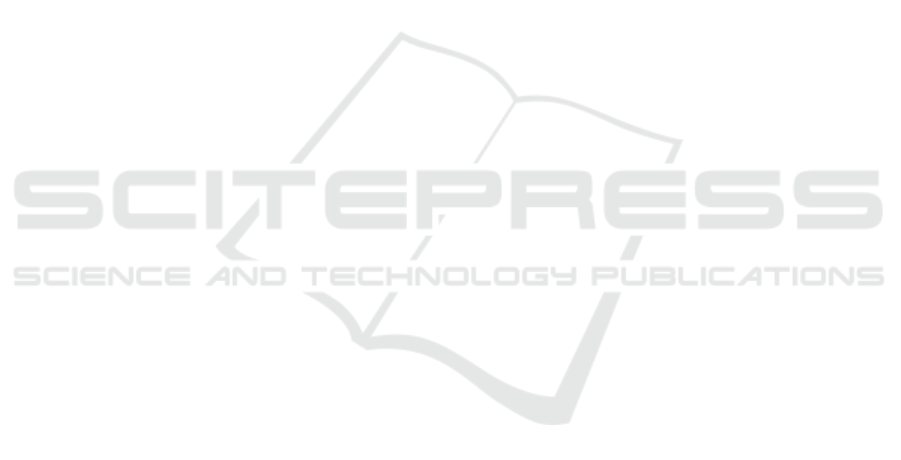
REFERENCES
An, Z., Li, S., Wang, J., Xin, Y., and Xu, K. (2019). Gener-
alization of deep neural network for bearing fault di-
agnosis under different working conditions using mul-
tiple kernel method. Neurocomputing, 352:42–53.
Gawde, S., Patil, S., Kumar, S., Kamat, P., Kotecha, K., and
Abraham, A. (2022). Multi-fault diagnosis of indus-
trial rotating machines using data-driven approach: A
review of two decades of research.
Gretton, A., Sriperumbudur, B. K., Sejdinovic, D., Strath-
mann, H., Balakrishnan, S., Pontil, M., and Fukumizu,
K. (2012). Optimal kernel choice for large-scale two-
sample tests. In NIPS.
Kancharla, C. R., Vankeirsbilck, J., Vanoost, D., Boydens,
J., and Hallez, H. (2022). Latent dimensions of auto-
encoder as robust features for inter-conditional bear-
ing fault diagnosis. Applied Sciences, 12(3):965.
Lessmeier, C., Kimotho, J., Zimmer, D., and Sextro, W.
(2016). Condition monitoring of bearing damage in
electromechanical drive systems by using motor cur-
rent signals of electric motors: A benchmark data set
for data-driven classification.
Li, J., Shen, C., Kong, L., Wang, D., Xia, M., and Zhu,
Z. (2022). A new adversarial domain generalization
network based on class boundary feature detection for
bearing fault diagnosis. IEEE Transactions on Instru-
mentation and Measurement, 71:1–9.
Li, X., Hu, Y., Zheng, J., Li, M., and Ma, W. (2021). Cen-
tral moment discrepancy based domain adaptation for
intelligent bearing fault diagnosis. Neurocomputing,
429:12–24.
Liu, C. and Gryllias, K. (2022). Simulation-driven do-
main adaptation for rolling element bearing fault di-
agnosis. IEEE Transactions on Industrial Informatics,
18(9):5760–5770.
Long, M., Zhu, H., Wang, J., and Jordan, M. I. (2016). Deep
transfer learning with joint adaptation networks.
Pan, S. J. and Yang, Q. (2009). A survey on transfer learn-
ing. IEEE Transactions on knowledge and data engi-
neering, 22(10):1345–1359.
Qiu, T., Chi, J., Zhou, X., Ning, Z., Atiquzzaman, M.,
and Wu, D. O. (2020). Edge computing in industrial
internet of things: Architecture, advances and chal-
lenges. IEEE Communications Surveys & Tutorials,
22(4):2462–2488.
Salah, A. A., Dorrell, D. G., and Guo, Y. (2019). A re-
view of the monitoring and damping unbalanced mag-
netic pull in induction machines due to rotor eccen-
tricity. IEEE Transactions on Industry Applications,
55(3):2569–2580.
Shan, N., Xu, X., Bao, X., and Qiu, S. (2022). Fast fault
diagnosis in industrial embedded systems based on
compressed sensing and deep kernel extreme learning
machines. Sensors, 22(11):3997.
Smith, W. A. and Randall, R. B. (2015). Rolling ele-
ment bearing diagnostics using the case western re-
serve university data: A benchmark study. Mechani-
cal Systems and Signal Processing, 64-65:100–131.
Wan, L., Li, Y., Chen, K., Gong, K., and Li, C. (2022).
A novel deep convolution multi-adversarial domain
adaptation model for rolling bearing fault diagnosis.
Measurement, 191:110752.
Wang, X., Han, Y., Leung, V. C. M., Niyato, D., Yan, X.,
and Chen, X. (2020). Convergence of edge computing
and deep learning: A comprehensive survey. IEEE
Communications Surveys & Tutorials, 22(2):869–904.
Wu, Y., Zhao, R., Ma, H., He, Q., Du, S., and Wu, J. (2022).
Adversarial domain adaptation convolutional neural
network for intelligent recognition of bearing faults.
Measurement, 195:111150.
Yang, Y. and Li, C. (2021). Quantitative analysis of
the generalization ability of deep feedforward neural
networks. Journal of Intelligent & Fuzzy Systems,
40(3):4867–4876.
Zhang, Q., Zhao, Z., Zhang, X., Liu, Y., Sun, C., Li, M.,
Wang, S., and Chen, X. (2021). Conditional adversar-
ial domain generalization with a single discriminator
for bearing fault diagnosis. IEEE Transactions on In-
strumentation and Measurement, 70:1–15.
Zhao, C. and Shen, W. (2022). A domain generalization
network combing invariance and specificity towards
real-time intelligent fault diagnosis. Mechanical Sys-
tems and Signal Processing, 173:108990.
Zhao, Z., Li, T., Wu, J., Sun, C., Wang, S., Yan, R., and
Chen, X. (2020). Deep learning algorithms for rotat-
ing machinery intelligent diagnosis: An open source
benchmark study. ISA Transactions.
Zhao, Z., Zhang, Q., Yu, X., Sun, C., Wang, S., Yan, R.,
and Chen, X. (2021). Applications of unsupervised
deep transfer learning to intelligent fault diagnosis: A
survey and comparative study. IEEE Transactions on
Instrumentation and Measurement, 70:1–28.
Zheng, H., Yang, Y., Yin, J., Li, Y., Wang, R., and Xu,
M. (2021). Deep domain generalization combining a
priori diagnosis knowledge toward cross-domain fault
diagnosis of rolling bearing. IEEE Transactions on
Instrumentation and Measurement, 70:1–11.
IoTBDS 2023 - 8th International Conference on Internet of Things, Big Data and Security
38