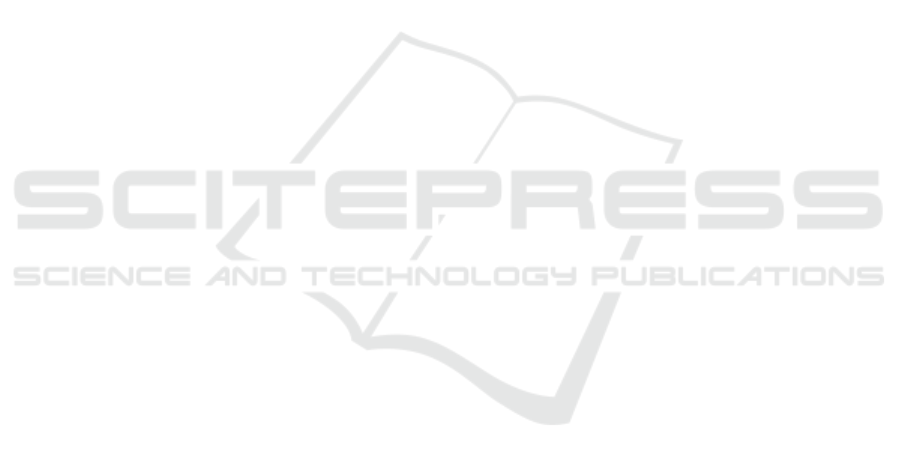
conference on computer vision and pattern recogni-
tion, pages 7482–7491.
Li, C., Yan, J., Wei, F., Dong, W., Liu, Q., and Zha, H.
(2017). Self-paced multi-task learning. In Thirty-First
AAAI Conference on Artificial Intelligence.
Li, C.-L., Sohn, K., Yoon, J., and Pfister, T. (2021). Cut-
paste: Self-supervised learning for anomaly detection
and localization. In Proceedings of the IEEE/CVF
Conference on Computer Vision and Pattern Recog-
nition, pages 9664–9674.
Lin, A., Chen, B., Xu, J., Zhang, Z., Lu, G., and Zhang,
D. (2022). Ds-transunet: Dual swin transformer u-net
for medical image segmentation. IEEE Transactions
on Instrumentation and Measurement.
Liu, Z., Lin, Y., Cao, Y., Hu, H., Wei, Y., Zhang, Z., Lin,
S., and Guo, B. (2021). Swin transformer: Hierarchi-
cal vision transformer using shifted windows. arXiv
preprint arXiv:2103.14030.
Mishra, P., Verk, R., Fornasier, D., Piciarelli, C., and
Foresti, G. L. (2021). Vt-adl: A vision transformer
network for image anomaly detection and localization.
arXiv preprint arXiv:2104.10036.
Pan, X., Ge, C., Lu, R., Song, S., Chen, G., Huang, Z., and
Huang, G. (2022). On the integration of self-attention
and convolution. In Proceedings of the IEEE/CVF
Conference on Computer Vision and Pattern Recog-
nition, pages 815–825.
Pirnay, J. and Chai, K. (2021). Inpainting transformer for
anomaly detection. arXiv preprint arXiv:2104.13897.
Quiros, A. C., Coudray, N., Yeaton, A., Yang, X., Chiri-
boga, L., Karimkhan, A., Narula, N., Pass, H., Mor-
eira, A. L., Quesne, J. L., Tsirigos, A., and Yuan,
K. (2022). Self-supervised learning in non-small cell
lung cancer discovers novel morphological clusters
linked to patient outcome and molecular phenotypes.
Ramachandran, P., Parmar, N., Vaswani, A., Bello, I.,
Levskaya, A., and Shlens, J. (2019). Stand-alone
self-attention in vision models. arXiv preprint
arXiv:1906.05909.
Roth, K., Pemula, L., Zepeda, J., Sch
¨
olkopf, B., Brox,
T., and Gehler, P. (2022). Towards total recall in
industrial anomaly detection. In Proceedings of the
IEEE/CVF Conference on Computer Vision and Pat-
tern Recognition, pages 14318–14328.
Rudolph, M., Wandt, B., and Rosenhahn, B. (2021). Same
same but differnet: Semi-supervised defect detec-
tion with normalizing flows. In Proceedings of
the IEEE/CVF Winter Conference on Applications of
Computer Vision, pages 1907–1916.
Shi, Y., Yang, J., and Qi, Z. (2021). Unsupervised anomaly
segmentation via deep feature reconstruction. Neuro-
computing, 424:9–22.
Simonyan, K. and Zisserman, A. (2014). Very deep con-
volutional networks for large-scale image recognition.
arXiv preprint arXiv:1409.1556.
Touvron, H., Cord, M., Douze, M., Massa, F., Sablayrolles,
A., and J
´
egou, H. (2021). Training data-efficient
image transformers & distillation through attention.
In International Conference on Machine Learning,
pages 10347–10357. PMLR.
Vaswani, A., Ramachandran, P., Srinivas, A., Parmar, N.,
Hechtman, B., and Shlens, J. (2021). Scaling lo-
cal self-attention for parameter efficient visual back-
bones. In Proceedings of the IEEE/CVF Conference
on Computer Vision and Pattern Recognition, pages
12894–12904.
Vaswani, A., Shazeer, N., Parmar, N., Uszkoreit, J., Jones,
L., Gomez, A. N., Kaiser, Ł., and Polosukhin, I.
(2017). Attention is all you need. In Advances in
neural information processing systems, pages 5998–
6008.
Wang, W., Xie, E., Li, X., Fan, D.-P., Song, K., Liang, D.,
Lu, T., Luo, P., and Shao, L. (2021). Pyramid vi-
sion transformer: A versatile backbone for dense pre-
diction without convolutions. In Proceedings of the
IEEE/CVF International Conference on Computer Vi-
sion, pages 568–578.
Yang, Y. (2021). Self-attention autoencoder for anomaly
segmentation.
Zagoruyko, S. and Komodakis, N. (2016). Wide residual
networks. arXiv preprint arXiv:1605.07146.
Zavrtanik, V., Kristan, M., and Sko caj, D. (2021). Recon-
struction by inpainting for visual anomaly detection.
Pattern Recognition, 112:107706.
Zhang, Y., Gong, Y., Zhu, H., Bai, X., and Tang, W. (2020).
Multi-head enhanced self-attention network for nov-
elty detection. Pattern Recognition, 107:107486.
Zhao, Y., Wang, G., Tang, C., Luo, C., Zeng, W., and Zha,
Z.-J. (2021). A battle of network structures: An empir-
ical study of cnn, transformer, and mlp. arXiv preprint
arXiv:2108.13002.
HaloAE: A Local Transformer Auto-Encoder for Anomaly Detection and Localization Based on HaloNet
335