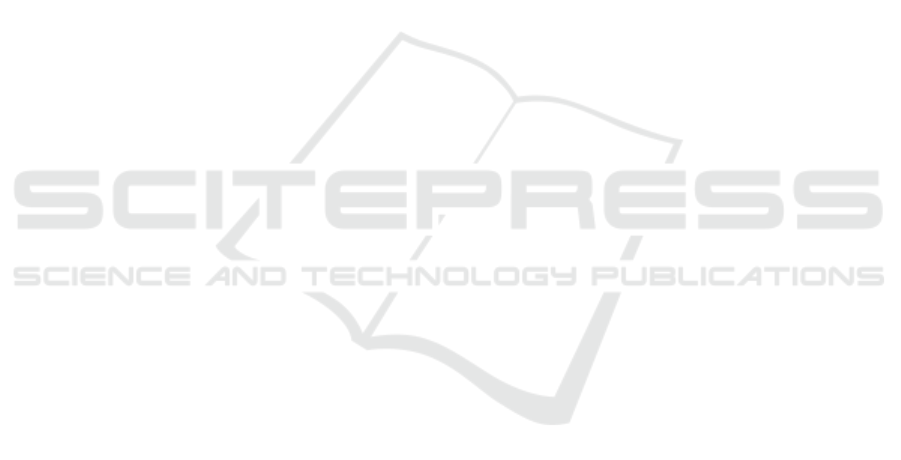
pleteness. IEEE Trans. Serv. Comput., 15(4):2086–
2099.
Liu, N., Huang, J., and Cui, L. (2018). A framework
for online process concept drift detection from event
streams. In IEEE Int’l Conf. Serv. Comput., pages
105–112.
Lu, J., Liu, A., Dong, F., Gu, F., Gama, J., and Zhang, G.
(2019). Learning under concept drift: A review. IEEE
Trans. on Knowledge and Data Eng., 31(12):2346–
2363.
Lu, Y., Chen, Q., and Poon, S. (2021a). Detecting and un-
derstanding branching frequency changes in process
models. In Enterprise, Business-Proc. and Inf. Syst.
Modeling, pages 39–46.
Lu, Y., Chen, Q., and Poon, S. (2021b). A robust and
accurate approach to detect process drifts from event
streams. In Bus. Process Manage., pages 383–399.
Luengo, D. and Sep
´
ulveda, M. (2012). Applying clustering
in process mining to find different versions of a busi-
ness process that changes over time. In 7th Int’l Wks.
on Bus. Proc. Intel., pages 153–158.
Maaradji, A., Dumas, M., La Rosa, M., and Ostovar, A.
(2015). Fast and accurate business process drift detec-
tion. In Bus. Process Manage., pages 406–422.
Maaradji, A., Dumas, M., Rosa, M., and Ostovar, A. (2017).
Detecting sudden and gradual drifts in business pro-
cesses from execution traces. IEEE Trans. on Knowl.
and Data Engin., 29(10):2140–2154.
Manoj Kumar, M., Thomas, L., and Annappa, B. (2015).
Capturing the sudden concept drift in process mining.
In Int’l Works. on Algor. & Theories for the Anal. of
Event Data, pages 132–143.
Martjushev, J., Jagadeesh Chandra Bose, R., and van der
Aalst, W. (2015). Change point detection and deal-
ing with gradual and multi-order dynamics in process
mining. In Perspectives in Bus. Inform. Research,
pages 161–178.
McDonald, J. and of Delaware, U. (2009). Handbook of
Biological Statistics. Sparky House Publishing, 2 edi-
tion.
Ostovar, A., Leemans, S., and Rosa, M. (2020). Robust
drift characterization from event streams of business
processes. ACM Trans. Knowl. Discov. Data, 14(3).
Ostovar, A., Maaradji, A., La Rosa, M., and Ter Hofstede,
A. (2017). Characterizing drift from event streams of
business processes. In Adv. Inf. Syst. Eng., pages 210–
228.
Ostovar, A., Maaradji, A., La Rosa, M., ter Hofstede, A.,
and van Dongen, B. (2016). Detecting drift from event
streams of unpredictable business processes. In 35th
Int’l Conf. on Conceptual Modeling, pages 330–346.
Pauwels, S. and Calders, T. (2019). An anomaly detection
technique for business processes based on extended
dynamic bayesian networks. In ACM/SIGAPP Symp.
Appl. Computing, page 494–501.
Richter, F. and Seidl, T. (2017). Tesseract: Time-drifts in
event streams using series of evolving rolling averages
of completion times. In Bus. Process Manage., pages
289–305.
Sato, D. M. V., De Freitas, S. C., Barddal, J. P., and Scal-
abrin, E. E. (2021). A survey on concept drift in pro-
cess mining. ACM Comput. Surv., 54(9).
Seeliger, A., Nolle, T., and M
¨
uhlh
¨
auser, M. (2017). De-
tecting concept drift in processes using graph metrics
on process graphs. In Conf. on Subject-Oriented Bus.
Process Manage.
Song, M., G
¨
unther, C. W., and van der Aalst, W. (2009).
Trace clustering in process mining. In Int’l Wks. on
Bus. Proc. Intel., pages 109–120.
Stertz, F. and Rinderle-Ma, S. (2018). Process histories - de-
tecting and representing concept drifts based on event
streams. In Int’l Conf. on Coop. Inform. Sys., pages
318–335.
Tavares, G., Ceravolo, P., Da Costa, V., Damiani, E., and Ju-
nior, S. (2019). Overlapping analytic stages in online
process mining. In IEEE Int’l Conf. Serv. Comput.,
pages 167–175.
Truong, C., Oudre, L., and Vayatis, N. (2020). Selective re-
view of offline change point detection methods. Signal
Processing, 167.
van der Aalst, W. (2014). Process mining in the large: A
tutorial. In 3rd Eur. Summer Sch. on Bus. Intell., pages
33–76.
van der Aalst, W. (2016). Process Mining: Data Science in
Action. Springer Berlin, Heidelberg, 2 edition.
van der Aalst, W. and et al. (2012). Process mining man-
ifesto. In 7th Int’l Wks. on Bus. Proc. Intel., pages
169–194.
Weber, P., Bordbar, B., and Ti
ˇ
no, P. (2011). Real-time de-
tection of process change using process mining. In
Imp. Coll. Comput. Stud. Wks, pages 108–114.
Yeshchenko, A., Ciccio, C., Mendling, J., and Polyvyanyy,
A. (2019a). Comprehensive process drift analysis with
the visual drift detection tool. In 38th Int’l Conf. on
Conceptual Modeling, pages 108–112.
Yeshchenko, A., Di Ciccio, C., Mendling, J., and
Polyvyanyy, A. (2019b). Comprehensive process drift
detection with visual analytics. In 38th Int’l Conf. on
Conceptual Modeling, pages 119–135.
Yeshchenko, A., Di Ciccio, C., Mendling, J., and
Polyvyanyy, A. (2021). Visual drift detection for event
sequence data of business processes. IEEE Trans. Vi-
sual Comput. Graphics, 28(8):3050–3068.
Yeshchenko, A., Mendling, J., Di Ciccio, C., and
Polyvyanyy, A. (2020). VDD: A visual drift detection
system for process mining. In ICPM Doc. Consort.
and Tool Demo. Track, pages 31–34.
Zheng, C., Wen, L., and Wang, J. (2017). Detecting process
concept drifts from event logs. In Int’l Conf. on Coop.
Inform. Sys., pages 524–542.
ICEIS 2023 - 25th International Conference on Enterprise Information Systems
372