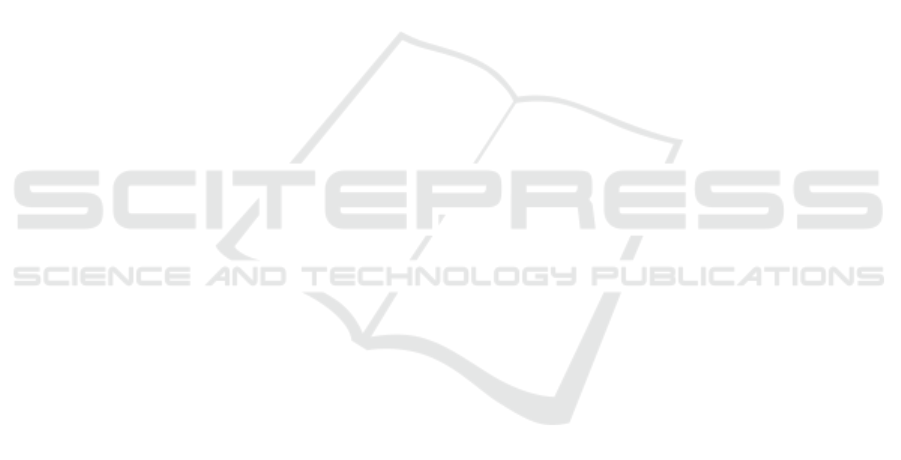
art in artificial neural network applications: A survey.
Heliyon, 4(11):e00938.
Aftabuzzaman, M. (2007). Measuring traffic congestion-
a critical review. In 30th Australasian Transport Re-
search Forum.
Arco, E., Ajmar, A., Arneodo, F., and Boccardo, P. (2017).
An operational framework to integrate traffic message
channel (tmc)in emergency mapping services (ems).
European Journal of Remote Sensing, 50(1):478–495.
Bai, J., Zhu, J., Song, Y., Zhao, L., Hou, Z., Du, R., and Li,
H. (2021). A3t-gcn: Attention temporal graph convo-
lutional network for traffic forecasting. ISPRS Inter-
national Journal of Geo-Information, 10(7):485.
Bergstra, J., Bardenet, R., Bengio, Y., and K
´
egl, B. (2011).
Algorithms for hyper-parameter optimization. Ad-
vances in neural information processing systems, 24.
Cowan, R. and Jonard, N. (2004). Network structure and
the diffusion of knowledge. Journal of economic Dy-
namics and Control, 28(8):1557–1575.
Gao, Y. and Wen, H.-m. (2007). Technique and standard-
ization research of radio data system-traffic message
channel (rds-tmc). Journal of Transportation Systems
Engineering and Information Technology, 3.
Guo, S., Lin, Y., Feng, N., Song, C., and Wan, H. (2019).
Attention based spatial-temporal graph convolutional
networks for traffic flow forecasting. In Proceedings
of the AAAI conference on artificial intelligence, vol-
ume 33, pages 922–929.
Hadorn, G. H., Biber-Klemm, S., Grossenbacher-Mansuy,
W., Hoffmann-Riem, H., Joye, D., Pohl, C., Wies-
mann, U., and Zemp, E. (2008). The emergence of
transdisciplinarity as a form of research. In Handbook
of transdisciplinary research, pages 19–39. Springer.
Hevner, A. and Chatterjee, S. (2010). Design research in
information systems: theory and practice, volume 22.
Springer Science & Business Media.
Li, Y., Yu, R., Shahabi, C., and Liu, Y. (2017). Diffusion
convolutional recurrent neural network: Data-driven
traffic forecasting. arXiv preprint arXiv:1707.01926.
Lin, H.-E., Zito, R., Taylor, M., et al. (2005). A review
of travel-time prediction in transport and logistics. In
Proceedings of the Eastern Asia Society for trans-
portation studies, volume 5, pages 1433–1448.
Mori, U., Mendiburu, A.,
´
Alvarez, M., and Lozano, J. A.
(2015). A review of travel time estimation and fore-
casting for advanced traveller information systems.
Transportmetrica A: Transport Science, 11(2):119–
157.
Pincay, J., Mensah, A. O., Portmann, E., and Ter
´
an, L.
(2020). Forecasting travel times with space partition-
ing methods. In Grueau, C., Laurini, R., and Ragia,
L., editors, Proceedings of the 6th International Con-
ference on Geographical Information Systems The-
ory, Applications and Management, GISTAM 2020,
Prague, Czech Republic, May 7-9, 2020, pages 151–
159. SCITEPRESS.
Pincay, J., Portmann, E., and Ter
´
an, L. (2021). Mining
linguistic summaries in traffic. In B
¨
ack, T., Wag-
ner, C., Garibaldi, J. M., Lam, H. K., Cottrell, M.,
Merelo, J. J., and Warwick, K., editors, Proceedings
of the 13th International Joint Conference on Compu-
tational Intelligence, IJCCI 2021, Online Streaming,
October 25-27, 2021, pages 169–176. SCITEPRESS.
Rozemberczki, B., Scherer, P., He, Y., Panagopoulos, G.,
Riedel, A., Astefanoaei, M., Kiss, O., Beres, F.,
L
´
opez, G., Collignon, N., et al. (2021). Pytorch ge-
ometric temporal: Spatiotemporal signal processing
with neural machine learning models. In Proceedings
of the 30th ACM International Conference on Infor-
mation & Knowledge Management, pages 4564–4573.
Shuman, D. I., Narang, S. K., Frossard, P., Ortega, A., and
Vandergheynst, P. (2013). The emerging field of signal
processing on graphs: Extending high-dimensional
data analysis to networks and other irregular domains.
IEEE signal processing magazine, 30(3):83–98.
Treiber, M. and Kesting, A. (2013). Trajectory and floating-
car data. In Traffic Flow Dynamics, pages 7–12.
Springer.
Wang, S.-C. (2003). Artificial Neural Network, pages 81–
100. Springer US, Boston, MA.
Wu, Z., Pan, S., Chen, F., Long, G., Zhang, C., and Philip,
S. Y. (2020). A comprehensive survey on graph neural
networks. IEEE transactions on neural networks and
learning systems, 32(1):4–24.
Yu, B., Yin, H., and Zhu, Z. (2017). Spatio-temporal
graph convolutional networks: A deep learning
framework for traffic forecasting. arXiv preprint
arXiv:1709.04875.
Zhou, P., Zheng, Y., and Li, M. (2012). How long to wait?:
predicting bus arrival time with mobile phone based
participatory sensing.
Zhu, H., Xie, Y., He, W., Sun, C., Zhu, K., Zhou, G.,
and Ma, N. (2020). A novel traffic flow forecasting
method based on rnn-gcn and brb. Journal of Ad-
vanced Transportation, 2020.
GISTAM 2023 - 9th International Conference on Geographical Information Systems Theory, Applications and Management
158