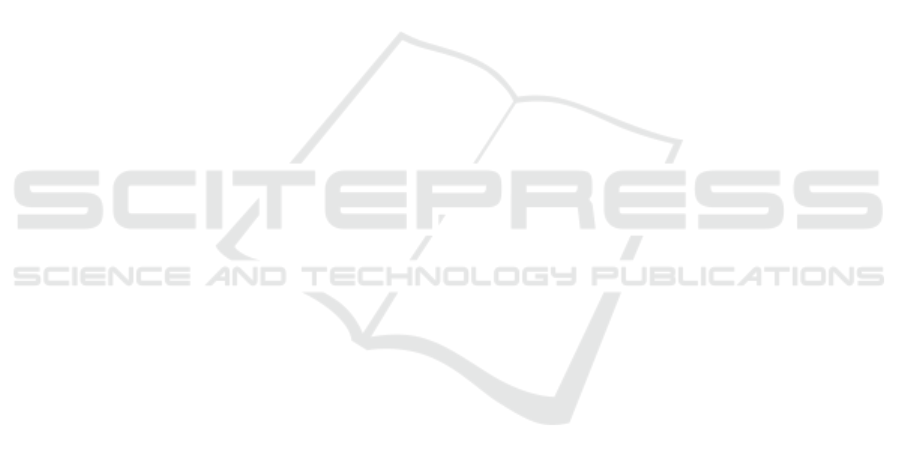
6 FUTURE WORK AND
RESEARCH CHALLENGES
In this work, we presented SAFR-AV, a platform
for end-to-end Simulation-in-the-loop (SIL) testing
of AV stacks using real-world data for the purpose
of pre-certification wrt various behavior competen-
cies. We presented results on extraction of real-world
scenes relevant for a behavioral competency under
test, conversion of these scenes into digital twin rep-
resentations and generation of real-world distribution
of scenario parameters for optimizing test coverage.
Future work would involve development of coverage
optimization and smart sampling engines for ensuring
exposure of statistical variability of the test scenario
and to generate edge/critical cases. The research chal-
lenges include:
1. Robustness and accuracy of the multi-sensor per-
ception algorithms used to extract relevant scenar-
ios from real world data.
2. Robust map-matching to position the objects cor-
rectly.
3. Generating multi-variate heterogeneous probabil-
ity distributions of sets of scenario parameters.
4. Causality Analysis in the safety assessment mod-
ule to identify failure points and modes for the AV
stack.
REFERENCES
ASAM (2017). ASAM OpenDrive 1.7.0 User Guide.
ASAM Standard ASAM OpenDRIVE 1.7.0, Associa-
tion for Standardization of Automation and Measuring
Systems (ASAM). [Online; accessed 15-February-
2023].
ASAM (2018). ASAM OpenSCENARIO 1.1 User guide.
ASAM Standard ASAM SCENARIO 1.1, Associa-
tion for Standardization of Automation and Measuring
Systems (ASAM). [Online; accessed 15-February-
2023].
ASAM (2022). ASAM OpenSCENARIO 2.0 Public release
candidate. ASAM Standard ASAM SCENARIO 2.0,
Association for Standardization of Automation and
Measuring Systems (ASAM). [Online; accessed 15-
February-2023].
Bagschik, G., Menzel, T., and Maurer, M. (2018). Ontol-
ogy based scene creation for the development of au-
tomated vehicles. In 2018 IEEE Intelligent Vehicles
Symposium (IV), pages 1813–1820.
Clarke, E. M., Klieber, W., Nov
´
a
ˇ
cek, M., and Zuliani, P.
(2011). Model checking and the state explosion prob-
lem. In LASER Summer School on Software Engineer-
ing, pages 1–30, Berlin, Heidelberg. Springer.
Fremont, D. J., Kim, E., Pant, Y. V., Seshia, S. A., Acharya,
A., Bruso, X., and Mehta, S. (2020). Formal scenario-
based testing of autonomous vehicles: From simula-
tion to the real world. In 2020 IEEE 23rd Interna-
tional Conference on Intelligent Transportation Sys-
tems (ITSC), pages 1–8.
Geiger, A., Lenz, P., Stiller, C., and Urtasun, R. (2013). Vi-
sion meets Robotics: The KITTI Dataset. In Interna-
tional Journal of Robotics Research, volume 32, pages
1231–1237. [Online; accessed 15-February-2023].
Kalra, N. and Paddock, S. M. (2016). Driving to safety:
How many miles of driving would it take to demon-
strate autonomous vehicle reliability?
Karunakaran, D., Berrio, J. S., Worrall, S., and Nebot,
E. (2022). Automatic lane change scenario extrac-
tion and generation of scenarios in openx format from
real-world data. Robotics and Autonomous Systems,
148:103816.
Knull, J. E. (2017). Turn detection and analysis of turn pa-
rameters for driver characterization.
Medrano-Berumen, C. and Akbas¸, M. I. (2019). Abstract
simulation scenario generation for autonomous vehi-
cle verification. In 2019 SoutheastCon, pages 1–6.
National Highway Traffic Safety Administration (NHTSA)
(2018). A framework for automated driving system
testable cases and scenarios.
Park, J., Wen, M., Sung, Y., and Cho, K. (2019). Multiple
event-based simulation scenario generation approach
for autonomous vehicle smart sensors and devices. In
2019 IEEE Intelligent Transportation Systems Confer-
ence (ITSC), pages 1003–1008.
Riedmaier, S., Ponn, T., Ludwig, D., Schick, B., and Dier-
meyer, F. (2020). Survey on scenario-based safety
assessment of automated vehicles. IEEE Access,
8:87456–87477.
Tenbrock, A., K
¨
onig, A., Keutgens, T., Bock, J., Weber, H.,
Krajewski, R., and Zlocki, A. (2021). The conscend
dataset: Concrete scenarios from the highd dataset ac-
cording to alks regulation unece r157 in openx.
Wei, F., Guo, W., Liu, X., Liang, C., and Feng, T. (2014).
Left-turning vehicle trajectory modeling and guide
line setting at the intersection. Discrete Dynamics in
Nature and Society.
Xiao, P., Shao, Z., Hao, S., Zhang, Z., Chai, X., Jiao,
J., Li, Z., Wu, J., Sun, K., Jiang, K., Wang, Y.,
and Yang, D. (2021). PandaSet: Advanced Sensor
Suite Dataset for Autonomous Driving. arXiv preprint
arXiv:2112.06058. [Online; accessed 15-February-
2023].
SAFR-AV: Safety Analysis of Autonomous Vehicles Using Real World Data: An End-to-End Solution for Real World Data Driven
Scenario-Based Testing for Pre-Certification of AV Stacks
239