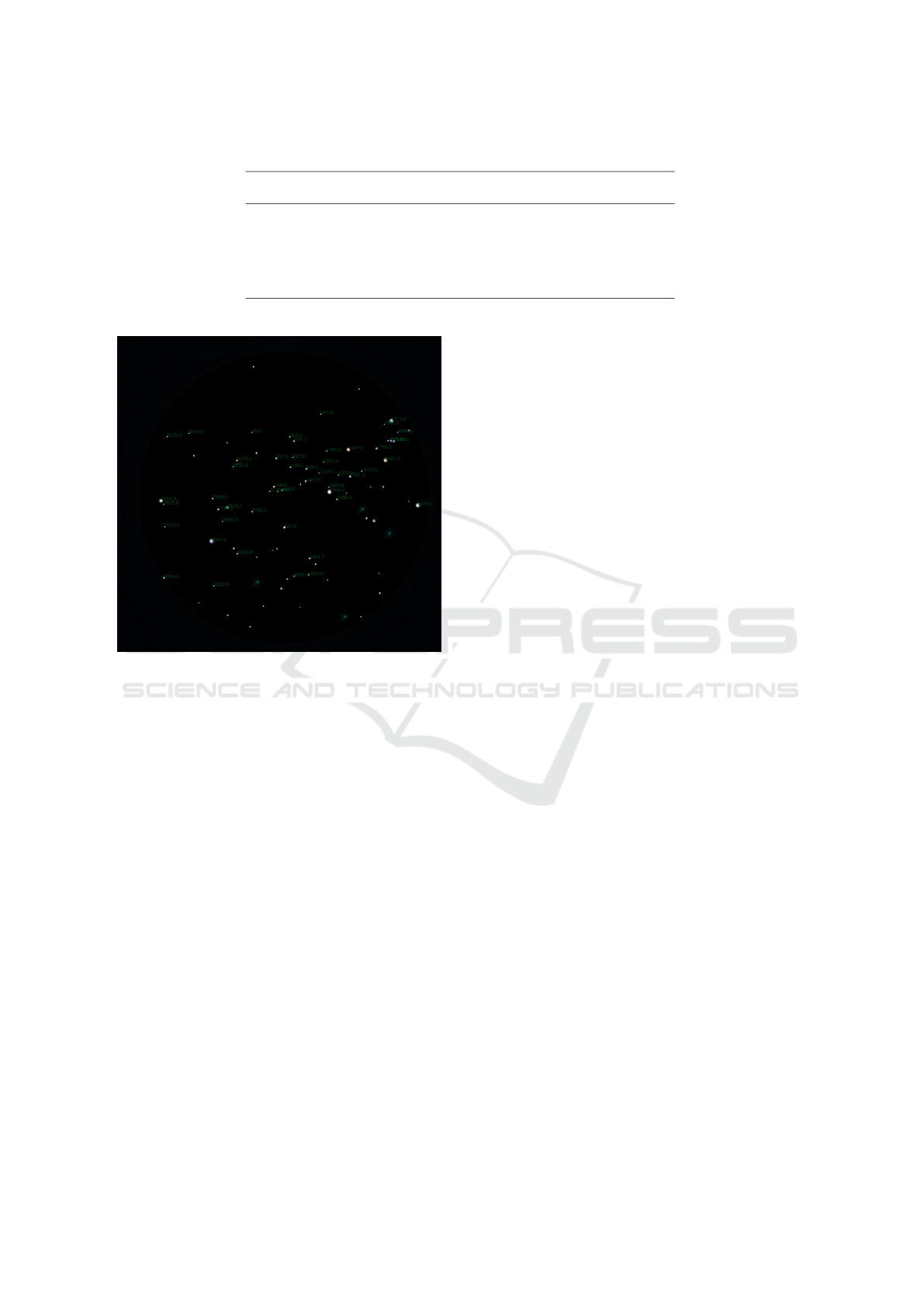
Table 3: Classification results and thresholding impact.
