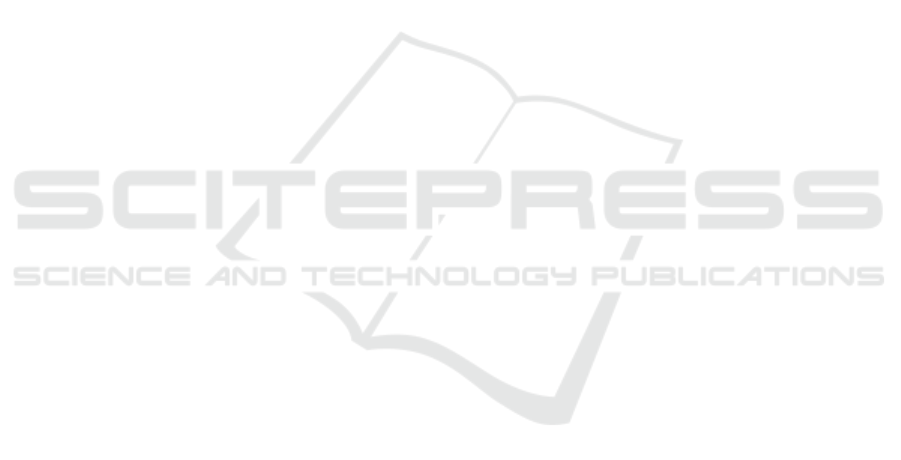
REFERENCES
Agarwal, M., Shah, R., and Mannem, P. (2011). Auto-
matic question generation using discourse cues. In
Proceedings of the Sixth Workshop on Innovative Use
of NLP for Building Educational Applications, pages
1–9, Portland, Oregon. Association for Computational
Linguistics.
Banerjee, S. and Lavie, A. (2005). METEOR: An Auto-
matic Metric for MT Evaluation with Improved Cor-
relation with Human Judgments. In Proceedings of
the ACL Workshop on Intrinsic and Extrinsic Evalu-
ation Measures for Machine Translation and/or Sum-
marization, pages 65–72, Ann Arbor, Michigan. ACL.
Callison-Burch, C., Osborne, M., and Koehn, P. (2006). Re-
evaluating the role of Bleu in machine translation re-
search. In 11th Conference of the European Chap-
ter of the Association for Computational Linguistics,
pages 249–256, Trento, Italy. Association for Compu-
tational Linguistics.
Chan, Y.-H. and Fan, Y.-C. (2019). A recurrent BERT-
based model for question generation. In Proceedings
of the 2nd Workshop on Machine Reading for Ques-
tion Answering, pages 154–162, Hong Kong, China.
Association for Computational Linguistics.
Chinkina, M., Ruiz, S., and Meurers, D. (2020). Crowd-
sourcing evaluation of the quality of automatically
generated questions for supporting computer-assisted
language teaching. ReCALL, 32(2):145–161.
Dong, L., Yang, N., Wang, W., Wei, F., Liu, X., Wang,
Y., Gao, J., Zhou, M., and Hon, H.-W. (2019). Uni-
fied Language Model Pre-training for Natural Lan-
guage Understanding and Generation. In Wallach,
H., Larochelle, H., Beygelzimer, A., Alch
´
e-Buc, F. d.,
Fox, E., and Garnett, R., editors, Advances in Neural
Information Processing Systems, volume 32. Curran
Associates, Inc.
Du, X., Shao, J., and Cardie, C. (2017). Learning to ask:
Neural question generation for reading comprehen-
sion. In Proceedings of the 55th Annual Meeting of the
Association for Computational Linguistics (Volume 1:
Long Papers), pages 1342–1352, Vancouver, Canada.
ACL.
Ferreira, J., Rodrigues, R., and Gonc¸alo Oliveira, H.
(2020). Assessing factoid question-answer genera-
tion for portuguese (short paper). In 9th Symposium
on Languages, Applications and Technologies (SLATE
2020). Schloss Dagstuhl-Leibniz-Zentrum f
¨
ur Infor-
matik.
