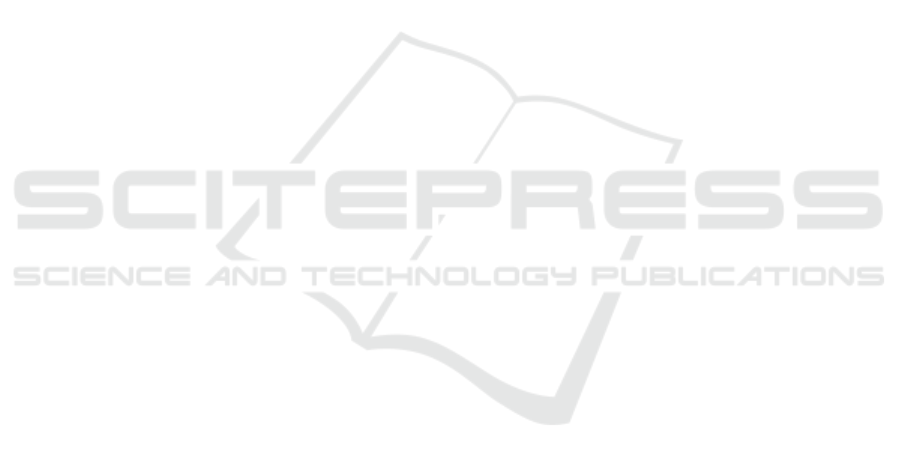
2019 Competition on Table Detection and Recogni-
tion (cTDaR). pages 1510–1515.
Gao, L., Yi, X., Jiang, Z., Hao, L., and Tang, Z. (2017). IC-
DAR2017 Competition on Page Object Detection. In
2017 14th IAPR International Conference on Docu-
ment Analysis and Recognition (ICDAR), pages 1417–
1422, Kyoto. IEEE.
Gilani, A., Qasim, S. R., Malik, I., and Shafait, F. (2017).
Table Detection Using Deep Learning. In 2017 14th
IAPR International Conference on Document Analy-
sis and Recognition (ICDAR), pages 771–776, Kyoto.
IEEE.
Girshick, R. (2015). Fast R-CNN. Technical Report
arXiv:1504.08083, arXiv. arXiv:1504.08083 [cs].
Gobel, M., Hassan, T., Oro, E., and Orsi, G. (2013). ICDAR
2013 Table Competition. In 2013 12th International
Conference on Document Analysis and Recognition,
pages 1449–1453, Washington, DC, USA. IEEE.
Hashmi, K. A., Liwicki, M., Stricker, D., Afzal, M. A.,
Afzal, M. A., and Afzal, M. Z. (2021). Current Sta-
tus and Performance Analysis of Table Recognition
in Document Images with Deep Neural Networks.
arXiv:2104.14272 [cs]. arXiv: 2104.14272.
He, K., Gkioxari, G., Dollár, P., and Girshick, R. (2018).
Mask R-CNN. arXiv:1703.06870 [cs].
Krizhevsky, A., Sutskever, I., and Hinton, G. E. (2012).
ImageNet classification with deep convolutional neu-
ral networks. In Pereira, F., Burges, C., Bottou, L.,
and Weinberger, K., editors, Advances in neural in-
formation processing systems, volume 25. Curran As-
sociates, Inc.
Li, M., Cui, L., Huang, S., Wei, F., Zhou, M., and
Li, Z. (2019). TableBank: Table Benchmark
for Image-based Table Detection and Recognition.
arXiv:1903.01949 [cs]. arXiv: 1903.01949.
Lin, T.-Y., Dollár, P., Girshick, R., He, K., Hariharan, B.,
and Belongie, S. (2017). Feature Pyramid Networks
for Object Detection. arXiv:1612.03144 [cs].
Lin, T.-Y., Maire, M., Belongie, S., Bourdev, L., Girshick,
R., Hays, J., Perona, P., Ramanan, D., Zitnick, C. L.,
and Dollár, P. (2015). Microsoft COCO: Common Ob-
jects in Context. arXiv:1405.0312 [cs].
