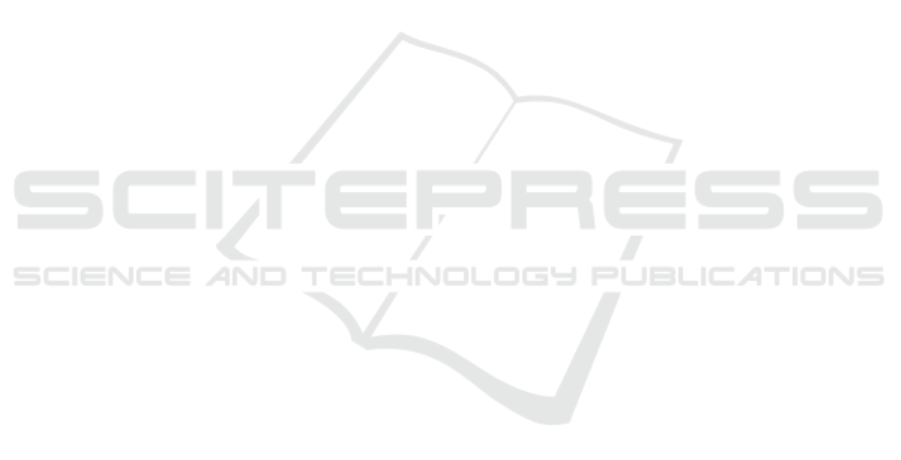
individuals not even know they have such a disease.
Considering the problems mentioned above, the
objective of this work is to identify forecasting and
classification methodologies, mainly involving fuzzy
logic, used to help the health area.
To this end, a systematic review was carried out
with the objective of finding prediction and classifica-
tion methods for diabetes or even for other diseases,
but that could help in understanding the state of the
art, that is, that could facilitate the understanding of
which techniques, currently, are being used to predict
or classify health problems.
In order to carry out the systematic review, inclu-
sion and exclusion criteria were considered so that the
works found could be filtered and, at the end, those
that best met the pre-established conditions could be
studied.
Thus, at the end of the work, it was possible to
observe that techniques using fuzzy logic, such as:
Fuzzy C−Means, ANFIS and Takagi-Sugeno zero or-
der fuzzy modeling are being used and from that it
can be observed which is the best way to contribute
in the area proposing techniques that can improve
the quality/perfomance/explainability/interpretability
of the existing related methods.
The next step, therefore, consists of implementing
a fuzzy methodology for predicting diabetes based on
blood test variables and also on information provided
by the patients themselves, such as: sleep, physical
activity and diet.
ACKNOWLEDGEMENTS
This work is supported by the Academic Master’s
and Doctorate Program for Innovation of the National
Council for Scientific and Technological Develop-
ment CNPq.
Public Notice FAPERGS/CNPq 07/2022 - Pro-
gram to Support the Settlement of Young Doctors in
Brazil
REFERENCES
Bergquist, S. L., Brooks, G. A., Keating, N. L., Landrum,
M. B., and Rose, S. (2017). Classifying lung can-
cer severity with ensemble machine learning in health
care claims data. In Machine Learning for Healthcare
Conference, pages 25–38. PMLR.
Bonaccorso, G. (2017). Machine learning algorithms.
Packt Publishing Ltd.
Cordeiro, A. M., Oliveira, G. M. d., Renter
´
ıa, J. M., and
Guimar
˜
aes, C. A. (2007). Revis
˜
ao sistem
´
atica: uma
revis
˜
ao narrativa. Revista do Col
´
egio Brasileiro de
Cirurgi
˜
oes, 34:428–431.
Deif, M., Hammam, R., and Solyman, A. (2021). Adaptive
neuro-fuzzy inference system (anfis) for rapid diagno-
sis of covid-19 cases based on routine blood tests. Int.
J. Intell. Eng. Syst, 14(2):178–189.
Dos Santos, H. G., do Nascimento, C. F., Izbicki, R.,
de Oliveira Duarte, Y. A., and Chiavegatto Filho, A.
D. P. (2019). Machine learning for predictive analy-
ses in health: an example of an application to predict
death in the elderly in s
˜
ao paulo, brazil. Cadernos de
saude publica, 35(7):e00050818.
Ercole, F. F., Melo, L. S. d., and Alcoforado, C. L. G. C.
(2014). Revis
˜
ao integrativa versus revis
˜
ao sistem
´
atica.
Revista Mineira de Enfermagem, 18(1):9–12.
Faraji-Biregani, M. and Nematbakhsh, N. (2019). Dia-
betes prediction recommender system based on artifi-
cial neural networks and sine-cosine optimization al-
gorithm. In 2019 5th Conference on Knowledge Based
Engineering and Innovation (KBEI), pages 263–268.
IEEE.
Goldar, S. Z., Ghiasi, A. R., Badamchizadeh, M. A., et al.
(2020). An anfis-pso algorithm for predicting four
grades of non-alcoholic fatty liver disease. In 2020
International Congress on Human-Computer Interac-
tion, Optimization and Robotic Applications (HORA),
pages 1–5. IEEE.
Grillo, M. d. F. F. and Gorini, M. I. P. C. (2007).
Caracterizac¸
˜
ao de pessoas com diabetes mellitus tipo
2. Revista Brasileira de Enfermagem, 60:49–54.
Jamuna, S. and Mohan Kumar, K. (2020). Prediction of
diabetes and clustering based on its levels using fuzzy
c means algorithm. International Journal of Scientific
and Technology Research, 9(2):3222–3225.
Keele, S. et al. (2007). Guidelines for performing system-
atic literature reviews in software engineering. Tech-
nical report, Technical report, ver. 2.3 ebse technical
report. ebse.
Lyra, R., Oliveira, M., Lins, D., and Cavalcanti, N.
(2006). Prevenc¸
˜
ao do diabetes mellitus tipo 2. Ar-
quivos Brasileiros de Endocrinologia & Metabologia,
50:239–249.
Saleck, M. M., ElMoutaouakkil, A., and Mouc¸ouf, M.
(2017). Tumor detection in mammography images us-
ing fuzzy c-means and glcm texture features. In 2017
14th International Conference on Computer Graph-
ics, Imaging and Visualization, pages 122–125.
Wedagu, M. A., Chen, D., Hussain, M. A. I., Gebremeskel,
T., Orlando, M. T., and Manzoor, A. (2020). Medicine
recommendation system for diabetes using prior med-
ical knowledge. In Proceedings of the 2020 4th In-
ternational Conference on Vision, Image and Signal
Processing, pages 1–5.
Zadeh, L. A., Klir, G. J., and Yuan, B. (1996). Fuzzy sets,
fuzzy logic, and fuzzy systems: selected papers, vol-
ume 6. World Scientific.
Fuzzy Logic for Diabetes Predictions: A Literature Review
483