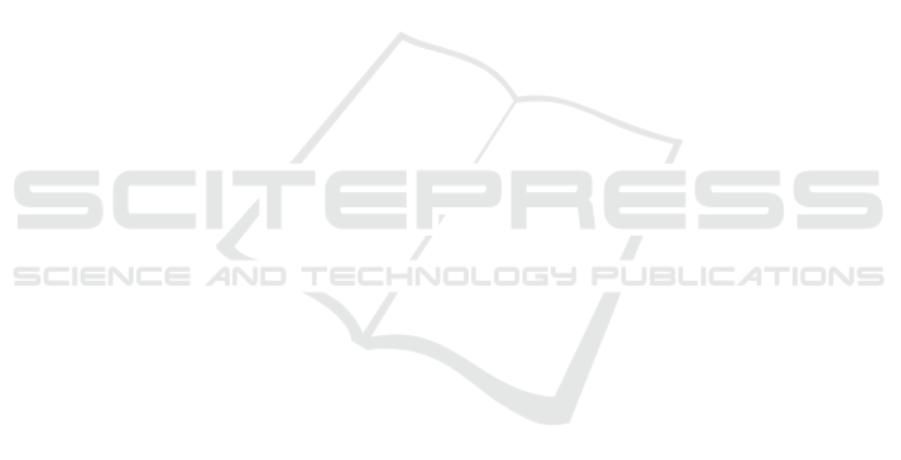
d04-012-10. Finally, an example of deletion is present
in the case of sample csg562-042-24. As already
mentioned, errors are present in the datasets. In the
IAM dataset, the GT transcriptions have many more
spaces than the correct ones, especially before punc-
tuation marks. Moreover, errors in the transcription
are present, such as in the case of d04-012-10 where
the GT transcription contains the final dot not present
in the image itself.
5 CONCLUSIONS
We empirically demonstrated that relaxation label-
ing processes help in generalisation abilities for
well-established architectures in the HTR field, the
CRNNs. Such processes can drive the network to-
wards a more consistent labeling output in all the
datasets considered. They improve the results in
terms of both validation and test CER and WER. As
a future work we consider to compare the relaxation
labeling module with attention mechanisms, which
have a similar role of contextual processing from the
context. Finally, we plan to conduct a more exten-
sive comparison of our proposed method with other
backbones, to consistently evaluate the performance
improvement provided by relaxation labeling.
ACKNOWLEDGEMENTS
We would especially like to thank Gregory Sech for
his valuable suggestions and his past work on Relax-
ation Labeling processes. Also, we want to thank the
Italian Institute of Technology (IIT) for the possibil-
ity to use their High-Performance Computing (HPC)
as it allowed for faster experimentation.
REFERENCES
Aradillas, J. C., Murillo-Fuentes, J. J., and Olmos, P. M.
(2020). Improving offline htr in small datasets by
purging unreliable labels. In 2020 17th International
Conference on Frontiers in Handwriting Recognition
(ICFHR), pages 25–30. IEEE.
Baydin, A. G., Pearlmutter, B. A., Radul, A. A., and
Siskind, J. M. (2018). Automatic differentiation in
machine learning: a survey. Journal of Marchine
Learning Research, 18:1–43.
Bluche, T., Louradour, J., and Messina, R. (2017). Scan,
attend and read: End-to-end handwritten paragraph
recognition with mdlstm attention. In 2017 14th IAPR
international conference on document analysis and
recognition (ICDAR), volume 1, pages 1050–1055.
IEEE.
Bridle, J. (1989). Training stochastic model recognition al-
gorithms as networks can lead to maximum mutual in-
formation estimation of parameters. Advances in neu-
ral information processing systems, 2.
Coquenet, D., Soullard, Y., Chatelain, C., and Paquet, T.
(2019). Have convolutions already made recurrence
obsolete for unconstrained handwritten text recogni-
tion? In 2019 International conference on document
analysis and recognition workshops (ICDARW), vol-
ume 5, pages 65–70. IEEE.
Fischer, A., Frinken, V., Forn
´
es, A., and Bunke, H. (2011).
Transcription alignment of latin manuscripts using
hidden markov models. In Proceedings of the 2011
Workshop on Historical Document Imaging and Pro-
cessing, pages 29–36.
Fischer, A., Keller, A., Frinken, V., and Bunke, H. (2012).
Lexicon-free handwritten word spotting using charac-
ter hmms. Pattern recognition letters, 33(7):934–942.
Goshtasby, A. and Ehrich, R. W. (1988). Contextual
word recognition using probabilistic relaxation label-
ing. Pattern Recognition, 21(5):455–462.
Graves, A., Fern
´
andez, S., Gomez, F., and Schmidhu-
ber, J. (2006). Connectionist temporal classification:
labelling unsegmented sequence data with recurrent
neural networks. In Proceedings of the 23rd interna-
tional conference on Machine learning, pages 369–
376.
Guo, J. (2013). Backpropagation through time. Unpubl.
ms., Harbin Institute of Technology, 40:1–6.
Haralick, R. M. and Shapiro, L. G. (1979). The consistent
labeling problem: Part i. IEEE Transactions on Pat-
tern Analysis and Machine Intelligence, (2):173–184.
Hochreiter, S. and Schmidhuber, J. (1997). Long short-term
memory. Neural computation, 9(8):1735–1780.
Hummel, R. A. and Zucker, S. W. (1983). On the foun-
dations of relaxation labeling processes. IEEE Trans-
actions on Pattern Analysis and Machine Intelligence,
(3):267–287.
Kingma, D. P. and Ba, J. (2014). Adam: A
method for stochastic optimization. arXiv preprint
arXiv:1412.6980.
Li, M., Lv, T., Cui, L., Lu, Y., Florencio, D., Zhang, C.,
Li, Z., and Wei, F. (2021). Trocr: Transformer-based
optical character recognition with pre-trained models.
arXiv preprint arXiv:2109.10282.
Lombardi, F. and Marinai, S. (2020). Deep learning for his-
torical document analysis and recognition—a survey.
Journal of Imaging, 6(10):110.
Marti, U.-V. and Bunke, H. (2002). The iam-database:
an english sentence database for offline handwrit-
ing recognition. International Journal on Document
Analysis and Recognition, 5(1):39–46.
Miller, D. A. and Zucker, S. W. (1991). Copositive-plus
lemke algorithm solves polymatrix games. Operations
research letters, 10(5):285–290.
Pelillo, M. (1997). The dynamics of nonlinear relaxation
labeling processes. Journal of Mathematical Imaging
and Vision, 7(4):309–323.
ICPRAM 2023 - 12th International Conference on Pattern Recognition Applications and Methods
580