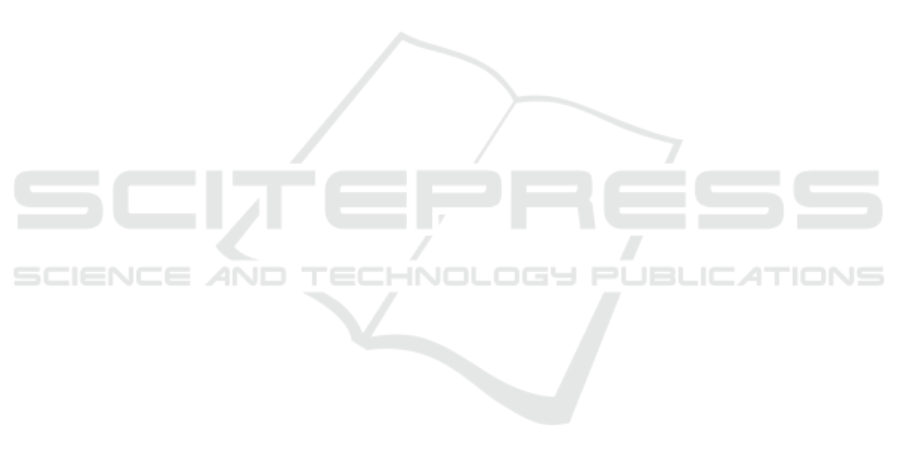
mation Processing Systems, volume 30. Curran Asso-
ciates, Inc.
Devlin, J., Chang, M.-W., Lee, K., and Toutanova,
K. (2018). Bert: Pre-training of deep bidirec-
tional transformers for language understanding. cite
arxiv:1810.04805Comment: 13 pages.
Everingham, M., Eslami, S. M. A., Van Gool, L., Williams,
C. K. I., Winn, J., and Zisserman, A. (2015). The pas-
cal visual object classes challenge: A retrospective.
International Journal of Computer Vision, 111(1):98–
136.
Huaman, R. N. E. and Jun, T. X. (2014). Energy related co2
emissions and the progress on ccs projects: a review.
Renewable and Sustainable Energy Reviews, 31:368–
385.
Ibrahim, E., Jouini, M., Bouchaala, F., and Gomes, J.
(2021). Simulation and validation of porosity and
permeability of synthetic and real rock models using
three-dimensional printing and digital rock physics.
ACS Omega.
IPCC (2022). Summary for Policymakers. Cambridge Uni-
versity Press, Cambridge, UK. In Press.
Johnson-Roberson, M., Barto, C., Mehta, R., Sridhar, S. N.,
and Vasudevan, R. (2016). Driving in the matrix: Can
virtual worlds replace human-generated annotations
for real world tasks? CoRR, abs/1610.01983.
Khan, S., Phan, B., Salay, R., and Czarnecki, K. (2019).
Procsy: Procedural synthetic dataset generation to-
wards influence factor studies of semantic segmenta-
tion networks. In Proceedings of the IEEE/CVF Con-
ference on Computer Vision and Pattern Recognition
(CVPR) Workshops.
Kirichenko, P., Izmailov, P., and Wilson, A. G. (2022). Last
layer re-training is sufficient for robustness to spurious
correlations.
Kirkpatrick, J., Pascanu, R., Rabinowitz, N., Veness, J.,
Desjardins, G., Rusu, A. A., Milan, K., Quan, J.,
Ramalho, T., Grabska-Barwinska, A., Hassabis, D.,
Clopath, C., Kumaran, D., and Hadsell, R. (2017).
Overcoming catastrophic forgetting in neural net-
works. Proceedings of the National Academy of Sci-
ences, 114(13):3521–3526.
Kramer, R. J., He, H., Soden, B. J., Oreopoulos,
L., Myhre, G., Forster, P. M., and Smith, C. J.
(2021). Observational evidence of increasing global
radiative forcing. Geophysical Research Letters,
48(7):e2020GL091585.