Prasad, D., Gadpal, A., Kapadni, K., Visave, M., and Sul-
tanpure, K. (2020). CascadeTabNet: An approach for
end to end table detection and structure recognition
from image-based documents. In 2020 IEEE/CVF
Conference on Computer Vision and Pattern Recogni-
tion Workshops (CVPRW), pages 2439–2447, Seattle,
WA, USA. IEEE.
Ren, S., He, K., Girshick, R., and Sun, J. (2016).
Faster R-CNN: Towards Real-Time Object Detection
with Region Proposal Networks. Technical Report
arXiv:1506.01497, arXiv. arXiv:1506.01497 [cs].
Rezatofighi, H., Tsoi, N., Gwak, J., Sadeghian, A., Reid,
I., and Savarese, S. (2019). Generalized Intersection
over Union: A Metric and A Loss for Bounding Box
Regression. arXiv:1902.09630 [cs].
Shafait, F. and Smith, R. (2010). Table detection in hetero-
geneous documents. In Proceedings of the 8th IAPR
International Workshop on Document Analysis Sys-
tems - DAS ’10, pages 65–72, Boston, Massachusetts.
ACM Press.
Siddiqui, S. A., Malik, M. I., Agne, S., Dengel, A., and
Ahmed, S. (2018). DeCNT: Deep Deformable CNN
for Table Detection. IEEE Access, 6:74151–74161.
Sildatke, M., Karwanni, H., Kraft, B., and Zündorf,
A. (2022a). ARTIFACT: Architecture for Auto-
mated Generation of Distributed Information Extrac-
tion Pipelines. In Filipe, J., Smialek, M., Brodsky, A.,
and Hammoudi, S., editors, Proceedings of the 24th
International Conference on Enterprise Information
Systems, ICEIS 2022, Online Streaming, April 25-27,
2022, Volume 2, pages 17–28. SCITEPRESS.
Sildatke, M., Karwanni, H., Kraft, B., and Zündorf, A.
(2022b). FUSION: Feature-based Processing of Het-
erogeneous Documents for Automated Information
Extraction. In Fill, H.-G., Sinderen, M. v., and Maci-
aszek, L. A., editors, Proceedings of the 17th Interna-
tional Conference on Software Technologies, ICSOFT
2022, Lisbon, Portugal, July 11-13, 2022, pages 250–
260. SCITEPRESS.
Solovyev, R., Wang, W., and Gabruseva, T. (2021).
Weighted boxes fusion: Ensembling boxes from dif-
ferent object detection models. Image and Vision
Computing, 107:104117. arXiv:1910.13302 [cs].
Taghva, K., Nartker, T., Borsack, J., and Condit, A. (2000).
Unlv-isri document collection for research in ocr and
information retrieval. 3967.
Torrey, L. and Shavlik, J. (2009). Transfer learning. Hand-
book of Research on Machine Learning Applications.
Viera, A. J. and Garrett, J. M. (2005). Understanding In-
terobserver Agreement: The Kappa Statistic. Family
Medicine, page 4.
Wu, Y., Kirillov, A., Massa, F., Lo, W.-Y., and Gir-
shick, R. (2019). Detectron2. https://github.com/
facebookresearch/detectron2.
Zeiler, M. D. and Fergus, R. (2013). Visualizing and Under-
standing Convolutional Networks. Technical Report
arXiv:1311.2901, arXiv. arXiv:1311.2901 [cs].
Zhang, C. and Ma, Y. (2012). Ensemble Machine Learn-
ing - Methods and Applications. Springer Science &
Business Media, Berlin Heidelberg.
Zhong, X., ShafieiBavani, E., and Yepes, A. J. (2020).
Image-based table recognition: data, model, and eval-
uation. Technical Report arXiv:1911.10683, arXiv.
arXiv:1911.10683 [cs].
Zhou, H., Li, Z., Ning, C., and Tang, J. (2017). CAD: Scale
Invariant Framework for Real-Time Object Detection.
In 2017 IEEE International Conference on Computer
Vision Workshops (ICCVW), pages 760–768, Venice.
IEEE.
Zou, Z., Shi, Z., Guo, Y., and Ye, J. (2019). Object Detec-
tion in 20 Years: A Survey. arXiv:1905.05055 [cs].
IMPROVE 2023 - 3rd International Conference on Image Processing and Vision Engineering
36