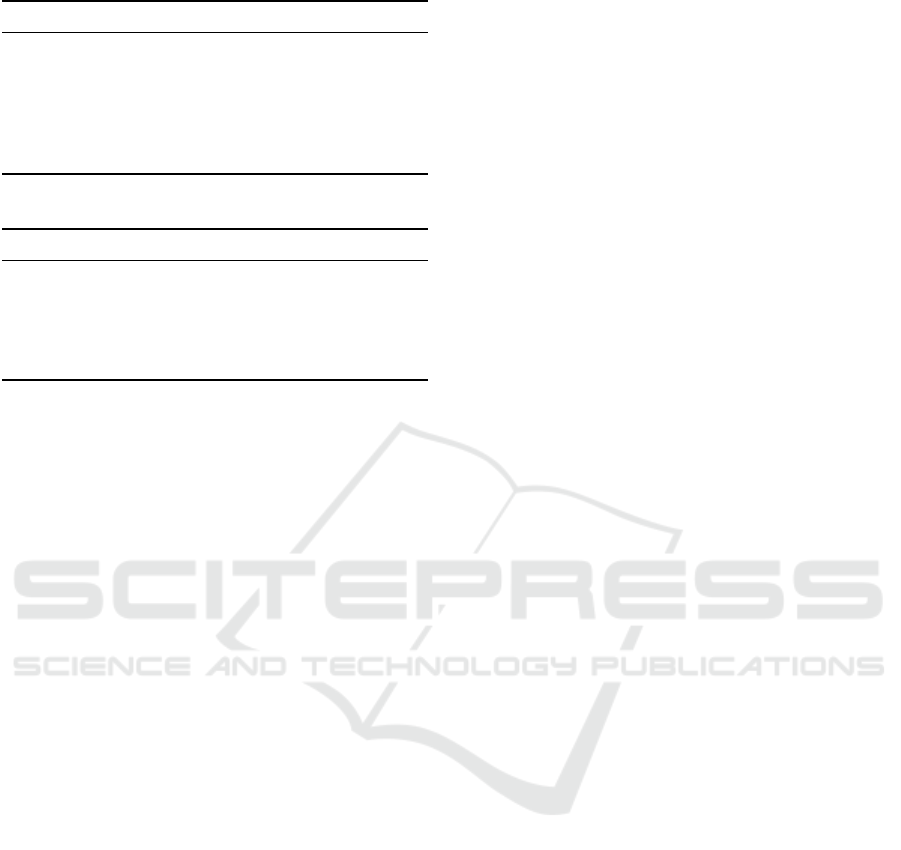
Table 4: Query results for Netflix data.
S. No. The Pacifier
1 Agent Cody Banks
2 Agent Cody Banks 2: Destination London
3 Lilo and Stitch 2
4 101 Dalmations II: Patch’s London
Adventure
5 Mean Creek
Table 5: Query results for Netflix data with Bi-LBLMA.
S. No. Resident Evil
1 Dawn of the Dead
2 Sasquatch
3 Wrong Turn
4 Evil Remains
5 Dead Birds
5 CONCLUSION
We have introduced two novel models for topic mod-
elling and applied it for recommendation tasks. The
models are found to be effective when compared to
widely used models such as LDA. From the exam-
ple queries, we see that our models are able to deliver
promising suggestions that the user might like. The
improvement achieved by using GD and BL distribu-
tions is also clearly seen. Using biterms in conjunc-
tion with our models tend to improve the results con-
siderably. Especially, the Bi-LBLMA model proves
to be a good alternative to LDA based on the results
from both the experiments.
REFERENCES
Attias, H. (1999). A variational baysian framework for
graphical models. In Solla, S., Leen, T., and M
¨
uller,
K., editors, Advances in Neural Information Process-
ing Systems, volume 12, Cambridge, Masschusetts.
MIT Press.