Guo, H., Pasunuru, R., and Bansal, M. (2018). Soft layer-
specific multi-task summarization with entailment and
question generation. In Proceedings of the 56th An-
nual Meeting of the Association for Computational
Linguistics (Volume 1: Long Papers), pages 687–697,
Melbourne, Australia. Association for Computational
Linguistics.
Harrison, V. and Walker, M. (2018). Neural generation of
diverse questions using answer focus, contextual and
linguistic features. In Proceedings of the 11th Interna-
tional Conference on Natural Language Generation,
pages 296–306, Tilburg University, The Netherlands.
Association for Computational Linguistics.
He, W., Liu, K., Liu, J., Lyu, Y., Zhao, S., Xiao, X., Liu, Y.,
Wang, Y., Wu, H., She, Q., Liu, X., Wu, T., and Wang,
H. (2018). DuReader: a Chinese machine reading
comprehension dataset from real-world applications.
In Proceedings of the Workshop on Machine Read-
ing for Question Answering, pages 37–46, Melbourne,
Australia. Association for Computational Linguistics.
Heilman, M. and Smith, N. A. (2010a). Good question!
statistical ranking for question generation. In Human
Language Technologies: The 2010 Annual Confer-
ence of the North American Chapter of the Associa-
tion for Computational Linguistics, pages 609–617,
Los Angeles, California. Association for Computa-
tional Linguistics.
Heilman, M. and Smith, N. A. (2010b). Rating computer-
generated questions with Mechanical Turk. In Pro-
ceedings of the NAACL HLT 2010 Workshop on Cre-
ating Speech and Language Data with Amazon’s Me-
chanical Turk, pages 35–40, Los Angeles. Association
for Computational Linguistics.
Ji, T., Lyu, C., Jones, G., Zhou, L., and Graham, Y. (2022).
Qascore — an unsupervised unreferenced metric for
the question generation evaluation. Entropy, 24(11).
Khullar, P., Rachna, K., Hase, M., and Shrivastava, M.
(2018). Automatic question generation using rela-
tive pronouns and adverbs. In Proceedings of ACL
2018, Student Research Workshop, pages 153–158,
Melbourne, Australia. Association for Computational
Linguistics.
Kurdi, G., Leo, J., Parsia, B., Sattler, U., and Al-Emari,
S. (2020). A systematic review of automatic ques-
tion generation for educational purposes. Interna-
tional Journal of Artificial Intelligence in Education,
30(1):121–204.
Leite, B. and Lopes Cardoso, H. (2022). Neural question
generation for the portuguese language: A prelimi-
nary study. In Marreiros, G., Martins, B., Paiva, A.,
Ribeiro, B., and Sardinha, A., editors, Progress in Ar-
tificial Intelligence, pages 780–793, Cham. Springer
International Publishing.
Leite, B., Lopes Cardoso, H., Reis, L. P., and Soares, C.
(2020). Factual question generation for the portuguese
language. In 2020 International Conference on INno-
vations in Intelligent SysTems and Applications (IN-
ISTA), pages 1–7. IEEE.
Lin, C.-Y. (2004). ROUGE: A Package for Automatic
Evaluation of Summaries. In Text Summarization
Branches Out, pages 74–81, Barcelona, Spain. ACL.
Lindberg, D., Popowich, F., Nesbit, J., and Winne, P.
(2013). Generating natural language questions to sup-
port learning on-line. In Proceedings of the 14th Eu-
ropean Workshop on Natural Language Generation,
pages 105–114, Sofia, Bulgaria. Association for Com-
putational Linguistics.
Liu, C.-W., Lowe, R., Serban, I., Noseworthy, M., Charlin,
L., and Pineau, J. (2016). How NOT to evaluate your
dialogue system: An empirical study of unsupervised
evaluation metrics for dialogue response generation.
Do Rules Still Rule? Comprehensive Evaluation of a Rule-Based Question Generation System
37