Threshold
Correct
Identifications
% Change
(Total)
Incorrect
Identifications
% Change
(Total)
Baseline 859287 N/A 149423 N/A
0.8 770485 10.33% 19874 86.70%
0.9 741632 13.69% 12039 91.94%
0.95 713120 17.01% 7184 95.19%
0.99 640618 25.45% 2119 98.51%
0.999 523322 39.10% 221 99.85%
Figure 11: Synthetic image results with 0.999 confi-
dence thresholding, showing the removal of all miss-
identifications in the image.
method by which we can garner realistic night-sky
images from a variety of viewpoints as well as the
corresponding information required to utilise them as
a labelled dataset of stars.
We create a data augmenter to avoid pre-
computing training patterns whilst allowing for
greater freedoms with training and testing and para-
metrically explore tolerance towards noise. The fi-
nal implementation performs well on simulated star
patterns and is still able to correctly identify the ma-
jority of stars in the markedly noisier synthetic im-
ages, we threshold our images to a visible magnitude
of 3.0 in order to appropriately simulate the capabili-
ties of inexpensive cameras with low exposure times,
an important consideration when examining this tech-
nology through the lens of celestial navigation from
the Earths surface. Whilst miss-identifications do oc-
cur, we are able to drastically reduce these by utilising
confidence thresholds, a necessary step if the method
were to be used for navigational purposes where miss-
identifications can be damaging.
REFERENCES
Jiang, J., Ji, F., Yan, J., Sun, L., and Wei, X. (2015).
Redundant-coded radial and neighbor star pattern
identification algorithm. IEEE Transactions on
Aerospace and Electronic Systems, 51(4):2811–2822.
Liebe, C. (1993). Pattern recognition of star constellations
for spacecraft applications. IEEE Aerospace and Elec-
tronic Systems Magazine, 8(1):31–39.
Mortari, D., Samaan, M. A., Bruccoleri, C., and Junkins,
J. L. (2004). The pyramid star identification tech-
nique. NAVIGATION, 51(3):171–183.
Na, M., Zheng, D., and Jia, P. (2009). Modified grid algo-
rithm for noisy all-sky autonomous star identification.
IEEE Transactions on Aerospace and Electronic Sys-
tems, 45(2):516–522.
Nabi, A., Foitih, Z., and Mohammed El Amine, C. (2019).
Improved triangular-based star pattern recognition al-
gorithm for low-cost star trackers. Journal of King
Saud University.
Padgett, C. and Kreutz-Delgado, K. (1997). A grid al-
gorithm for autonomous star identification. IEEE
Transactions on Aerospace and Electronic Systems,
33(1):202–213.
Rijlaarsdam, D., Yous, H., Byrne, J., Oddenino, D., Fu-
rano, G., and Moloney, D. (2020). A survey of lost-in-
space star identification algorithms since 2009. Sen-
sors, 20(9).
Stetson, P. B. (1987). DAOPHOT: A Computer Program
for Crowded-Field Stellar Photometry. Publications
of the Astronomical Society of the Pacific, 99:191.
van Bezooijen, R. (1989). A star pattern recognition al-
gorithm for autonomous attitude determination. IFAC
Proceedings Volumes, 22(7):51–58. IFAC Symposium
on Automatic Control in Aerospace, Tsukuba, Japan,
17-21 July 1989.
Wang, G., Li, J., and Wei, X. (2018). Star identifica-
tion based on hash map. IEEE Sensors Journal,
18(4):1591–1599.
Xu, L., Jiang, J., and Liu, L. (2019). Rpnet: A representa-
tion learning based star identification algorithm. IEEE
Access, PP:1–1.
Yang, S., Liu, L., Zhou, J., Zhao, Y., Hua, G., Sun, H.,
and Zheng, N. (2022). Robust and efficient star iden-
tification algorithm based on 1-d convolutional neural
network. IEEE Transactions on Aerospace and Elec-
tronic Systems, 58(5):4156–4167.
Zhang, G., Wei, X., and Jiang, J. (2008). Full-sky au-
tonomous star identification based on radial and cyclic
features of star pattern. Image and Vision Computing,
26(7):891–897.
ICPRAM 2023 - 12th International Conference on Pattern Recognition Applications and Methods
184