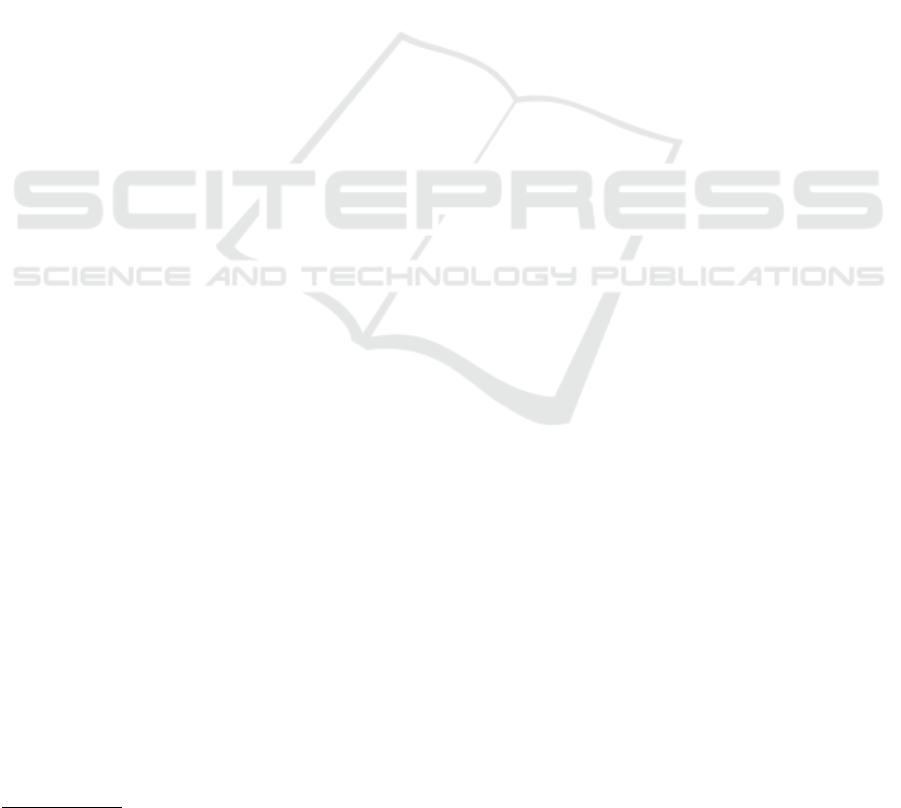
to meet the ESG data collection needs of banks and
other players in the European financial service arena.
ACKNOWLEDGEMENTS
This paper has been supported by the following
projects:
• “ESG - Alternative data in credit management” re-
alized in the context of the first call for project of
the Fintech Milano Hub
1
;
• “Validated Question Answering” n.
F/190114/01/X44 - CUP: B28I20000040005
PON “I&C” 2014-2020 FESR - And for sustain-
able growth - Sustainable manufacturing DM
05.03.2018 - DD 20/11/2018, art. 38, 47 e 48
D.P.R. n. 445 of 28/12/2000.
REFERENCES
Ackerman, S., Farchi, E., Raz, O., Zalmanovici, M., and
Dube, P. (2020). Detection of data drift and outliers
affecting machine learning model performance over
time.
Amati, G.
de Franco, C., Geissler, C., Margot, V., and Monnier, B.
(2020). Esg investments: Filtering versus machine
learning approaches.
Devlin, J., Chang, M., Lee, K., and Toutanova, K. (2018).
BERT: pre-training of deep bidirectional transformers
for language understanding. CoRR, abs/1810.04805.
Do
ˇ
silovi
´
c, F. K., Br
ˇ
ci
´
c, M., and Hlupi
´
c, N. (2018). Explain-
able artificial intelligence: A survey. In 2018 41st In-
ternational Convention on Information and Communi-
cation Technology, Electronics and Microelectronics
(MIPRO), pages 0210–0215.
Gupta, A., Sharma, U., and Gupta, S. K. (2021). The role of
esg in sustainable development: An analysis through
the lens of machine learning. In 2021 IEEE Interna-
tional Humanitarian Technology Conference (IHTC),
pages 1–5.
Hughes, A., Urban, M. A., and W
´
ojcik, D. (2021). Alterna-
tive esg ratings: How technological innovation is re-
shaping sustainable investment. Sustainability, 13(6).
Karpukhin, V., Oguz, B., Min, S., Wu, L., Edunov, S.,
Chen, D., and Yih, W. (2020). Dense passage re-
trieval for open-domain question answering. CoRR,
abs/2004.04906.
Kim, S.-W. and Gil, J.-M. (2019). Research paper clas-
sification systems based on tf-idf and lda schemes.
Human-centric Computing and Information Sciences,
9(1):30.
1
https://www.bancaditalia.it/focus/milano-hub/
Lee, O., Joo, H., Choi, H., and Cheon, M. (2022). Propos-
ing an integrated approach to analyzing esg data via
machine learning and deep learning algorithms. Sus-
tainability, 14(14).
Macpherson, M., Gasperini, A., and Bosco, M. (2021). Ar-
tificial intelligence and fintech technologies for esg
data and analysis (february 15, 2021).
Mikolov, T., Chen, K., Corrado, G., and Dean, J. (2013).
Efficient estimation of word representations in vector
space.
Nguyen, Q.-D., Le, D.-A., Phan, N.-M., and Zelinka, I.
(2021). Ocr error correction using correction pat-
terns and self-organizing migrating algorithm. Pattern
Analysis and Applications, 24(2):701–721.
Ni, J., Zhu, C., Chen, W., and McAuley, J. (2018). Learning
to attend on essential terms: An enhanced retriever-
reader model for open-domain question answering.
Pennington, J., Socher, R., and Manning, C. (2014). GloVe:
Global vectors for word representation. In Proceed-
ings of the 2014 Conference on Empirical Methods in
Natural Language Processing (EMNLP), pages 1532–
1543, Doha, Qatar. Association for Computational
Linguistics.
Rony, M. R. A. H., Zuo, Y., Kovriguina, L., Teucher, R., and
Lehmann, J. (2022). Climate bot: A machine read-
ing comprehension system for climate change ques-
tion answering. IJCAI.
Schultz, M. and Tropmann-Frick, M. (2020). Autoencoder
neural networks versus external auditors: Detecting
unusual journal entries in financial statement audits.
Sheth, A. P. and Thirunarayan, K. (2021). The in-
escapable duality of data and knowledge. CoRR,
abs/2103.13520.
Vaswani, A., Shazeer, N., Parmar, N., Uszkoreit, J.,
Jones, L., Gomez, A. N., Kaiser, L., and Polo-
sukhin, I. (2017). Attention is all you need. CoRR,
abs/1706.03762.
Wu, X., Xiao, L., Sun, Y., Zhang, J., Ma, T., and He, L.
(2021). A survey of human-in-the-loop for machine
learning. CoRR, abs/2108.00941.
ESG Data Collection with Adaptive AI
475