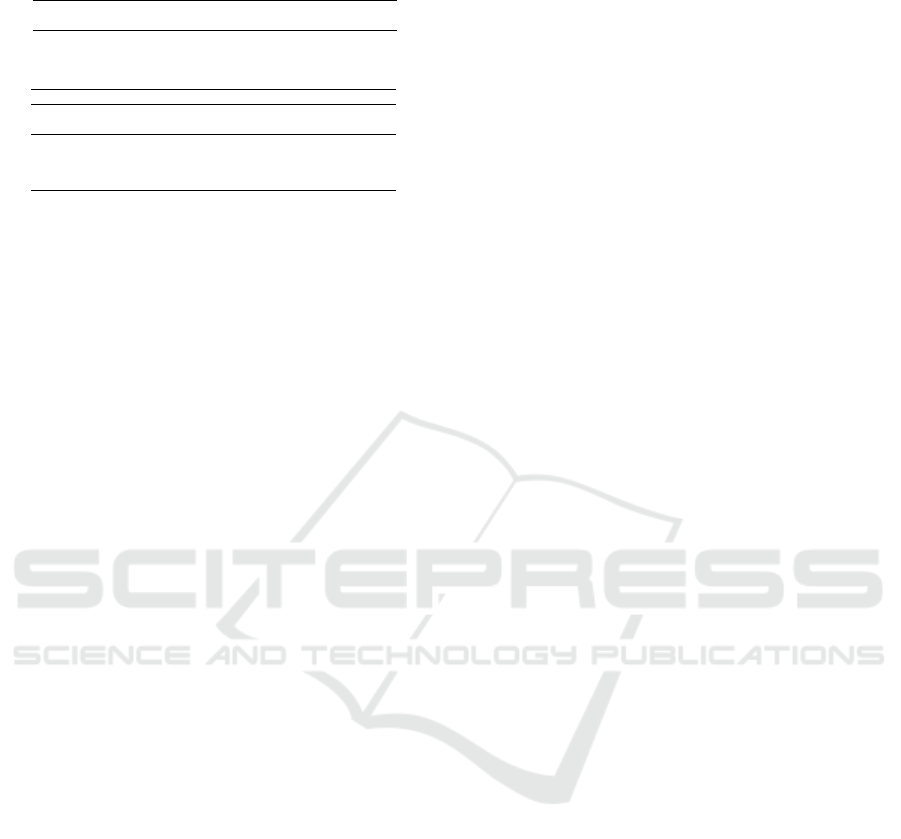
Table 4: Evaluation metrics for residence 2.
Training set residence 2
Models MSE MAPE RMSE R
2
Execution
time [sec]
ARIMA 8.20 2.86 18.32 -0.37 24
SARIMA 8.23 2.87 18.81 -0.37 24
Prophet 3.18 1.78 11.23 0.47 24
LSTM 2.67 1.63 11.11 0.53 29
Testing set residence 2
Models MSE MAPE RMSE R
2
Execution
time [sec]
ARIMA 6.90 2.63 19.02 -2.92 24
SARIMA 0.41 0.64 4.16 0.77 24
Prophet 1.09 1.05 7.19 0.38 24
LSTM 1.96 1.40 9.95 -0.26 29
available data set was not large enough. Also the data
were collected during the COVID-19 pandemic, when
the subsequent lockdown periods caused an unusual
behavior on the part of the consumers. The data were
collected over the past three years, but each year was
different. In 2019 the residents’ behavior was nor-
mal, since there was no lockdown, but in 2020 all
changed since people had to stay at home and thus the
energy consumption increased (Abu-Rayash and Din-
cer, 2020). Abnormalities like these heavily affect the
data especially in the seasonality aspect, that is crucial
for time-series data as the ones in hand. This might be
the reason of models performing worse when no ex-
ternal data are utilized, since the target variable that is
used is compromised. Moreover, all the models, ex-
cept FB Prophet performed worse with large sizes of
training data. The FB Prophet had better performance
with a larger size of training data.
Concluding, the forecasting models are part of a
software service that is accessible via a dashboard.
The service can be used by the customer to moni-
tor historical energy consumption, obtain predictions
and thus to draw conclusions providing a better under-
standing of the residence’s energy consumption, and
to possibly take actions in economizing. The service
can also be used by the electric company to acquire
sensor data from all residences. The company could
possibly “intervene” in the residence by switching off
unused smart plugs should they have the customer’s
consent; or even to suggest ways to reduce the resi-
dential energy consumption by replacing old and in-
efficient domestic appliances, i.e., oven, fridge, wash-
ing machine.
The current work can be expanded in several di-
rections. First, we can try to predict energy consump-
tion based on all 20 residences instead of only 2. Sec-
ond, as data continue to be gathered we could enhance
the training data sets. Finally, we could try different
forecasting models such as the transformer networks.
ACKNOWLEDGEMENTS
We would like to thank OTE Academy for provid-
ing the data, and the American College of Greece,
Deree for supporting the current work, as well as
Mr. Theodoros Diamantopoulos and Mr. Dimitrios
Salmatanis for their contribution to this project.
REFERENCES
Abu-Rayash, A. and Dincer, I. (2020). Analysis of the
electricity demand trends amidst the covid-19 coron-
avirus pandemic. Energy Research & Social Science,
68:101682.
Amasyali, K. and El-Gohary, N. M. (2018). A review of
data-driven building energy consumption prediction
studies. Renewable and Sustainable Energy Reviews,
81:1192–1205.
Batini, C., Scannapieco, M., et al. (2016). Data and infor-
mation quality. Cham, Switzerland: Springer Interna-
tional Publishing.
Bourdeau, M., qiang Zhai, X., Nefzaoui, E., Guo, X.,
and Chatellier, P. (2019). Modeling and forecast-
ing building energy consumption: A review of data-
driven techniques. Sustainable Cities and Society,
48:101533.
Box, G. E., Jenkins, G. M., Reinsel, G. C., and Ljung, G. M.
(2015). Time series analysis: forecasting and control.
John Wiley & Sons.
Chaturvedi, S., Rajasekar, E., Natarajan, S., and McCullen,
N. (2022). A comparative assessment of sarima, lstm
rnn and fb prophet models to forecast total and peak
monthly energy demand for india. Energy Policy,
168:113097.
Greff, K., Srivastava, R. K., Koutn
´
ık, J., Steunebrink, B. R.,
and Schmidhuber, J. (2016). Lstm: A search space
odyssey. IEEE transactions on neural networks and
learning systems, 28(10):2222–2232.
Hochreiter, S. and Schmidhuber, J. (1997). Long short-term
memory. Neural computation, 9(8):1735–1780.
Miller, C., Picchetti, B., Fu, C., and Pantelic, J. (2022).
Limitations of machine learning for building energy
prediction: Ashrae great energy predictor iii kaggle
competition error analysis. Science and Technology
for the Built Environment, pages 1–18.
Ozili, P. K. and Ozen, E. (2021). Global energy crisis: im-
pact on the global economy. In Proceedings of IAC in
Budapest 2021, volume 1, pages 85–89. Czech Insti-
tute of Academic Education.
Seel, J., Mills, A. D., and Wiser, R. H. (2018). Impacts of
high variable renewable energy futures on wholesale
electricity prices, and on electric-sector decision mak-
ing. Lawrence Berkeley National Laboratory (May
2018).
Shumway, R. H., Stoffer, D. S., and Stoffer, D. S. (2000).
Time series analysis and its applications, volume 3.
Springer.
Forecasting Residential Energy Consumption: A Case Study for Greece
491