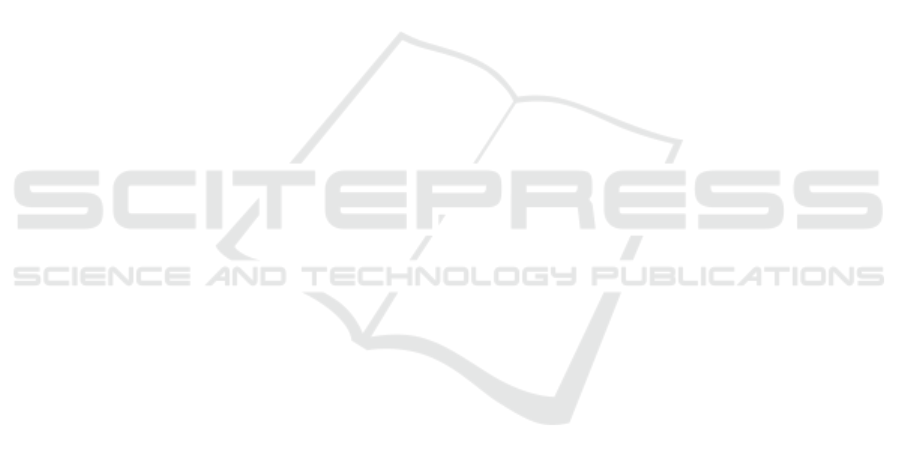
The present study has not yet clarified the most
reasonable way in generating attention map. Re-
sults shown in Figure 5 implies that External Atten-
tion sometimes emphasizes noises. Such excessive
attentions could inhibit training progresses of ADN.
So we need to establish some methodologies for ad-
justing the strength of external attention at each at-
tention point. One simple idea is to introduce spar-
sity assumptions on attention map. At the same time,
the effectiveness of Layer-wise External Attention
most likely depends on the quality of anomaly map.
In experiments that we conducted in this study, we
use CAAN to generate anomaly map for all datasets.
However, for more practical use, it is reasonable to
choose the most appropriate Anomaly Attention Net-
work for each dataset and task. The LEA-Net sys-
tem was originally designed to appropriately replace
Anomaly Attention Network to suit for the target do-
main. In this regard, we can say that Layer-wise Ex-
ternal Attention is more versatile system in compari-
son with Self-Attention.
REFERENCES
Akcay, S., Atapour-Abarghouei, A., and Breckon, T. P.
(2018). Ganomaly: semi-supervised anomaly detec-
tion via adversarial training.
ashok (2020). Cloud and non-cloud images (anomaly
detection). https://www.kaggle.com/ashoksrinivas/
cloud-anomaly-detection-images. Accessed: 2020-
08-22.
Bergmann, P., Fauser, M., Sattlegger, D., and Steger, C.
(2019). MVTec AD — A Comprehensive Real-World
Dataset for Unsupervised Anomaly Detection. In
2019 IEEE/CVF Conference on Computer Vision and
Pattern Recognition (CVPR).
Cao, C., Liu, F., Tan, H., Song, D., Shu, W., Li, W., Zhou,
Y., Bo, X., and Xie, Z. (2018). Deep learning and its
applications in biomedicine. Genomics, proteomics &
bioinformatics, 16(1):17–32.
Chun, M. M., Golomb, J. D., and Turk-Browne, N. B.
(2011). A taxonomy of external and internal attention.
Annual review of psychology, 62:73–101.
Emami, H., Aliabadi, M. M., Dong, M., and Chinnam, R. B.
(2020). Spa-gan: Spatial attention gan for image-to-
image translation. IEEE Transactions on Multimedia,
23:391–401.
Ferentinos, K. P. (2018). Deep learning models for plant
disease detection and diagnosis. Computers and Elec-
tronics in Agriculture, 145:311–318.
Fukui, H., Hirakawa, T., Yamashita, T., and Fujiyoshi, H.
(2019). Attention branch network: Learning of atten-
tion mechanism for visual explanation.
Haselmann, M., Gruber, D. P., and Tabatabai, P. (2018).
Anomaly detection using deep learning based image
completion.
He, K., Zhang, X., Ren, S., and Sun, J. (2016). Deep resid-
ual learning for image recognition. In Proceedings of
the IEEE conference on computer vision and pattern
recognition, pages 770–778.
Hinton, G., Vinyals, O., and Dean, J. (2015). Distilling the
knowledge in a neural network.
Howard, A., Sandler, M., Chu, G., Chen, L.-C., Chen, B.,
Tan, M., Wang, W., Zhu, Y., Pang, R., Vasudevan, V.,
Le, Q. V., and Adam, H. (2019). Searching for mo-
bilenetv3.
Hu, J., Shen, L., Albanie, S., Sun, G., and Wu, E. (2020a).
Squeeze-and-Excitation Networks. IEEE Transac-
tions on Pattern Analysis and Machine Intelligence,
42(8):2011–2023.
Hu, J., Shen, L., Albanie, S., Sun, G., and Wu, E. (2020b).
Squeeze-and-excitation networks. IEEE Transac-
tions on Pattern Analysis and Machine Intelligence,
42(8):2011–2023.
Hughes, D. P. and Salathe, M. (2016). An open access
repository of images on plant health to enable the de-
velopment of mobile disease diagnostics.
Hyunjae, L., Hyo Eun, K., and Hyeonseob, N. (2019). Srm:
A style-based re- calibration module for convolutional
neural networks. In Proceedings of the IEEE Interna-
tional Conference on Computer Vision.
Katafuchi, R. and Tokunaga, T. (2021). Image-based Plant
Disease Diagnosis with Unsupervised Anomaly De-
tection based on Reconstructability of Colors. pages
112–120.
Krizhevsky, A., Sutskever, I., and Hinton, G. E. (2012). Im-
agenet classification with deep convolutional neural
networks. Advances in neural information processing
systems, 25:1097–1105.
Lee, H., Kim, H. E., and Nam, H. (2019). SRM: A style-
based recalibration module for convolutional neural
networks. Proceedings of the IEEE International Con-
ference on Computer Vision, 2019-Octob:1854–1862.
Li, W., Cheng, S., Qian, K., Yue, K., and Liu, H. (2021).
Automatic Recognition and Classification System of
Thyroid Nodules in CT Images Based on CNN. Com-
putational Intelligence and Neuroscience, 2021.
Lu, L. (2020). Dying relu and initialization: Theory and nu-
merical examples. Communications in Computational
Physics, 28(5):1671–1706.
Minhas, M. S. and Zelek, J. (2019). Anomaly detection in
images. arXiv preprint arXiv:1905.13147.
Natarajan, V., Mao, S., and Chia, L.-T. (2019). Salient tex-
tural anomaly proposals and classification for metal
surface anomalies. In 2019 IEEE 31st International
Conference on Tools with Artificial Intelligence (IC-
TAI), pages 621–628.
Nico Goernitz, Marius Micha Kloft, K. R. U. B. (2013).
Toward supervised anomaly detection. Journal Of Ar-
tificial Intelligence Research (JAIR), 46:235–262.
Pires, R., Jelinek, H. F., Wainer, J., Valle, E., and Rocha, A.
(2016). Advancing Bag-of-Visual-Words Representa-
tions for Lesion Classification in Retinal Images.
Reynolds, J. H. and Chelazzi, L. (2004). Attentional mod-
ulation of visual processing. Annu. Rev. Neurosci.,
27:611–647.
Layer-wise External Attention for Efficient Deep Anomaly Detection
109