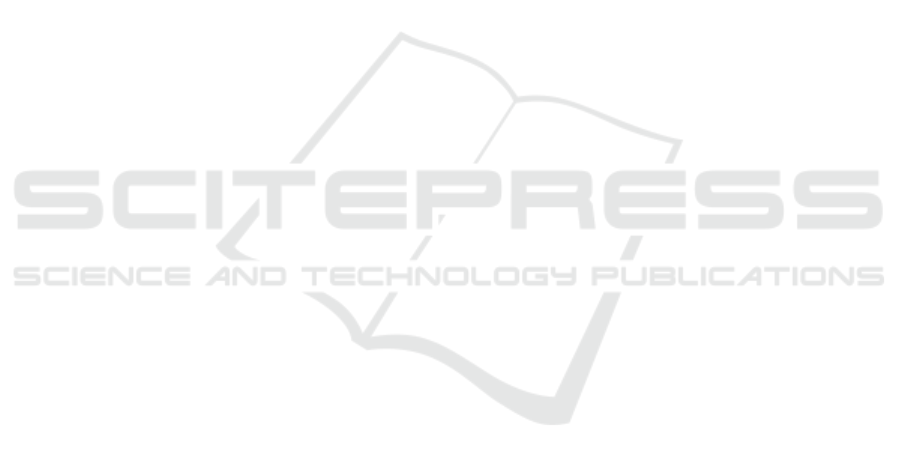
prove the accuracy of the fake news detector.
Moreover, we plan to evaluate the combination of
our framework with more sophisticated uncertainty
estimation methods, as well as to devise mechanisms
for differentiating true labelled data from pseudo-
labelled data in the self-training process, in order to
reduce the risk of confirmation bias that may arise
from computing traditional loss functions over pseudo
labels.
ACKNOWLEDGEMENTS
This work was partly supported by the Euro-
pean Commission funded project ”HumanE-AI-
Net” (grant no. 952026) and by project SER-
ICS (PE00000014) under the NRRP MUR program
funded by the EU - NGEU. Their support is gratefully
acknowledged.
REFERENCES
Benamira, A., Devillers, B., Lesot, E., Ray, A. K., Saadi,
M., and Malliaros, F. D. (2019). Semi-supervised
learning and graph neural networks for fake news de-
tection. In Spezzano, F., Chen, W., and Xiao, X.,
editors, ASONAM ’19: International Conference on
Advances in Social Networks Analysis and Mining,
Vancouver, British Columbia, Canada, 27-30 August,
2019, pages 568–569. ACM.
Cascante-Bonilla, P., Tan, F., Qi, Y., and Ordonez, V.
(2021). Curriculum labeling: Revisiting pseudo-
labeling for semi-supervised learning. In Proceedings
of the AAAI Conference on Artificial Intelligence, vol-
ume 35, pages 6912–6920.
Devlin, J., Chang, M. W., Lee, K., and Toutanova, K.
(2019). BERT: Pre-training of deep bidirectional
transformers for language understanding. In NAACL-
HLT, pages 4171–4186. Association for Computa-
tional Linguistics.
Dong, X., Victor, U., Chowdhury, S., and Qian, L. (2019).
Deep two-path semi-supervised learning for fake news
detection. CoRR, abs/1906.05659.
Guacho, G. B., Abdali, S., Shah, N., and Papalexakis, E. E.
(2020). Semi-supervised content-based detection of
misinformation via tensor embeddings. In Proceed-
ings of the 2018 IEEE/ACM International Conference
on Advances in Social Networks Analysis and Mining,
ASONAM ’18, page 322–325. IEEE Press.
Guarascio, M., Manco, G., and Ritacco, E. (2018). Deep
learning. Encyclopedia of Bioinformatics and Com-
putational Biology: ABC of Bioinformatics, 1-3:634–
647.
Laine, S. and Aila, T. (2016). Temporal ensem-
bling for semi-supervised learning. arXiv preprint
arXiv:1610.02242.
Lee, D.-H. (2013). Pseudo-label : The simple and efficient
semi-supervised learning method for deep neural net-
works. ICML 2013 Workshop : Challenges in Repre-
sentation Learning (WREPL).
Liu, C., Wu, X., Yu, M., Li, G., Jiang, J., Huang, W., and
Lu, X. (2019). A two-stage model based on bert for
short fake news detection. In Douligeris, C., Kara-
giannis, D., and Apostolou, D., editors, Knowledge
Science, Engineering and Management, pages 172–
183, Cham. Springer International Publishing.
Liu, Z., Lin, Y., and Sun, M. (2020). Representation learn-
ing for natural language processing. Springer Nature.
Meel, P. and Vishwakarma, D. K. (2021a). Fake news detec-
tion using semi-supervised graph convolutional net-
work. CoRR, abs/2109.13476.
Meel, P. and Vishwakarma, D. K. (2021b). A temporal en-
sembling based semi-supervised convnet for the de-
tection of fake news articles. Expert Systems with Ap-
plications, 177:115002.
Mena, J., Pujol, O., and Vitri
`
a, J. (2021). A survey on
uncertainty estimation in deep learning classification
systems from a bayesian perspective. ACM Comput.
Surv., 54(9).
Rout, J. K., Dalmia, A., Choo, K.-K. R., Bakshi, S., and
Jena, S. K. (2017). Revisiting semi-supervised learn-
ing for online deceptive review detection. IEEE Ac-
cess, 5:1319–1327.
Shu, K., Mahudeswaran, D., Wang, S., Lee, D., and Liu,
H. (2018). Fakenewsnet: A data repository with news
content, social context and dynamic information for
studying fake news on social media. arXiv preprint
arXiv:1809.01286.
Shu, K., Sliva, A., Wang, S., Tang, J., and Liu, H. (2017).
Fake news detection on social media: A data mining
perspective. ACM SIGKDD Explorations Newsletter,
19(1):22–36.
Zhou, X. and Zafarani, R. (2020). A survey of fake news:
Fundamental theories, detection methods, and oppor-
tunities. ACM Comput. Surv., 53(5).
Learning Deep Fake-News Detectors from Scarcely-Labelled News Corpora
353