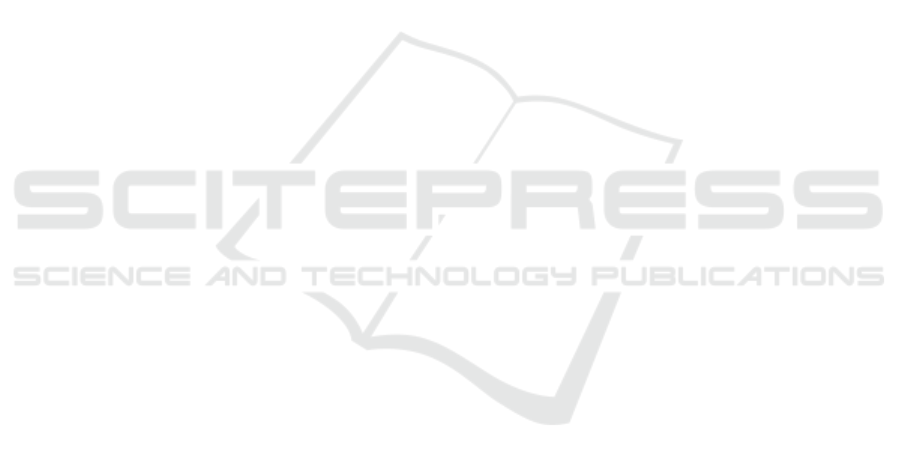
ditions. Developing all of these additional ideas re-
quires time and effort, but the results obtained could
unravel the reason for GAIL’s failure to learn obsta-
cle avoidance in scenarios as S4, as well as enrich the
knowledge of each strategy’s capabilities and applica-
bility.
ACKNOWLEDGEMENTS
The authors would like to thank the National Coun-
cil for Scientific and Technological Development
(CNPq) (421548/2022-3 and 88881.753469/2022-01)
and Araucaria Foundation (17.633.124-0) for their fi-
nancial support on this work. We would also like to
thank the Manna Team for support, learning and col-
laboration of its members.
REFERENCES
Antonelo, E. A. and Schrauwen, B. (2010). Supervised
learning of internal models for autonomous goal-
oriented robot navigation using reservoir computing.
In 2010 IEEE International Conference on Robotics
and Automation, pages 2959–2964. IEEE.
Braitenberg, V. (1986). Vehicles: Experiments in synthetic
psychology. MIT press.
Brockman, G., Cheung, V., Pettersson, L., Schneider, J.,
Schulman, J., Tang, J., and Zaremba, W. (2016). Ope-
nai gym.
Couto, G. C. K. and Antonelo, E. A. (2021). Genera-
tive adversarial imitation learning for end-to-end au-
tonomous driving on urban environments. In 2021
IEEE Symposium Series on Computational Intelli-
gence (SSCI), pages 1–7. IEEE.
Fahad, M., Yang, G., and Guo, Y. (2020). Learning human
navigation behavior using measured human trajecto-
ries in crowded spaces. In 2020 IEEE/RSJ Interna-
tional Conference on Intelligent Robots and Systems
(IROS), pages 11154–11160. IEEE.
Goodfellow, I., Pouget-Abadie, J., Mirza, M., Xu, B.,
Warde-Farley, D., Ozair, S., Courville, A., and Ben-
gio, Y. (2014). Generative adversarial nets. In
Advances in neural information processing systems,
pages 2672–2680.
Ho, J. and Ermon, S. (2016). Generative adversarial im-
itation learning. In Advances in neural information
processing systems, pages 4565–4573.
Kuefler, A., Morton, J., Wheeler, T., and Kochenderfer, M.
(2017). Imitating driver behavior with generative ad-
versarial networks. In 2017 IEEE Intelligent Vehicles
Symposium (IV), pages 204–211. IEEE.
Mao, Y., Gao, F., Zhang, Q., and Yang, Z. (2022). An auv
target-tracking method combining imitation learning
and deep reinforcement learning. Journal of Marine
Science and Engineering, 10(3):383.
Pham, D.-T., Tran, T.-N., Alam, S., and Duong, V. N.
(2021). A generative adversarial imitation learning ap-
proach for realistic aircraft taxi-speed modeling. IEEE
Transactions on Intelligent Transportation Systems,
23(3):2509–2522.
Pore, A., Tagliabue, E., Piccinelli, M., Dall’Alba, D.,
Casals, A., and Fiorini, P. (2021). Learning from
demonstrations for autonomous soft-tissue retrac-
tion. In 2021 International Symposium on Medical
Robotics (ISMR), pages 1–7. IEEE.
Rohmer, E., Singh, S. P. N., and Freese, M. (2013). Cop-
peliasim (formerly v-rep): a versatile and scalable
robot simulation framework. In Proc. of The Interna-
tional Conference on Intelligent Robots and Systems
(IROS).
Schulman, J., Levine, S., Abbeel, P., Jordan, M., and
Moritz, P. (2015). Trust region policy optimization. In
International conference on machine learning, pages
1889–1897. PMLR.
Schulman, J., Wolski, F., Dhariwal, P., Radford, A., and
Klimov, O. (2017). Proximal policy optimization al-
gorithms. arXiv preprint arXiv:1707.06347.
Sheh, R., Hengst, B., and Sammut, C. (2011). Behavioural
cloning for driving robots over rough terrain. In
2011 IEEE/RSJ International Conference on Intelli-
gent Robots and Systems, pages 732–737. IEEE.
Wang, C., P
´
erez-D’Arpino, C., Xu, D., Fei-Fei, L., Liu, K.,
and Savarese, S. (2022). Co-gail: Learning diverse
strategies for human-robot collaboration. In Confer-
ence on Robot Learning, pages 1279–1290. PMLR.
Wang, S., Toyer, S., Gleave, A., and Emmons, S. (2020).
The imitation library for imitation learning and
inverse reinforcement learning. https://github.com/
HumanCompatibleAI/imitation.
Xiao, X., Liu, B., Warnell, G., and Stone, P. (2022). Motion
planning and control for mobile robot navigation us-
ing machine learning: a survey. Autonomous Robots,
pages 1–29.
Zhou, Y., Fu, R., Wang, C., and Zhang, R. (2020). Mod-
eling car-following behaviors and driving styles with
generative adversarial imitation learning. Sensors,
20(18):5034.
Zolna, K., Reed, S., Novikov, A., Colmenarejo, S. G., Bud-
den, D., Cabi, S., Denil, M., de Freitas, N., and Wang,
Z. (2019). Task-relevant adversarial imitation learn-
ing. arXiv preprint arXiv:1910.01077.
Zuo, G., Chen, K., Lu, J., and Huang, X. (2020). Determin-
istic generative adversarial imitation learning. Neuro-
computing, 388:60–69.
ICEIS 2023 - 25th International Conference on Enterprise Information Systems
524