222–2229.
Deep Learning Assisted Plus Disease Screening of Retinal Image of Infants
545