Kristj
´
ansd
´
ottir, H. and Kristj
´
ansd
´
ottir, S. (2021). Carbfix
and sulfix in geothermal production, and the blue la-
goon in iceland: Grindav
´
ık urban settlement, and vol-
canic activity. Baltic Journal of Economic Studies,
7(1):1–9.
Lee, J., Tang, R., and Lin, J. (2019). What would elsa do?
freezing layers during transformer fine-tuning. ArXiv,
abs/1911.03090.
Lee, Y., Chen, A. S., Tajwar, F., Kumar, A., Yao, H., Liang,
P., and Finn, C. (2022). Surgical fine-tuning improves
adaptation to distribution shifts.
Lin, T., Maire, M., Belongie, S. J., Bourdev, L. D., Girshick,
R. B., Hays, J., Perona, P., Ramanan, D., Doll
´
ar, P.,
and Zitnick, C. L. (2014). Microsoft COCO: common
objects in context. CoRR, abs/1405.0312.
Myung, S., Huh, I., Jang, W., Choe, J. M., Ryu, J., Kim,
D., Kim, K.-E., and Jeong, C. (2022). Pac-net: A
model pruning approach to inductive transfer learning.
In International Conference on Machine Learning.
Nichol, A., Dhariwal, P., Ramesh, A., Shyam, P., Mishkin,
P., McGrew, B., Sutskever, I., and Chen, M. (2021).
GLIDE: towards photorealistic image generation and
editing with text-guided diffusion models. CoRR,
abs/2112.10741.
Nikolenko, S. (2021). Synthetic Data for Deep Learning.
Springer Optimization and Its Applications. Springer
International Publishing.
Oquab, M., Bottou, L., Laptev, I., and Sivic, J. (2014).
Learning and transferring mid-level image represen-
tations using convolutional neural networks. In 2014
IEEE Conference on Computer Vision and Pattern
Recognition, pages 1717–1724.
Qiu, W., Zhong, F., Zhang, Y., Qiao, S., Xiao, Z., Kim,
T. S., and Wang, Y. (2017). UnrealCV: Virtual worlds
for computer vision. In Proceedings of the 25th
ACM International Conference on Multimedia, page
1221–1224. Association for Computing Machinery.
Razavian, A. S., Azizpour, H., Sullivan, J., and Carlsson,
S. (2014). Cnn features off-the-shelf: an astounding
baseline for recognition.
Saraf, S. and Bera, A. (2021). A review on pore-scale mod-
eling and ct scan technique to characterize the trapped
carbon dioxide in impermeable reservoir rocks during
sequestration. Renewable and Sustainable Energy Re-
views, 144:110986.
Sener, O., Song, H. O., Saxena, A., and Savarese, S.
(2016). Learning transferrable representations for un-
supervised domain adaptation. In Lee, D., Sugiyama,
M., Luxburg, U., Guyon, I., and Garnett, R., editors,
Advances in Neural Information Processing Systems,
volume 29. Curran Associates, Inc.
Shen, Z., Liu, Z., Qin, J., Savvides, M., and Cheng,
K.-T. (2021). Partial is better than all: Revisiting
fine-tuning strategy for few-shot learning. ArXiv,
abs/2102.03983.
Varsavsky, T., Orbes-Arteaga, M., Sudre, C. H., Graham,
M. S., Nachev, P., and Cardoso, M. J. (2020). Test-
time unsupervised domain adaptation. In Martel,
A. L., Abolmaesumi, P., Stoyanov, D., Mateus, D.,
Zuluaga, M. A., Zhou, S. K., Racoceanu, D., and
Joskowicz, L., editors, Medical Image Computing
and Computer Assisted Intervention. Springer Inter-
national Publishing.
Wang, B., Chen, Y., Lu, J., and Jin, W. (2018). A rock
physics modelling algorithm for simulating the elastic
parameters of shale using well logging data. Scientific
Reports, 8.
Wennersten, R., Sun, Q., and Li, H. (2015). The future
potential for carbon capture and storage in climate
change mitigation–an overview from perspectives of
technology, economy and risk. Journal of Cleaner
Production, 103:724–736.
ICPRAM 2023 - 12th International Conference on Pattern Recognition Applications and Methods
572