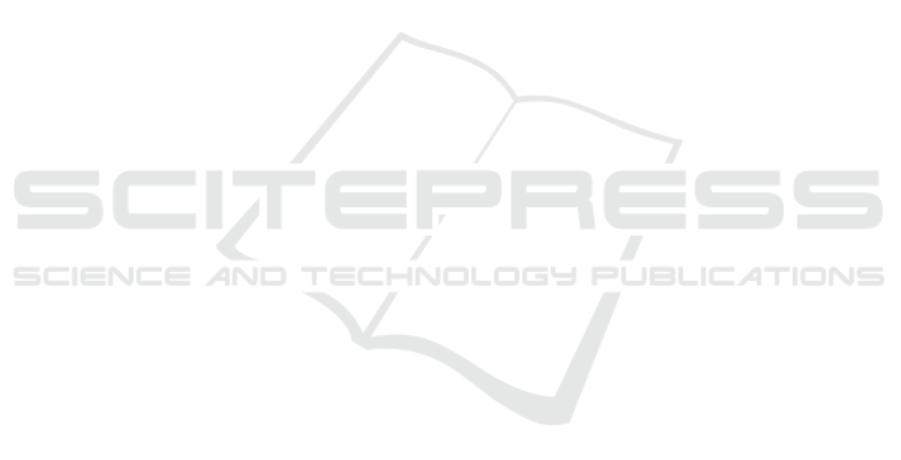
lies, including also software specific indicators. As
expected, the anomaly detection data processing re-
quires a substantial computing power. The optimiza-
tion in terms or efficiency is an interesting field, that
could drive to lower detection rates and could be used
to achieve a more reasonable anomaly detection for
elements that will achieve lower security levels.
ACKNOWLEDGEMENTS
This work has been funded by the European Union’s
Horizon 2020 research and innovation programme
(grant agreement No 871465 (UP2DATE)).
REFERENCES
Agelastos, A., Allan, B., Brandt, J., Cassella, P., Enos, J.,
Fullop, J., Gentile, A., Monk, S., Naksinehaboon, N.,
Ogden, J., et al. (2014). The lightweight distributed
metric service: a scalable infrastructure for continu-
ous monitoring of large scale computing systems and
applications. In SC’14: Proceedings of the Interna-
tional Conference for High Performance Computing,
Networking, Storage and Analysis, pages 154–165.
IEEE.
Agirre, I., Yarza, I., Mugarza, I., Binchi, J., Onaindia,
P., Poggi, T., Cazorla, F. J., Kosmidis, L., Gr
¨
uttner,
K., Uven, P., et al. (2021). Safe and secure soft-
ware updates on high-performance mixed-criticality
systems: The up2date approach. Microprocessors and
Microsystems, 87:104351.
Cherkasova, L., Ozonat, K., Mi, N., Symons, J., and Smirni,
E. (2009). Automated anomaly detection and per-
formance modeling of enterprise applications. ACM
Transactions on Computer Systems (TOCS), 27(3):1–
32.
Chiara, P. G. (2022). The cyber resilience act: the eu com-
mission’s proposal for a horizontal regulation on cy-
bersecurity for products with digital elements. Inter-
national Cybersecurity Law Review, pages 1–18.
Ding, Y., Xia, X., Chen, S., and Li, Y. (2018). A malware
detection method based on family behavior graph.
Computers & Security, 73:73–86.
Hyndman, R. J., Wang, E., and Laptev, N. (2015). Large-
scale unusual time series detection. In 2015 IEEE
International Conference on Data Mining Workshop
(ICDMW), pages 1616–1619.
Ibidunmoye, O., Hern
´
andez-Rodriguez, F., and Elmroth, E.
(2015). Performance anomaly detection and bottle-
neck identification. ACM Computing Surveys (CSUR),
48(1):1–35.
Jayathilaka, H., Krintz, C., and Wolski, R. (2017). Perfor-
mance monitoring and root cause analysis for cloud-
hosted web applications. In Proceedings of the 26th
International Conference on World Wide Web, pages
469–478.
Jayathilaka, H., Krintz, C., and Wolski, R. (2020). De-
tecting performance anomalies in cloud platform ap-
plications. IEEE Transactions on Cloud Computing,
8(3):764–777.
Kang, H., Zhu, X., and Wong, J. L. (2012). {DAPA}: Diag-
nosing application performance anomalies for virtual-
ized infrastructures. In 2nd USENIX Workshop on Hot
Topics in Management of Internet, Cloud, and Enter-
prise Networks and Services (Hot-ICE 12).
Kim, H.-A. and Karp, B. (2004). Autograph: Toward au-
tomated, distributed worm signature detection. In
USENIX security symposium, volume 286. San Diego,
CA.
Kolbitsch, C., Comparetti, P. M., Kruegel, C., Kirda, E.,
Zhou, X., and Wang, X. (2009). Effective and efficient
malware detection at the end host. In Proceedings of
the 18th Conference on USENIX Security Symposium,
SSYM’09, page 351–366, USA. USENIX Associa-
tion.
Lee, S., Levanti, K., and Kim, H. S. (2014). Network
monitoring: Present and future. Computer Networks,
65:84–98.
L
´
opez, J. (2010). Monitorizaci
´
on con pandora fms 3.
Todo linux: la revista mensual para entusiastas de
GNU/LINUX, (115):20–23.
Mugarza, I., Parra, J., and Jacob, E. (2017). Software up-
dates in safety and security co-engineering. In Inter-
national Conference on Computer Safety, Reliability,
and Security, pages 199–210. Springer.
Mugarza, I., Yarza, I., Agirre, I., Lussiana, F., and Botta, S.
(2021). Safety and security concept for software up-
dates on mixed-criticality systems. In 2021 5th Inter-
national Conference on System Reliability and Safety
(ICSRS), pages 171–180. IEEE.
Samir, A. and Pahl, C. (2019). Detecting and predicting
anomalies for edge cluster environments using hidden
markov models. In 2019 Fourth International Con-
ference on Fog and Mobile Edge Computing (FMEC),
pages 21–28. IEEE.
Samir, A. and Pahl, C. (2020). Detecting and localizing
anomalies in container clusters using markov models.
Electronics, 9(1):64.
Singh, S., Estan, C., Varghese, G., and Savage, S. (2004).
Automated worm fingerprinting. In OSDI, volume 4,
pages 4–4.
Tuncer, O., Ates, E., Zhang, Y., Turk, A., Brandt, J., Leung,
V. J., Egele, M., and Coskun, A. K. (2018). Online di-
agnosis of performance variation in hpc systems using
machine learning. IEEE Transactions on Parallel and
Distributed Systems, 30(4):883–896.
IoTBDS 2023 - 8th International Conference on Internet of Things, Big Data and Security
122