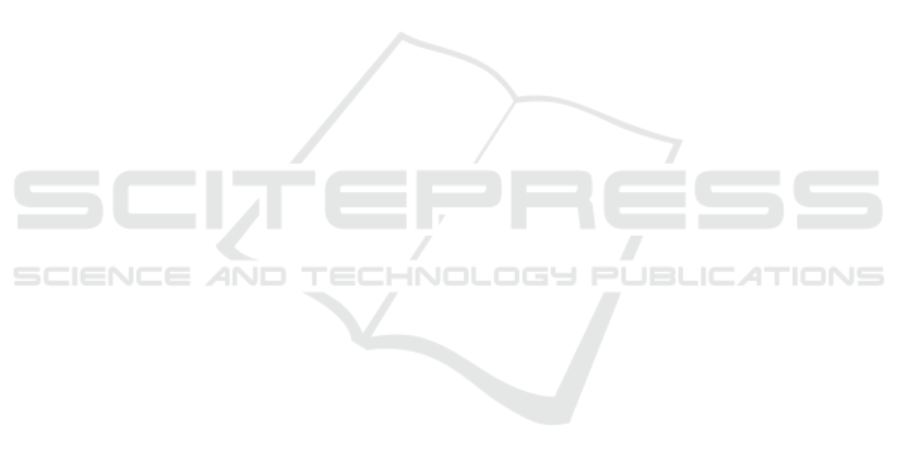
Abtahi, S., Omidyeganeh, M., Shirmohammadi, S., and
Hariri, B. (2020). Yawdd: Yawning detection dataset.
Bai, J., Yu, W., Xiao, Z., Havyarimana, V., Regan, A. C.,
Jiang, H., and Jiao, L. (2021). Two-stream spatial-
temporal graph convolutional networks for driver
drowsiness detection. IEEE Transactions on Cyber-
netics, pages 1–13.
Carreira, J. and Zisserman, A. (2017). Quo vadis, action
recognition? a new model and the kinetics dataset.
In proceedings of the IEEE Conference on Computer
Vision and Pattern Recognition, pages 6299–6308.
Deng, J., Guo, J., Ververas, E., Kotsia, I., and Zafeiriou, S.
(2020). Retinaface: Single-shot multi-level face local-
isation in the wild. In Proceedings of the IEEE/CVF
Conference on Computer Vision and Pattern Recogni-
tion (CVPR).
Ding, L., Tang, H., and Bruzzone, L. (2020). Lanet: Local
attention embedding to improve the semantic segmen-
tation of remote sensing images. IEEE Transactions
on Geoscience and Remote Sensing, 59(1):426–435.
Dong, B.-T. and Lin, H.-Y. (2021). An on-board monitor-
ing system for driving fatigue and distraction detec-
tion. In 2021 22nd IEEE International Conference on
Industrial Technology (ICIT), volume 1, pages 850–
855. IEEE.
Doudou, M., Bouabdallah, A., and Berge-Cherfaoui, V.
(2020). Driver drowsiness measurement technolo-
gies: Current research, market solutions, and chal-
lenges. International Journal of Intelligent Trans-
portation Systems Research, 18(2):297–319.
Feichtenhofer, C., Fan, H., Malik, J., and He, K. (2019).
Slowfast networks for video recognition. In Proceed-
ings of the IEEE/CVF international conference on
computer vision, pages 6202–6211.
Jap, B. T., Lal, S., Fischer, P., and Bekiaris, E. (2009). Us-
ing eeg spectral components to assess algorithms for
detecting fatigue. Expert Systems with Applications,
36(2):2352–2359.
Krizhevsky, A., Sutskever, I., and Hinton, G. E. (2012). Im-
agenet classification with deep convolutional neural
networks. In Pereira, F., Burges, C. J. C., Bottou, L.,
and Weinberger, K. Q., editors, Advances in Neural
Information Processing Systems, volume 25. Curran
Associates, Inc.
