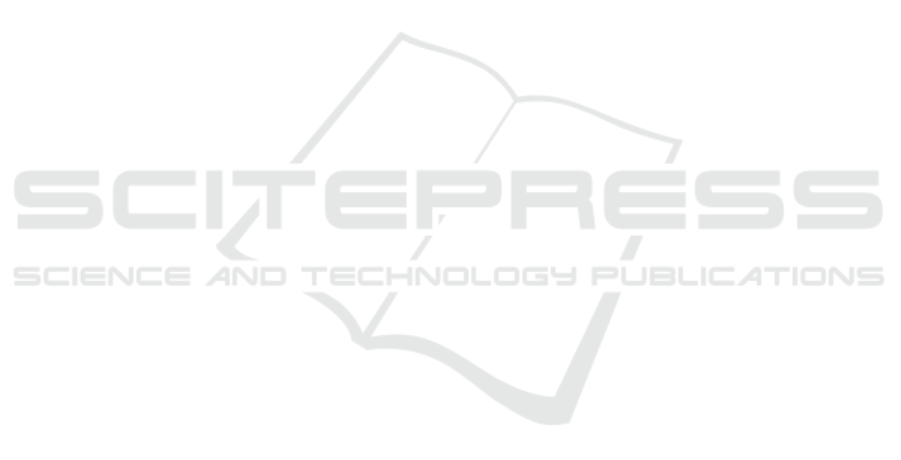
ing for modeling students’ performance: A tutoring
action plan to prevent academic dropout. Computers
& Electrical Engineering, 66:541–556.
Cameron, A. C. and Trivedi, P. K. (2005). Microecono-
metrics : methods and applications. Cambridge Univ.
Press.
Chen, Y., Zheng, Q., Ji, S., Tian, F., Zhu, H., and Liu,
M. (2020). Identifying at-risk students based on the
phased prediction model. Knowledge and Information
Systems, 62(3):987–1003.
Chung, J. Y. and Lee, S. (2019). Dropout early warning sys-
tems for high school students using machine learning.
Children and Youth Services Review, 96:346–353.
S¸ ahin, M. and Yurdug
¨
ul, H. (2019). An intervention en-
gine design and development based on learning an-
alytics: the intelligent intervention system (in 2 s).
Smart Learning Environments, 6(1):18.
Edmunds, K. and Tancock, S. M. (2002). Incentives: The
effects on the reading motivation of fourth-grade stu-
dents. Reading Research and Instruction, 42(2):17–
37.
Gray, G., McGuinness, C., and Owende, P. (2014). An
application of classification models to predict learner
progression in tertiary education. In International
Advance Computing Conference (IACC), pages 549–
554. IEEE.
Huang, S. and Fang, N. (2013). Predicting student academic
performance in an engineering dynamics course: A
comparison of four types of predictive mathematical
models. Computers & Education, 61:133–145.
Huntington-Klein, N. (2022). The effect : an introduction
to research design and causality. Chapman & Hall
book. CRC Press, Taylor & Francis Group, Boca Ra-
ton ; London ; New York, first edition edition.
Imbens, G. and Kalyanaraman, K. (2009). Optimal band-
width choice for the regression discontinuity esti-
mator. National Bureau of Economic Research,
1(14726).
Jacob, B. A. and Lefgren, L. (2004). Remedial ed-
ucation and student achievement: A regression-
discontinuity analysis. Review of economics and
statistics, 86(1):226–244.
Koc¸ak,
¨
O., G
¨
oksu,
˙
I., and G
¨
oktas, Y. (2021). The factors
affecting academic achievement: A systematic review
of meta analyses. International Online Journal of Ed-
ucation and Teaching, 8(1):454–484.
Laur
´
ıa, E. J., Moody, E. W., Jayaprakash, S. ., Jonnala-
gadda, N., and Baron, J. D. (2013). Open academic
analytics initiative: initial research findings. In Pro-
ceedings of the Third International Conference on
Learning Analytics and Knowledge, pages 150–154.
Lee, D. S. and Lemieux, T. (2010). Regression discontinu-
ity designs in economics. 48(2):281.
Lu, O. H. T., Huang, A. Y. Q., Huang, J. C. H., Lin, A.
J. Q., Ogata, H., and Yang, S. J. H. (2018). Applying
learning analytics for the early prediction of students’
academic performance in blended learning. Journal of
Educational Technology & Society, 21(2):220–232.
Mac Iver, M. A., Stein, M. L., Davis, M. H., Balfanz, R. W.,
and Fox, J. H. (2019). An efficacy study of a ninth-
grade early warning indicator intervention. Journal
of Research on Educational Effectiveness, 12(3):363–
390.
Macfadyen, L. P. and Dawson, S. (2010). Mining LMS
data to develop an ”early warning system” for edu-
cators: A proof of concept. Computers & Education,
54(2):588–599.
Massing, T., Reckmann, N., Otto, B., Hermann, K. J.,
Hanck, C., and Goedicke, M. (2018a). Klausurprog-
nose mit Hilfe von E-Assessment-Nutzerdaten. In
DeLFI 2018 - Die 16. E-Learning Fachtagung Infor-
matik, pages 171–176.
Massing, T., Schwinning, N., Striewe, M., Hanck, C., and
Goedicke, M. (2018b). E-assessment using variable-
content exercises in mathematical statistics. Journal
of Statistics Education, 26(3):174–189.
McCrary, J. (2008). Manipulation of the running variable
in the regression discontinuity design: A density test.
Journal of Econometrics, 142(2):698–714.
McEwan, P. J. and Shapiro, J. S. (2008). The benefits of
delayed primary school enrollment discontinuity esti-
mates using exact birth dates. The Journal of human
Resources, 43(1):1–29.
Oskouei, R. J. and Askari, M. (2014). Predicting Academic
Performance with Applying Data Mining Techniques
(Generalizing the results of Two Different Case Stud-
ies). Computer Engineering and Applications Jour-
nal, 3(2):79–88.
Parkin, H. J., Hepplestone, S., Holden, G., Irwin, B., and
Thorpe, L. (2012). A role for technology in enhancing
students’ engagement with feedback. Assessment &
Evaluation in Higher Education, 37(8):963–973.
Schiefele, U., Krapp, A., and Winteler, A. (1992). In-
terest as a predictor of academic achievement: A
meta-analysis of research, chapter 8, pages 183–212.
Lawrence Erlbaum Associates, Inc, New York, 1 edi-
tion.
Sosa, G. W., Berger, D. E., Saw, A. T., and Mary, J. C.
(2011). Effectiveness of computer-assisted instruction
in statistics: A meta-analysis. Review of Educational
Research, 81(1):97–128.
Stanfield, G. M. (2008). Incentives: The effects on reading
attitude and reading behaviors of third-grade students.
The Corinthian, 9(1):8.
Wolff, A., Zdrahal, Z., Nikolov, A., and Pantucek, M.
(2013). Improving retention: Predicting at-risk stu-
dents by analysing clicking behaviour in a virtual
learning environment. In Proceedings of the Third
International Conference on Learning Analytics and
Knowledge, LAK ’13, pages 145–149, New York, NY,
USA. ACM.
CSEDU 2023 - 15th International Conference on Computer Supported Education
232