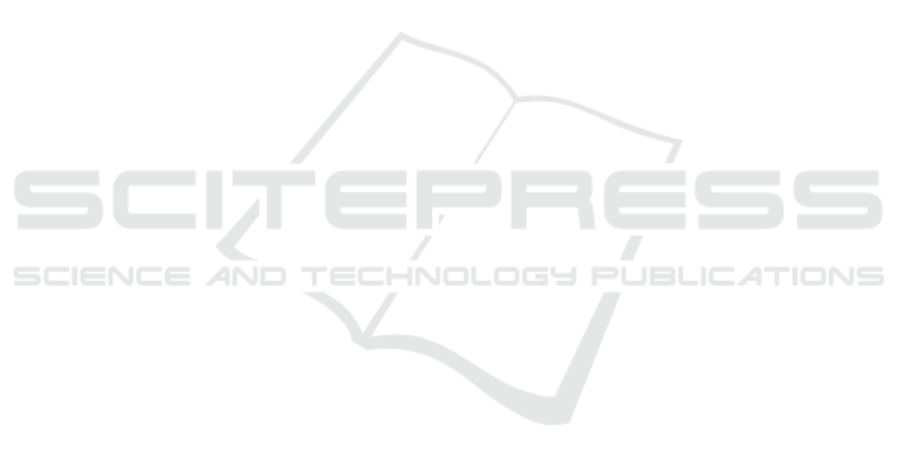
erage 10.6 ms, Figure 4b) is lower than cloud-based
DT (average 18.8 ms, Figure 4a). Security is impor-
tant to provide secure and reliable services to users,
so it is a metric we should consider in the systems
we develop (Asim et al., 2020). Edge-based systems
are more secure than cloud-based systems because of
their decentralized architecture, whereas cloud-based
systems are more vulnerable to attacks because they
transmit long distances between users and the cloud
(Asim et al., 2020).
6 CONCLUSION
The concept of Industry 4.0, which combines the do-
mains of Informatics and Industry, has spread from
the industrial sector to all other sectors. IoT, 5G and
6G networks, cloud and edge computing, big data, AI
and DT technologies are at the center of these devel-
opments.
In this paper, we discussed the comparison of
cloud-based DT and edge-based DT via a case study.
In this study, we built ML models on PTBD health-
care dataset to predict human heart diseases in real
time and thus to apply quick treatments. In this
context, we performed preprocessing stages such as
cleaning the signal data, denoising, smoothing, peak
extraction, eliminate the NaN values, imputer for
missing values and feature engineering stages such
as feature binning, feature selection, sampling. Even
though in Cardio Twin (Martinez-Velazquez et al.,
2019) paper was obtained the highest precision rate,
we tuning it based on the recall metric because TP
value is more vital in detecting diseases in the health
field. Thus, we outperformed better in terms of recall,
F-score and accuracy.
A major future step of this study is to apply a so-
lution to the data security and privacy concern, which
is frequently encountered in health studies, by com-
bining cloud computing, edge computing, federated
learning and DT technologies. In addition, our future
work also will include on trying different ML mod-
els with the new feature dataset containing the clini-
cal findings of the patients and validating models with
different health dataset. Additionally, a case study in-
cludes data which is collected from sensors can be
added for the real world usage experiment.
REFERENCES
Asim, M., Wang, Y., Wang, K., and Huang, P.-Q. (2020).
A review on computational intelligence techniques
in cloud and edge computing. IEEE Transactions
on Emerging Topics in Computational Intelligence,
4(6):742–763.
Bellavista, P., Giannelli, C., Mamei, M., Mendula, M., and
Picone, M. (2021). Application-driven network-aware
digital twin management in industrial edge environ-
ments. IEEE Transactions on Industrial Informatics,
17(11):7791–7801.
Bj
¨
ornsson, B., Borrebaeck, C., Elander, N., Gasslander, T.,
Gawel, D. R., Gustafsson, M., J
¨
ornsten, R., Lee, E. J.,
Li, X., Lilja, S., et al. (2020). Digital twins to person-
alize medicine. Genome medicine, 12(1):1–4.
Bousseljot, R., Kreiseler, D., and Schnabel, A. (1995).
Nutzung der ekg-signaldatenbank cardiodat der ptb
¨
uber das internet.
Dritsas., E., Alexiou., S., and Moustakas., K. (2022).
Cardiovascular disease risk prediction with super-
vised machine learning techniques. In Proceedings
of the 8th International Conference on Information
and Communication Technologies for Ageing Well
and e-Health - ICT4AWE,, pages 315–321. INSTICC,
SciTePress.
Friedman, J. H. (2001). Greedy function approximation: a
gradient boosting machine. Annals of statistics, pages
1189–1232.
Fuller, A., Fan, Z., Day, C., and Barlow, C. (2020). Digi-
tal twin: Enabling technologies, challenges and open
research. IEEE access, 8:108952–108971.
Ge, X., Zhou, R., and Li, Q. (2019). 5g nfv-based tactile
internet for mission-critical iot services. IEEE Internet
of Things Journal, 7(7):6150–6163.
Githens, G. (2007). Product lifecycle management: driv-
ing the next generation of lean thinking by michael
grieves.
Glatt, M., K
¨
olsch, P., Siedler, C., Langlotz, P., Ehmsen, S.,
and Aurich, J. C. (2021). Edge-based digital twin to
trace and ensure sustainability in cross-company pro-
duction networks. Procedia CIRP, 98:276–281.
Goldberger, A. L., Amaral, L. A., Glass, L., Hausdorff,
J. M., Ivanov, P. C., Mark, R. G., Mietus, J. E., Moody,
G. B., Peng, C.-K., and Stanley, H. E. (2000). Phys-
iobank, physiotoolkit, and physionet: components of
a new research resource for complex physiologic sig-
nals. circulation, 101(23):e215–e220.
Grieves, M. (2002). Plm initiatives [powerpoint slides]. In
Product Lifecycle Management Special Meeting.
Grieves, M. W. (2005). Product lifecycle management: the
new paradigm for enterprises. International Journal
of Product Development, 2(1-2):71–84.
Grieves, M. W. (2019). Virtually intelligent product sys-
tems: digital and physical twins.
Halepmollası, R., Zeybel, M., Eyvaz, E., Arkan, R., Genc,
A., Bilgen, I., and Haklidir, M. (2021). Towards fed-
erated learning in identification of medical images: A
case study. Artificial Intelligence Theory and Appli-
cation, Volume: 1:30–39.
Hassani, H., Huang, X., and MacFeely, S. (2022). Impactful
digital twin in the healthcare revolution. Big Data and
Cognitive Computing, 6(3):83.
Huang., J., Chang., L., and Lin., H. (2021). Implementation
of iot, wearable devices, google assistant and google
ICT4AWE 2023 - 9th International Conference on Information and Communication Technologies for Ageing Well and e-Health
168