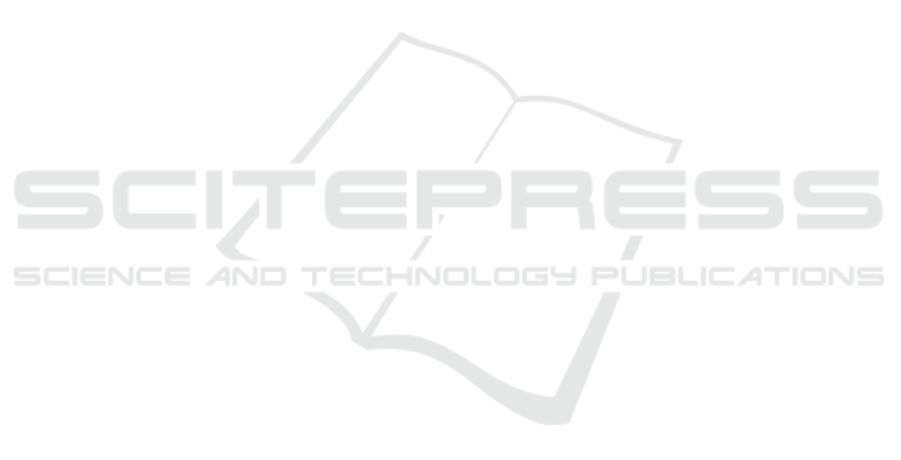
Lin, T.-Y., Goyal, P., Girshick, R. B., He, K., and Doll
´
ar, P.
(2020). Focal loss for dense object detection. IEEE
Transactions on Pattern Analysis and Machine Intel-
ligence, 42:318–327.
Liu, Z., Hu, H., Lin, Y., Yao, Z., Xie, Z., Wei, Y., Ning,
J., Cao, Y., Zhang, Z., Dong, L., Wei, F., and Guo,
B. (2022a). Swin transformer v2: Scaling up capac-
ity and resolution. IEEE/CVF CVPR, pages 11999–
12009.
Liu, Z., Lin, Y., Cao, Y., Hu, H., Wei, Y., Zhang, Z., Lin,
S., and Guo, B. (2021). Swin transformer: Hierarchi-
cal vision transformer using shifted windows. 2021
IEEE/CVF ICCV, pages 9992–10002.
Liu, Z., Mao, H., Wu, C., Feichtenhofer, C., Darrell, T.,
and Xie, S. (2022b). A convnet for the 2020s. pages
11976–11986.
Nikita Kozodoi, Gilberto Titericz, H. G. (2020). 11th
place solution writeup. https://www.kaggle.
com/competitions/siim-isic-melanoma-classification/
discussion/175624. Accessed: 2022-04-30.
Pacheco, A. G. C., Sastry, C. S., Trappenberg, T. P., Oore,
S., and Krohling, R. A. (2020). On out-of-distribution
detection algorithms with deep neural skin cancer
classifiers. IEEE/CVF CVPRW, pages 3152–3161.
Potdar, K., Pardawala, T. S., and Pai, C. D. (2017). A com-
parative study of categorical variable encoding tech-
niques for neural network classifiers. International
Journal of Computer Applications, 175:7–9.
Richard, M. D. and Lippmann, R. (1991). Neural network
classifiers estimate bayesian a posteriori probabilities.
Neural Computation, 3:461–483.
Rotemberg, V. M., Kurtansky, N. R., Betz-Stablein, B., Caf-
fery, L. J., Chousakos, E., Codella, N. C. F., Combalia,
M., Dusza, S. W., Guitera, P., Gutman, D., Halpern,
A. C., Kittler, H., K
¨
ose, K., Langer, S. G., Liopryis,
K., Malvehy, J., Musthaq, S., Nanda, J., Reiter, O.,
Shih, G., Stratigos, A. J., Tschandl, P., Weber, J., and
Soyer, H. P. (2021). A patient-centric dataset of im-
ages and metadata for identifying melanomas using
clinical context. Scientific Data, 8.
Russakovsky, O., Deng, J., Su, H., Krause, J., Satheesh, S.,
Ma, S., Huang, Z., Karpathy, A., Khosla, A., Bern-
stein, M. S., Berg, A. C., and Fei-Fei, L. (2015). Im-
agenet large scale visual recognition challenge. Inter-
national Journal of Computer Vision, 115:211–252.
