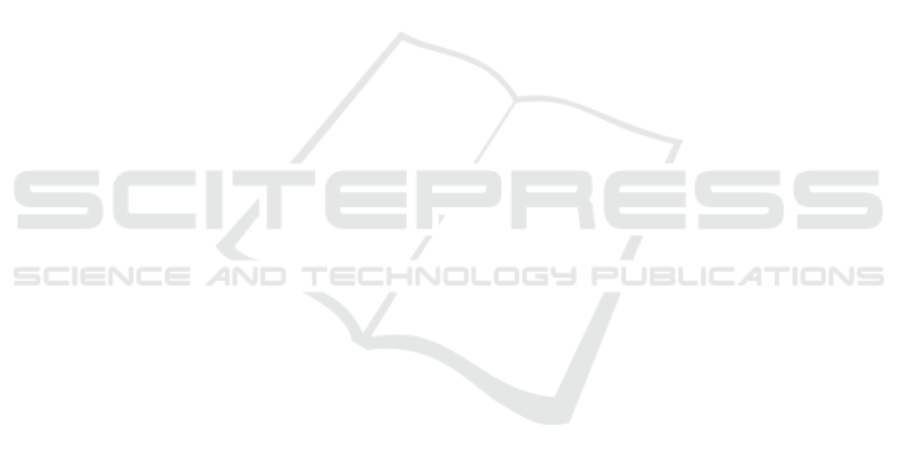
to localize accurately the transition between cheese
rind and paste.
Future work will be devoted to providing a more
comprehensive characterization of the rind thickness,
for instance, by measuring it in other parts of the
cheese wheel, like in the angles formed by the heel
and the upper and lower faces. We are furthermore
interested in investigating the estimation of the rind
thickness using images depicting rock-cracked cheese
slices rather than wire-cut. In this new scenario, the
hand-crafted algorithms have little chance of success
because there are even fewer visual changes in the
transition from the rind to the paste.
Finally, rind thickness is only one of the features
considered by the internal quality panel of Trentin-
grana Consortium. Automatic estimation of other vi-
sual characteristics, such as paste color and texture,
could be a topic for future research to provide more
comprehensive support to experts.
ACKNOWLEDGEMENTS
The research was funded by Trentingrana - Consorzio
dei Caseifici Sociali Trentini, Italy and by the Au-
tonomous Province of Trento, Italy (as part of the
ADP funding prot. n. 244380 dd 04/05/2020 and
the TRENTINGRANA project - RDP 201-202, CUP
C66D17000180008).
REFERENCES
Akikawa, M. and Yamamura, M. (2021). Materializing
Architecture for Processing Multimodal Signals for
a Humanoid Robot Control System. Journal of Ad-
vanced Computational Intelligence and Intelligent In-
formatics, 25(3):335–345.
Badaro, A., de Matos, G., Karaziack, C., et al. (2021). Au-
tomated Method for Determination of Cheese Melta-
bility by Computer Vision. Food Analytical Methods,
14:2630–2641.
Bosakova-Ardenska, A., Panayotov, P., Boyanova, P., and
Pashova, E. (2020). Application of Images Segmenta-
tion for Evaluation Structure of White Brined Cheese.
In International Conference on Information Technolo-
gies, pages 1–4.
Brosnan, T. and Sun, D.-W. (2004). Improving Quality In-
spection of Food Products by Computer Vision - A
Review. Journal of Food Engineering, 61(1):3–16.
Du, C.-J. and Sun, D.-W. (2004). Recent Developments in
the Applications of Image Processing Techniques for
Food Quality Evaluation. Trends in Food Science &
Technology, 15(5):230–249.
EyeQuestion (Accessed: Dec 2022). https://eyequestion.nl.
He, K., Zhang, X., Ren, S., and Sun, J. (2016). Deep Resid-
ual Learning for Image Recognition. In IEEE Con-
ference on Computer Vision and Pattern Recognition,
pages 770–778.
Jackman, P. and Sun, D.-W. (2013). Recent Advances in Im-
age Processing using Image Texture Features for Food
Quality Assessment. Trends in Food Science & Tech-
nology, 29(1):35–43.
Lei, T. and Sun, D.-W. (2019). Developments of Nonde-
structive Techniques for Evaluating Quality Attributes
of Cheeses: A review. Trends in Food Science & Tech-
nology, 88:527–542.
Liu, Z., Mao, H., Wu, C., et al. (2022). A ConvNet for the
2020s. In IEEE Conference on Computer Vision and
Pattern Recognition, pages 11966–11976.
Loshchilov, I. and Hutter, F. (2018). Decoupled Weight De-
cay Regularization. In International Conference on
Learning Representations.
Lukinac, J., Juki
´
c, M., Mastanjevi
´
c, K., and Lu
ˇ
can, M.
(2018). Application of Computer Vision and Image
Analysis Method in Cheese-Quality Evaluation: a Re-
view. Ukrainian Food Journal, 7(2):192–214.
Ma, J., Sun, D.-W., Qu, J.-H., et al. (2016). Applications
of Computer Vision for Assessing Quality of Agri-
Food Products: A Review of Recent Research Ad-
vances. Critical Reviews in Food Science and Nutri-
tion, 56(1):113–127.
Radford, A., Kim, J. W., Hallacy, C., et al. (2021). Learn-
ing transferable visual models from natural language
supervision. In International Conference on Machine
Learning, pages 8748–8763.
Radosavovic, I., Kosaraju, R. P., Girshick, R., et al. (2020).
Designing network design spaces. In 2020 IEEE/CVF
Conference on Computer Vision and Pattern Recogni-
tion (CVPR), pages 10425–10433.
Russ, J. C. (2015). Image analysis of foods. Journal of Food
Science, 80(9):E1974–E1987.
Russakovsky, O., Deng, J., Su, H., et al. (2015). Imagenet
large scale visual recognition challenge. International
journal of computer vision, 115(3):211–252.
Sun, D.-W. (2016). Computer Vision Technology for Food
Quality Evaluation. Academic Press.
Turgut, S. S., Karacabey, E., K
¨
uc¸
¨
uk
¨
oner, E., et al. (2014).
Potential of image analysis based systems in food
quality assessments and classifications. In 9th Baltic
Conference on Food Science and Technology, pages
8–12.
Zhang, X., Zhou, X., Lin, M., and Sun, J. (2018). Shuf-
fleNet: An extremely efficient Convolutional Neural
Network for mobile devices. In IEEE Conference
on Computer Vision and Pattern Recognition, pages
6848–6856.
A Deep Learning Approach for Estimating the Rind Thickness of Trentingrana Cheese from Images
83