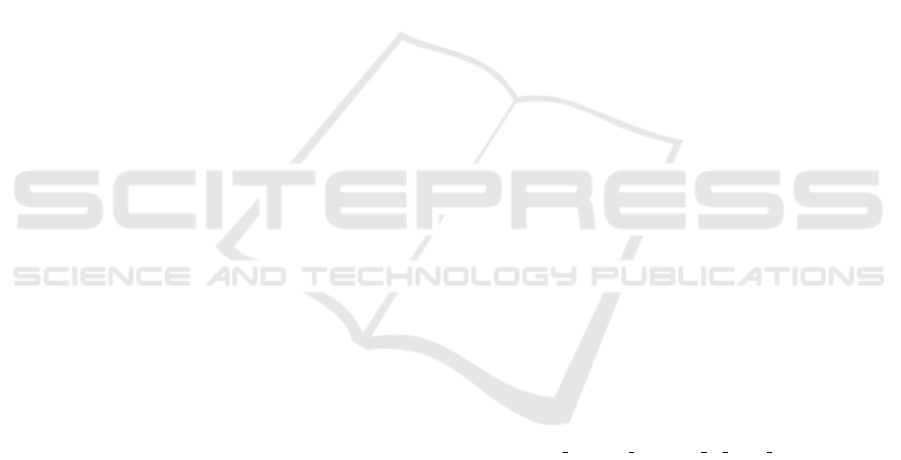
two sensors most often combined together in MSF.
We have discussed these and their countermeasures in
depth, before reviewing them in the context of MSF.
We have opened a discussion into attack complex-
ity and suggested a framework to review them in to
better grasp the challenging factors of this issue. It
is clear that more countermeasures are needed, how-
ever advancements in MSF are looking very promis-
ing, and some of the state-of-the-art solutions will be
a huge leap in making attacks too complex to be fea-
sible. It is still important to keep in mind that since
everyone, including heads-of-state, will be driving
around in these cars, complexity alone cannot be seen
as a sufficient. Solid standards need to be adopted.
REFERENCES
Burke, K. (2019). How Does a Self-Driving Car
See? https://blogs.nvidia.com/blog/2019/04/15/how-
does-a-self-driving-car-see/, Accessed 2023-01-19.
Cameron, O. (2017). An Introduction to LIDAR: The Key
Self-Driving Car Sensor — by Oliver Cameron —
Voyage. Voyage.
Cao, Y., Wang, N., Xiao, C., Yang, D., Fang, J., Yang, R.,
Chen, Q. A., Liu, M., and Li, B. (2021). Invisible
for both Camera and LiDAR: Security of multi-sensor
fusion based perception in autonomous driving under
physical-world attacks. In Proc. - IEEE Symp. Secur.
Priv., volume 2021-May, pages 176–194.
Cao, Y., Zhou, Y., Chen, Q. A., Xiao, C., Park, W., Fu, K.,
Cyr, B., Rampazzi, S., and Morley Mao, Z. (2019).
Adversarial sensor attack on LiDAR-based perception
in autonomous driving. In Proc. ACM Conf. Comput.
Commun. Secur., pages 2267–2281.
Deng, Y., Zhang, T., Lou, G., Zheng, X., Jin, J., and Han,
Q. L. (2021). Deep Learning-Based Autonomous
Driving Systems: A Survey of Attacks and Defenses.
IEEE Trans. Ind. Informatics.
Eykholt, K., Evtimov, I., Fernandes, E., Li, B., Rahmati,
A., Tram
`
er, F., Prakash, A., Kohno, T., and Song,
D. (2018a). Physical adversarial examples for object
detectors. In 12th USENIX Work. Offensive Technol.
WOOT 2018, co-located with USENIX Secur. 2018.
Eykholt, K., Evtimov, I., Fernandes, E., Li, B., Rahmati,
A., Xiao, C., Prakash, A., Kohno, T., and Song, D.
(2018b). Robust Physical-World Attacks on Deep
Learning Visual Classification. In Proc. IEEE Com-
put. Soc. Conf. Comput. Vis. Pattern Recognit., pages
1625–1634.
Jahromi, B. S., Tulabandhula, T., and Cetin, S. (2019).
Real-time hybrid multi-sensor fusion framework for
perception in autonomous vehicles. Sensors (Switzer-
land), 19(20).
Jones, D. (2022). California Approves A Pi-
lot Program For Driverless Rides : NPR.
https://www.npr.org/2021/06/05/1003623528/
california-approves-pilot-program-for-driverless-
rides, Accessed 2023-01-10.
Kocic, J., Jovicic, N., and Drndarevic, V. (2018). Sensors
and Sensor Fusion in Autonomous Vehicles. In 2018
26th Telecommun. Forum, TELFOR 2018 - Proc.
Liu, J. and Park, J. (2021). ”Seeing is not Always Believ-
ing”: Detecting Perception Error Attacks Against Au-
tonomous Vehicles. IEEE Trans. Dependable Secur.
Comput.
Matsumura, R., Sugawara, T., and Sakiyama, K. (2018). A
secure LiDAR with AES-based side-channel finger-
printing. In Proc. - 2018 6th Int. Symp. Comput. Netw.
Work. CANDARW 2018, pages 479–482.
Neal, A. (2018). LiDAR vs. RADAR.
https://www.fierceelectronics.com/ components/lidar-
vs-radar, Accessed 2023-01-19.
Petit, F. (2022). What are the 5 levels of autonomous
driving? - Blickfeld. https://www.blickfeld.com/
blog/levels-of-autonomous-driving/, Accessed 2023-
01-14.
Petit, J., Stottelaar, B., Feiri, M., and Kargl, F. (2015). Re-
mote Attacks on Automated Vehicles Sensors: Exper-
iments on Camera and LiDAR. Blackhat.com, pages
1–13.
Qayyum, A., Usama, M., Qadir, J., and Al-Fuqaha, A.
(2020). Securing Connected Autonomous Vehicles:
Challenges Posed by Adversarial Machine Learning
and the Way Forward. IEEE Commun. Surv. Tutori-
als, 22(2):998–1026.
Roriz, R., Cabral, J., and Gomes, T. (2022). Automotive
LiDAR Technology: A Survey. IEEE Trans. Intell.
Transp. Syst., 23(7):6282–6297.
Shin, H., Kim, D., Kwon, Y., and Kim, Y. (2017). Illu-
sion and dazzle: Adversarial optical channel exploits
against lidars for automotive applications. In Lect.
Notes Comput. Sci. (including Subser. Lect. Notes Ar-
tif. Intell. Lect. Notes Bioinformatics), volume 10529
LNCS, pages 445–467.
Tencent Keen Security Lab (2019). Experimental Se-
curity Research of Tesla Autopilot. page 38.
https://keenlab.tencent.com/en/whitepapers/Experi-
mental
Security Research of Tesla Autopilot.pdf,
Accessed 2023-01-13.
Xu, D., Anguelov, D., and Jain, A. (2018). PointFusion:
Deep Sensor Fusion for 3D Bounding Box Estima-
tion. In Proc. IEEE Comput. Soc. Conf. Comput. Vis.
Pattern Recognit., pages 244–253.
Zhou, H., Li, W., Kong, Z., Guo, J., Zhang, Y., Yu, B.,
Zhang, L., and Liu, C. (2020). Deepbillboard: Sys-
tematic physical-world testing of autonomous driving
systems. In Proc. - Int. Conf. Softw. Eng., pages 347–
358.
Analysis of Sensor Attacks Against Autonomous Vehicles
139