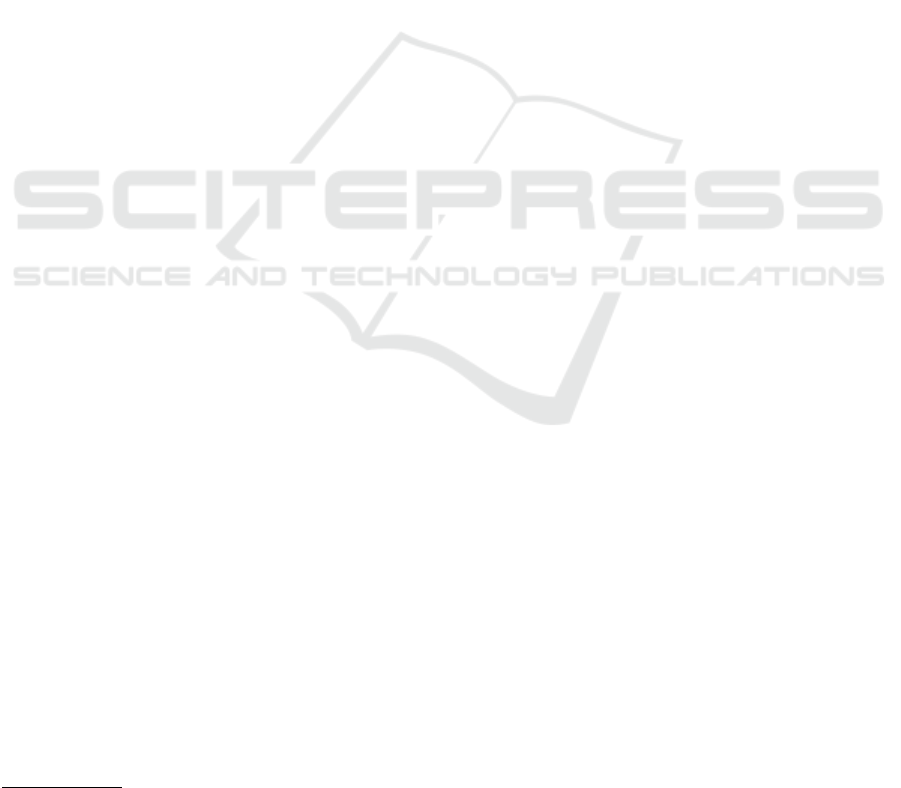
(∆GAP close to zero). However, although NMF+CP
was able to achieve higher MAP and MRR than our
proposal, we obtained higher MRMC Genre, indicat-
ing that our method can provide genre and popularity
calibration at the same time
9
.
6 CONCLUSION AND FUTURE
WORK
In this paper, we proposed a personalized calibra-
tion technique, which uses popularity and genre cali-
brations in a switch-based approach to provide fairer
recommendations to users according to their inter-
ests. Our main contribution is the possibility to
calibrate recommendations generated by any recom-
mender model, whose choice could be according to
application requirements.
We showed that the calibration of items based on
the popularity aspect is a way to improve a recom-
mendation system to bring fairer recommendations to
users to meet their preferences and reduce the impact
of popularity bias in the system. We presented a cal-
ibration approach that works in the post-processing
step and is independent of any recommendation algo-
rithm.
Our experiments showed that our proposal could
reduce the popularity bias, recommending less popu-
lar items by covering the long tail and consequently
increasing diversity and fairness related to genres
and popularity in recommendations. Although we
achieved better results in precision against genre cal-
ibration, other methods provided more accurate rec-
ommendations, but at the cost of higher popularity
bias.
In future work, we plan to analyze the effect of
our calibration with other recommendation models,
particularly considering the aspects of precision and
popularity bias. We will also conduct online experi-
ments to verify the performance of the proposed cal-
ibration system with real users. In addition, we plan
to evaluate our approaches with other metadata and
different contexts.
ACKNOWLEDGEMENTS
The authors would like to thank the financial support
from FAPESP, process number 2022/07016-9.
9
In this comparison, we selected the λ 6= 0 with the
highest LTC value.
REFERENCES
Abdollahpouri, H., Burke, R., and Mobasher, B. (2018).
Popularity-aware item weighting for long-tail recom-
mendation. arXiv.
Abdollahpouri, H., Mansoury, M., Burke, R., and
Mobasher, B. (2019a). The impact of popularity bias
on fairness and calibration in recommendation.
Abdollahpouri, H., Mansoury, M., Burke, R., and
Mobasher, B. (2019b). The unfairness of pop-
ularity bias in recommendation. arXiv preprint
arXiv:1907.13286.
Abdollahpouri, H., Mansoury, M., Burke, R., and
Mobasher, B. (2020). The connection between pop-
ularity bias, calibration, and fairness in recommenda-
tion. In Fourteenth ACM conference on recommender
systems, pages 726–731.
Abdollahpouri, H., Mansoury, M., Burke, R., Mobasher, B.,
and Malthouse, E. (2021). User-centered evaluation of
popularity bias in recommender systems. In Proceed-
ings of the 29th ACM Conference on User Modeling,
Adaptation and Personalization, pages 119–129.
Beutel, A., Chen, J., Doshi, T., Qian, H., Wei, L., Wu,
Y., Heldt, L., Zhao, Z., Hong, L., Chi, E. H., et al.
(2019). Fairness in recommendation ranking through
pairwise comparisons. In Proceedings of the 25th
ACM SIGKDD International Conference on Knowl-
edge Discovery & Data Mining, pages 2212–2220.
Boratto, L., Fenu, G., and Marras, M. (2021). Connecting
user and item perspectives in popularity debiasing for
collaborative recommendation. Information Process-
ing & Management, 58(1):102387.
Borges, R. and Stefanidis, K. (2021). On mitigating pop-
ularity bias in recommendations via variational au-
toencoders. In Proceedings of the 36th Annual ACM
Symposium on Applied Computing, SAC ’21, page
1383–1389, New York, NY, USA. Association for
Computing Machinery.
Cha, S.-H. (2007). Comprehensive survey on dis-
tance/similarity measures between probability den-
sity functions. International Journal of Mathematical
Models and Methods in Applied Sciences, 1(4):300–
307.
da Silva, D. C., Manzato, M. G., and Dur
˜
ao, F. A. (2021).
Exploiting personalized calibration and metrics for
fairness recommendation. Expert Systems with Appli-
cations, 181:115112.
de Winter, J. (2013). Using the student’s t-test with ex-
tremely small sample sizes. Practical Assessment, Re-
search & Evaluation, 18.
Ekstrand, M. D., Tian, M., Azpiazu, I. M., Ekstrand, J. D.,
Anuyah, O., McNeill, D., and Pera, M. S. (2018).
All the cool kids, how do they fit in?: Popularity
and demographic biases in recommender evaluation
and effectiveness. In Friedler, S. A. and Wilson, C.,
editors, Conference on Fairness, Accountability and
Transparency, FAT 2018, 23-24 February 2018, New
York, NY, USA, volume 81 of Proceedings of Machine
Learning Research, pages 172–186. PMLR.
Counteracting Popularity-Bias and Improving Diversity Through Calibrated Recommendations
719