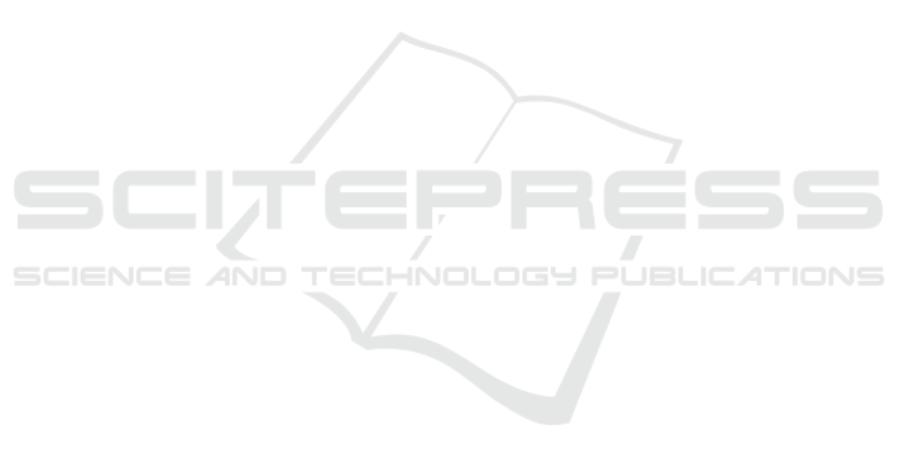
REFERENCES
Achieve, Inc (2013). Next Generation Science Standards.
Britt, M. A., Rouet, J. F., and Durik, A. M. (2017). Literacy
Beyond Text Comprehension: A Theory of Purposeful
Reading. Routledge, New York.
Brown, T. B., Mann, B., Ryder, N., Subbiah, M., Kaplan,
J., Dhariwal, P., Neelakantan, A., Shyam, P., Sastry,
G., Askell, A., et al. (2020). Language models are
few-shot learners. arXiv preprint arXiv:2005.14165.
Casa
˜
n, R. R., Garc
´
ıa-Vidal, E., Grimaldi, D., Carrasco-
Farr
´
e, C., Vaquer-Estalrich, F., and Vila-Franc
´
es, J.
(2022). Online polarization and cross-fertilization in
multi-cleavage societies: the case of spain. Social Net-
work Analysis and Mining, 12(1):1–17.
Chandrasekaran, D. and Mago, V. (2021). Evolution of se-
mantic similarity—a survey. ACM Computing Surveys
(CSUR), 54(2):1–37.
Chen, J., Tam, D., Raffel, C., Bansal, M., and Yang,
D. (2021). An empirical survey of data augmenta-
tion for limited data learning in nlp. arXiv preprint
arXiv:2106.07499.
Cochran, K., Cohn, C., Hutchins, N., Biswas, G., and Hast-
ings, P. (2022). Improving automated evaluation of
formative assessments with text data augmentation. In
International Conference on Artificial Intelligence in
Education, pages 390–401. Springer.
Cohn, C. (2020). BERT Efficacy on Scientific and Medi-
cal Datasets: A Systematic Literature Review. DePaul
University.
Devlin, J., Chang, M.-W., Lee, K., and Toutanova, K.
(2018). BERT: Pre-training of deep bidirectional
transformers for language understanding. arXiv
preprint arXiv:1810.04805.
Hastings, P., Hughes, S., Britt, A., Blaum, D., and Wal-
lace, P. (2014). Toward automatic inference of causal
structure in student essays. In International Confer-
ence on Intelligent Tutoring Systems, pages 266–271.
Springer.
Hughes, S. (2019). Automatic Inference of Causal Reason-
ing Chains from Student Essays. PhD thesis, DePaul
University, Chicago.
Litschko, R., Vuli
´
c, I., Ponzetto, S. P., and Glava
ˇ
s, G.
(2022). On cross-lingual retrieval with multilin-
gual text encoders. Information Retrieval Journal,
25(2):149–183.
Neyman, J. (1992). On the two different aspects of the rep-
resentative method: the method of stratified sampling
and the method of purposive selection. Springer.
Nguyen, K. H., Dinh, D. C., Le, H. T.-T., and Dinh, D.
(2022). English-vietnamese cross-lingual semantic
textual similarity using sentence transformer model.
In 2022 14th International Conference on Knowledge
and Systems Engineering (KSE), pages 1–5. IEEE.
Nugumanova, A., Baiburin, Y., and Alimzhanov, Y. (2022).
Sentiment analysis of reviews in kazakh with trans-
fer learning techniques. In 2022 International Confer-
ence on Smart Information Systems and Technologies
(SIST), pages 1–6. IEEE.
OECD (2021). 21st-Century Readers. PISA, OECD
Publishing. https://www.oecd-ilibrary.org/content/
publication/a83d84cb-en.
Reimers, N. and Gurevych, I. (2020). Making monolin-
gual sentence embeddings multilingual using knowl-
edge distillation. In Proceedings of the 2020 Confer-
ence on Empirical Methods in Natural Language Pro-
cessing. Association for Computational Linguistics.
S¸as¸maz, E. and Tek, F. B. (2021). Tweet sentiment anal-
ysis for cryptocurrencies. In 2021 6th International
Conference on Computer Science and Engineering
(UBMK), pages 613–618. IEEE.
Schumann, G., Meyer, K., and Gomez, J. M. (2022). Query-
based retrieval of german regulatory documents for in-
ternal auditing purposes. In 2022 5th International
Conference on Data Science and Information Tech-
nology (DSIT), pages 01–10. IEEE.
Seo, J.-W., Jung, H.-G., and Lee, S.-W. (2021). Self-
augmentation: Generalizing deep networks to un-
seen classes for few-shot learning. Neural Networks,
138:140–149.
Shorten, C. and Khoshgoftaar, T. M. (2019). A survey on
image data augmentation for deep learning. Journal
of big data, 6(1):1–48.
Verma, A., Walbe, S., Wani, I., Wankhede, R., Thakare,
R., and Patankar, S. (2022). Sentiment analysis us-
ing transformer based pre-trained models for the hindi
language. In 2022 IEEE International Students’ Con-
ference on Electrical, Electronics and Computer Sci-
ence (SCEECS), pages 1–6. IEEE.
Vikraman, L. N. (2022). Answer similarity grouping and di-
versification in question answering systems. Doctoral
Dissertation.
Wang, W., Wei, F., Dong, L., Bao, H., Yang, N., and Zhou,
M. (2020). Minilm: Deep self-attention distillation for
task-agnostic compression of pre-trained transform-
ers.
Wei, J. and Zou, K. (2019). EDA: Easy data augmentation
techniques for boosting performance on text classifi-
cation tasks. arXiv preprint arXiv:1901.11196.
Wu, L., Xie, P., Zhou, J., Zhang, M., Ma, C., Xu, G., and
Zhang, M. (2022). Self-augmentation for named en-
tity recognition with meta reweighting. arXiv preprint
arXiv:2204.11406.
Xu, F., Kurz, D., Piskorski, J., and Schmeier, S. (2002). A
domain adaptive approach to automatic acquisition of
domain relevant terms and their relations with boot-
strapping. In LREC.
Zhang, N., Biswas, G., McElhaney, K. W., Basu, S.,
McBride, E., and Chiu, J. L. (2020). Studying the in-
teractions between science, engineering, and compu-
tational thinking in a learning-by-modeling environ-
ment. In International Conference on Artificial Intel-
ligence in Education, pages 598–609. Springer.
CSEDU 2023 - 15th International Conference on Computer Supported Education
78