Lin, X., Li, J., Ma, Z., Li, H., Li, S., Xu, K., Lu, G.,
and Zhang, D. (2022). Learning modal-invariant and
temporal-memory for video-based visible-infrared
person re-identification. In Proceedings of the
IEEE/CVF Conference on Computer Vision and Pat-
tern Recognition, pages 20973–20982.
Liu, W., Qian, J., Yao, Z., Jiao, X., and Pan, J. (2019). Con-
volutional two-stream network using multi-facial fea-
ture fusion for driver fatigue detection. Future Inter-
net, 11(5):115.
Lyu, J., Yuan, Z., and Chen, D. (2018). Long-term multi-
granularity deep framework for driver drowsiness de-
tection. arXiv preprint arXiv:1801.02325.
Murugan, S., Selvaraj, J., and Sahayadhas, A. (2020). De-
tection and analysis: driver state with electrocardio-
gram (ecg). Physical and engineering sciences in
medicine, 43(2):525–537.
Park, S., Pan, F., Kang, S., and Yoo, C. D. (2016). Driver
drowsiness detection system based on feature repre-
sentation learning using various deep networks. In
Asian Conference on Computer Vision, pages 154–
164. Springer.
Quddus, A., Zandi, A. S., Prest, L., and Comeau, F. J.
(2021). Using long short term memory and convolu-
tional neural networks for driver drowsiness detection.
Accident Analysis & Prevention, 156:106107.
Savas¸, B. K. and Becerikli, Y. (2020). Real time driver
fatigue detection system based on multi-task connn.
Ieee Access, 8:12491–12498.
Sikander, G. and Anwar, S. (2018). Driver fatigue detection
systems: A review. IEEE Transactions on Intelligent
Transportation Systems, 20(6):2339–2352.
Wang, Q., Wu, B., Zhu, P., Li, P., Zuo, W., and Hu, Q.
(2020). Eca-net: Efficient channel attention for deep
convolutional neural networks. In 2020 IEEE/CVF
Conference on Computer Vision and Pattern Recog-
nition (CVPR), pages 11531–11539.
Warnecke, J. M., Ganapathy, N., Koch, E., Dietzel, A., Flor-
mann, M., Henze, R., and Deserno, T. M. (2022).
Printed and flexible ecg electrodes attached to the
steering wheel for continuous health monitoring dur-
ing driving. Sensors, 22(11):4198.
Weng, C.-H., Lai, Y.-H., and Lai, S.-H. (2016). Driver
drowsiness detection via a hierarchical temporal deep
belief network. In Asian Conference on Computer Vi-
sion, pages 117–133. Springer.
Woo, S., Park, J., Lee, J.-Y., and Kweon, I. S. (2018). Cbam:
Convolutional block attention module. In Proceed-
ings of the European conference on computer vision
(ECCV), pages 3–19.
Xue, F., Wang, Q., and Guo, G. (2021). Transfer: Learn-
ing relation-aware facial expression representations
with transformers. In Proceedings of the IEEE/CVF
International Conference on Computer Vision, pages
3601–3610.
You, F., Gong, Y., Tu, H., Liang, J., and Wang, H. (2020).
A fatigue driving detection algorithm based on fa-
cial motion information entropy. Journal of advanced
transportation, 2020.
Yu, J., Park, S., Lee, S., and Jeon, M. (2016). Represen-
tation learning, scene understanding, and feature fu-
sion for drowsiness detection. In Asian Conference on
Computer Vision, pages 165–177. Springer.
Zhang, J.-Z. and Lin, H.-Y. (2021). Driving behavior analy-
sis and traffic improvement using onboard sensor data
and geographic information. In VEHITS, pages 284–
291.
Zhang, Y.-F., Gao, X.-Y., Zhu, J.-Y., Zheng, W.-L., and Lu,
B.-L. (2015). A novel approach to driving fatigue
detection using forehead eog. In 2015 7th Interna-
tional IEEE/EMBS Conference on Neural Engineer-
ing (NER), pages 707–710. IEEE.
VEHITS 2023 - 9th International Conference on Vehicle Technology and Intelligent Transport Systems
280