Sarker, M. M. K., Moreno-Garc
´
ıa, C. F., Ren, J., and
Elyan, E. (2022). Transslc: Skin lesion classifica-
tion in dermatoscopic images using transformers. In
Annual Conference on Medical Image Understanding
and Analysis, pages 651–660. Springer.
Sastry, C. S. and Oore, S. (2019). Detecting out-of-
distribution examples with in-distribution examples
and gram matrices. ArXiv, abs/1912.12510.
Shanmugam, D., Blalock, D. W., Balakrishnan, G., and
Guttag, J. V. (2020). When and why test-time aug-
mentation works. ArXiv, abs/2011.11156.
Smith, L. N. (2018). A disciplined approach to neu-
ral network hyper-parameters: Part 1 - learning rate,
batch size, momentum, and weight decay. ArXiv,
abs/1803.09820.
Smith, L. N. and Topin, N. (2019). Super-convergence: very
fast training of neural networks using large learning
rates. In Defense + Commercial Sensing.
Steppan, J. and Hanke, S. (2021). Analysis of skin lesion
images with deep learning. ArXiv, abs/2101.03814.
Strzelecki, M., Strakowska, M., Kozłowski, M., Urba
´
nczyk,
T., Wielowieyska-Szybi
´
nska, D., and Kociolek, M.
(2021). Skin lesion detection algorithms in whole
body images. Sensors (Basel, Switzerland), 21.
Sun, X., Yang, J., Sun, M., and Wang, K. (2016). A
benchmark for automatic visual classification of clin-
ical skin disease images. In ECCV.
Szegedy, C., Ioffe, S., Vanhoucke, V., and Alemi, A. A.
(2017). Inception-v4, inception-resnet and the impact
of residual connections on learning. In AAAI.
Tan, M. and Le, Q. V. (2019). Efficientnet: Rethink-
ing model scaling for convolutional neural networks.
ArXiv, abs/1905.11946.
Touvron, H., Cord, M., Douze, M., Massa, F., Sablayrolles,
A., and J’egou, H. (2021a). Training data-efficient im-
age transformers & distillation through attention. In
ICML.
Touvron, H., Cord, M., Sablayrolles, A., Synnaeve, G., and
J’egou, H. (2021b). Going deeper with image trans-
formers. 2021 IEEE/CVF ICCV, pages 32–42.
Tschandl, P., Rosendahl, C., and Kittler, H. (2018). The
ham10000 dataset, a large collection of multi-source
dermatoscopic images of common pigmented skin le-
sions. Scientific Data, 5.
Vaswani, A., Shazeer, N. M., Parmar, N., Uszkoreit, J.,
Jones, L., Gomez, A. N., Kaiser, L., and Polosukhin,
I. (2017). Attention is all you need. In NIPS.
Walter, F. M., Prevost, A. T., Vasconcelos, J. C., Hall, P.,
Burrows, N. P., Morris, H. C., Kinmonth, A. L., and
Emery, J. D. (2013). Using the 7-point checklist as
a diagnostic aid for pigmented skin lesions in general
practice: a diagnostic validation study. The British
journal of general practice : the journal of the Royal
College of General Practitioners, 63 610:e345–53.
WHO, W. H. O. (2017). Radiation: Ultraviolet (uv)
radiation and skin cancer. https://www.who.int/news-
room/questions-and-answers/item/radiation-
ultraviolet-(uv)-radiation-and-skin-cancer. Accessed:
2022-06-30.
Xie, Q., Hovy, E. H., Luong, M.-T., and Le, Q. V. (2020).
Self-training with noisy student improves imagenet
classification. IEEE/CVF CVPR, pages 10684–10695.
Xie, S., Girshick, R. B., Doll
´
ar, P., Tu, Z., and He, K.
(2017). Aggregated residual transformations for deep
neural networks. IEEE CVPR, pages 5987–5995.
Yalniz, I. Z., J
´
egou, H., Chen, K., Paluri, M., and Mahajan,
D. K. (2019). Billion-scale semi-supervised learning
for image classification. ArXiv, abs/1905.00546.
Yuan, L., Hou, Q., Jiang, Z., Feng, J., and Yan, S. (2022).
Volo: Vision outlooker for visual recognition. IEEE
Trans. PAMI, PP.
VISAPP 2023 - 18th International Conference on Computer Vision Theory and Applications
314