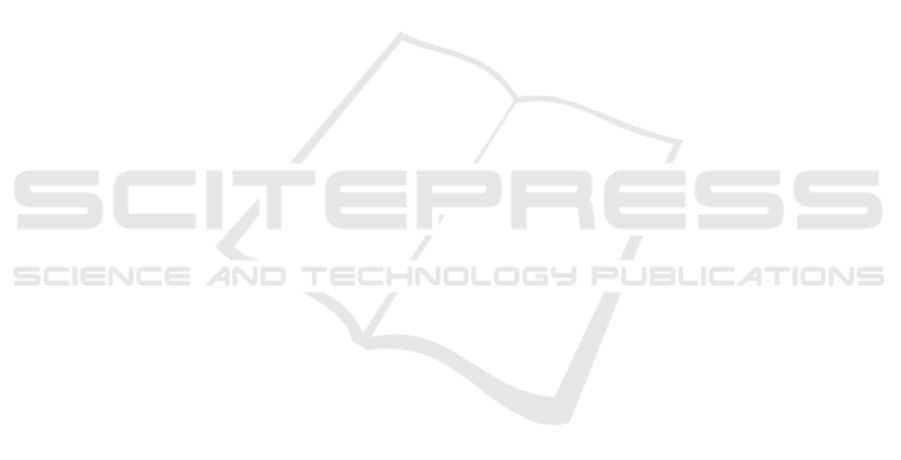
problems with invertible neural networks. CoRR,
abs/1808.04730.
Ardizzone, L., L
¨
uth, C., Kruse, J., Rother, C., and K
¨
othe,
U. (2019). Guided image generation with conditional
invertible neural networks. CoRR, abs/1907.02392.
Cheng, Z., Sun, H., Takeuchi, M., and Katto, J. (2020).
Learned image compression with discretized gaus-
sian mixture likelihoods and attention modules. 2020
IEEE/CVF Conference on Computer Vision and Pat-
tern Recognition (CVPR), pages 7936–7945.
Ding, D., Ma, Z., Chen, D., Chen, Q., Liu, Z., and Zhu,
F. (2021). Advances in video compression system us-
ing deep neural network: A review and case studies.
Proceedings of the IEEE, 109(9):1494–1520.
Dinh, L., Krueger, D., and Bengio, Y. (2015). Nice: Non-
linear independent components estimation. CoRR,
abs/1410.8516.
Dinh, L., Sohl-Dickstein, J., and Bengio, S. (2016). Density
estimation using real NVP. CoRR, abs/1605.08803.
Dosselmann, R. and Yang, X. D. (2005). Existing and
emerging image quality metrics. In Canadian Confer-
ence on Electrical and Computer Engineering, 2005.,
pages 1906–1913.
Duda, J. (2009). Asymmetric numeral systems. CoRR,
abs/0902.0271.
Hor
´
e, A. and Ziou, D. (2010). Image quality metrics: Psnr
vs. ssim. In 2010 20th International Conference on
Pattern Recognition, pages 2366–2369.
Huang, G., Liu, Z., and Weinberger, K. Q. (2016).
Densely connected convolutional networks. CoRR,
abs/1608.06993.
Kingma, D. P. and Ba, J. (2015). Adam: A method for
stochastic optimization. CoRR, abs/1412.6980.
Kingma, D. P. and Dhariwal, P. (2018). Glow: Gener-
ative flow with invertible 1x1 convolutions. ArXiv,
abs/1807.03039.
Liu, D., Li, Y., Lin, J., Li, H., and Wu, F. (2021). Deep
learning-based video coding. ACM Computing Sur-
veys, 53(1):1–35.
Lugmayr, A., Danelljan, M., Gool, L. V., and Timofte, R.
(2020). Srflow: Learning the super-resolution space
with normalizing flow. CoRR, abs/2006.14200.
Mercat, A., Viitanen, M., and Vanne, J. (2020). Uvg dataset:
50/120fps 4k sequences for video codec analysis and
development. Proceedings of the 11th ACM Multime-
dia Systems Conference.
Minnen, D. C., Ball
´
e, J., and Toderici, G. (2018). Joint au-
toregressive and hierarchical priors for learned image
compression. ArXiv, abs/1809.02736.
Networking, C. V. (2016). Cisco global cloud index: Fore-
cast and methodology, 2015-2020. white paper. Cisco
Public, San Jose, page 2016.
Rassool, R. (2017). Vmaf reproducibility: Validating a per-
ceptual practical video quality metric. In 2017 IEEE
International Symposium on Broadband Multimedia
Systems and Broadcasting (BMSB), pages 1–2.
Shi, W., Caballero, J., Husz
´
ar, F., Totz, J., Aitken, A. P.,
Bishop, R., Rueckert, D., and Wang, Z. (2016). Real-
time single image and video super-resolution using
an efficient sub-pixel convolutional neural network.
CoRR, abs/1609.05158.
Skodras, A., Christopoulos, C., and Ebrahimi, T. (2001).
The jpeg 2000 still image compression standard. IEEE
Signal Processing Magazine, 18(5):36–58.
Sullivan, G. J., Ohm, J.-R., Han, W.-J., and Wiegand, T.
(2012). Overview of the high efficiency video coding
(hevc) standard. IEEE Transactions on Circuits and
Systems for Video Technology, 22(12):1649–1668.
Wallace, G. (1992). The jpeg still picture compression stan-
dard. IEEE Transactions on Consumer Electronics,
38(1):xviii–xxxiv.
Wang, Y., Inguva, S., and Adsumilli, B. (2019). Youtube
ugc dataset for video compression research. 2019
IEEE 21st International Workshop on Multimedia Sig-
nal Processing (MMSP), pages 1–5.
Wang, Y., Xiao, M., Liu, C., Zheng, S., and Liu, T. (2020).
Modeling lost information in lossy image compres-
sion. CoRR, abs/2006.11999.
Wang, Z., Simoncelli, E., and Bovik, A. (2003). Multiscale
structural similarity for image quality assessment. vol-
ume 2, pages 1398 – 1402 Vol.2.
Wiegand, T., Sullivan, G., Bjontegaard, G., and Luthra, A.
(2003). Overview of the h.264/avc video coding stan-
dard. IEEE Transactions on Circuits and Systems for
Video Technology, 13(7):560–576.
Xiao, M., Zheng, S., Liu, C., Wang, Y., He, D., Ke, G.,
Bian, J., Lin, Z., and Liu, T.-Y. (2020). Invertible im-
age rescaling. ArXiv, abs/2005.05650.
Xie, Y., Cheng, K. L., and Chen, Q. (2021). Enhanced in-
vertible encoding for learned image compression. In
Proceedings of the 29th ACM International Confer-
ence on Multimedia, MM ’21, page 162–170, New
York, NY, USA. Association for Computing Machin-
ery.
Xue, T., Chen, B., Wu, J., Wei, D., and Freeman,
W. T. (2019). Video enhancement with task-oriented
flow. International Journal of Computer Vision,
127(8):1106–1125.
ICPRAM 2023 - 12th International Conference on Pattern Recognition Applications and Methods
564