Bakhtiari, A. S. and Bouguila, N. (2014). Online learning
for two novel latent topic models. In Linawati, Ma-
hendra, M. S., Neuhold, E. J., Tjoa, A. M., and You, I.,
editors, Information and Communication Technology,
pages 286–295, Berlin, Heidelberg. Springer Berlin
Heidelberg.
Bakhtiari, A. S. and Bouguila, N. (2016). A latent beta-
liouville allocation model. Expert Systems with Appli-
cations, 45:260–272.
Blei, D. M., Ng, A. Y., and Jordan, M. I. (2003). Latent
dirichlet allocation. Journal of machine Learning re-
search, 3(Jan):993–1022.
Bobadilla, J., Hernando, A., Ortega, F., and Bernal, J.
(2011). A framework for collaborative filtering rec-
ommender systems. Expert Systems with Applica-
tions, 38(12):14609–14623.
Chien, J.-T., Lee, C.-H., and Tan, Z.-H. (2018). Latent
dirichlet mixture model. Neurocomputing, 278:12–
22. Recent Advances in Machine Learning for Non-
Gaussian Data Processing.
Chong, W., Blei, D., and Li, F.-F. (2009). Simultaneous im-
age classification and annotation. In 2009 IEEE Con-
ference on Computer Vision and Pattern Recognition,
pages 1903–1910.
Fan, W., Bouguila, N., and Ziou, D. (2012). Variational
learning for finite dirichlet mixture models and appli-
cations. IEEE transactions on neural networks and
learning systems, 23(5):762–774.
Hu, C., Fan, W., Du, J.-X., and Bouguila, N. (2019). A
novel statistical approach for clustering positive data
based on finite inverted beta-liouville mixture models.
Neurocomputing, 333:110–123.
Liu, Y., Du, F., Sun, J., and Jiang, Y. (2020). ilda: An
interactive latent dirichlet allocation model to im-
prove topic quality. Journal of Information Science,
46(1):23–40.
Maanicshah, K., Amayri, M., and Bouguila, N. (2022).
Improving topic quality with interactive beta-liouville
mixture allocation model. In 2022 IEEE Symposium
Series on Computational Intelligence (SSCI), pages
1143–1148.
Maanicshah, K., Amayri, M., and Bouguila, N. (2023).
Interactive generalized dirichlet mixture allocation
model. In Structural, Syntactic, and Statistical Pattern
Recognition: Joint IAPR International Workshops, S+
SSPR 2022, Montreal, QC, Canada, August 26–27,
2022, Proceedings, pages 33–42. Springer.
Mimno, D., Wallach, H. M., Naradowsky, J., Smith, D. A.,
and McCallum, A. (2009). Polylingual topic models.
In Proceedings of the 2009 Conference on Empirical
Methods in Natural Language Processing: Volume 2 -
Volume 2, EMNLP ’09, page 880–889, USA. Associ-
ation for Computational Linguistics.
Mimno, D., Wallach, H. M., Talley, E., Leenders, M., and
McCallum, A. (2011). Optimizing semantic coher-
ence in topic models. In Proceedings of the Confer-
ence on Empirical Methods in Natural Language Pro-
cessing, Proceedings of the Conference on Empirical
Methods in Natural Language Processing; EMNLP
’11, page 262–272, USA. Association for Computa-
tional Linguistics.
Nagori, R. and Aghila, G. (2011). Lda based integrated
document recommendation model for e-learning sys-
tems. In 2011 International Conference on Emerging
Trends in Networks and Computer Communications
(ETNCC), pages 230–233.
Opper, M. and Saad, D. (2001). Advanced mean field meth-
ods: Theory and practice. MIT press, Cambridge,
Masschusetts.
Pazzani, M. J. and Billsus, D. (2007). Content-Based
Recommendation Systems, pages 325–341. Springer
Berlin Heidelberg, Berlin, Heidelberg.
Novel Topic Models for Content Based Recommender Systems
145