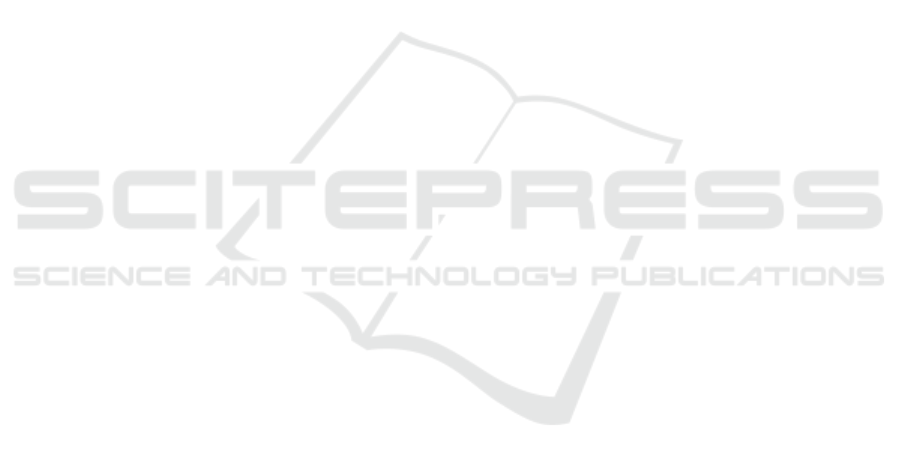
Ben-Sasson, E., Chiesa, A., Genkin, D., Tromer, E., and
Virza, M. (2013). Snarks for C: verifying program
executions succinctly and in zero knowledge. In
CRYPTO (2), volume 8043 of Lecture Notes in Com-
puter Science, pages 90–108. Springer.
Bitansky, N., Canetti, R., Chiesa, A., and Tromer, E. (2012).
From extractable collision resistance to succinct non-
interactive arguments of knowledge, and back again.
In Innovations in Theoretical Computer Science 2012,
pages 326–349.
Blum, M. (1981). Coin flipping by telephone. In CRYPTO,
pages 11–15. U. C. Santa Barbara, Dept. of Elec. and
Computer Eng., ECE Report No 82-04.
Costello, C., Fournet, C., Howell, J., Kohlweiss, M.,
Kreuter, B., Naehrig, M., Parno, B., and Zahur, S.
(2015). Geppetto: Versatile verifiable computation.
In IEEE Symposium on Security and Privacy, pages
253–270. IEEE Computer Society.
Fischlin, M. (2001). Trapdoor commitment schemes and
their applications. PhD thesis, Goethe University
Frankfurt, Frankfurt am Main, Germany.
Gennaro, R., Gentry, C., Parno, B., and Raykova, M.
(2013). Quadratic span programs and succinct nizks
without pcps. In EUROCRYPT, volume 7881 of
Lecture Notes in Computer Science, pages 626–645.
Springer.
Groth, J. (2010). Short pairing-based non-interactive zero-
knowledge arguments. In ASIACRYPT, volume 6477
of Lecture Notes in Computer Science, pages 321–
340. Springer.
Groth, J. (2016). On the size of pairing-based non-
interactive arguments. In EUROCRYPT (2), volume
9666 of Lecture Notes in Computer Science, pages
305–326. Springer.
Groth, J. and Maller, M. (2017). Snarky signatures:
Minimal signatures of knowledge from simulation-
extractable snarks. In CRYPTO (2), volume 10402 of
Lecture Notes in Computer Science, pages 581–612.
Springer.
He, K., Zhang, X., Ren, S., and Sun, J. (2016). Deep resid-
ual learning for image recognition. In CVPR, pages
770–778. IEEE Computer Society.
Hidano, S., Ohki, T., and Takahashi, K. (2012). Evaluation
of security for biometric guessing attacks in biomet-
ric cryptosystem using fuzzy commitment scheme. In
BIOSIG, volume P-196 of LNI, pages 1–6. GI.
Jain, A. K., Ross, A., and Pankanti, S. (2006). Biomet-
rics: a tool for information security. IEEE Trans. Inf.
Forensics Secur., 1(2):125–143.
Juels, A. and Wattenberg, M. (1999). A fuzzy commitment
scheme. In CCS, pages 28–36. ACM.
Kawachi, A., Tanaka, K., and Xagawa, K. (2008). Con-
currently secure identification schemes based on the
worst-case hardness of lattice problems. In ASI-
ACRYPT, volume 5350 of Lecture Notes in Computer
Science, pages 372–389. Springer.
Keller, D., Osadchy, M., and Dunkelman, O. (2020). Fuzzy
commitments offer insufficient protection to biomet-
ric templates produced by deep learning. CoRR,
abs/2012.13293.
Kosba, A. E., Zhao, Z., Miller, A., Qian, Y., Chan, T. H.,
Papamanthou, C., Pass, R., Shelat, A., and Shi, E.
(2015). How to use snarks in universally composable
protocols. IACR Cryptol. ePrint Arch., 2015:1093.
Marcel, S., Nixon, M. S., Fi
´
errez, J., and Evans, N. W. D.,
editors (2019). Handbook of Biometric Anti-Spoofing
- Presentation Attack Detection, Second Edition. Ad-
vances in Computer Vision and Pattern Recognition.
Springer.
Parno, B., Howell, J., Gentry, C., and Raykova, M. (2013).
Pinocchio: Nearly practical verifiable computation. In
IEEE Symposium on Security and Privacy, pages 238–
252. IEEE Computer Society.
Rathgeb, C. and Uhl, A. (2012). Statistical attack against
fuzzy commitment scheme. IET Biom., 1(2):94–104.
Schroff, F., Kalenichenko, D., and Philbin, J. (2015).
Facenet: A unified embedding for face recognition
and clustering. In CVPR, pages 815–823. IEEE Com-
puter Society.
Simoens, K., Bringer, J., Chabanne, H., and Seys, S. (2012).
A framework for analyzing template security and pri-
vacy in biometric authentication systems. IEEE Trans.
Inf. Forensics Secur., 7(2):833–841.
Tuyls, P., Skoric, B., and Kevenaar, T., editors (2007). Secu-
rity with noisy data : on private biometrics, secure key
storage and anti-counterfeiting. Springer, Germany.
Wahby, R. S., Setty, S. T. V., Ren, Z., Blumberg, A. J., and
Walfish, M. (2015). Efficient RAM and control flow
in verifiable outsourced computation. In NDSS. The
Internet Society.
Wang, M. and Deng, W. (2021). Deep face recognition: A
survey. Neurocomputing, 429:215–244.
Wu, X., He, R., Sun, Z., and Tan, T. (2018). A light CNN
for deep face representation with noisy labels. IEEE
Trans. Inf. Forensics Secur., 13(11):2884–2896.
Zinkus, M., Jois, T. M., and Green, M. (2022). Sok: Cryp-
tographic confidentiality of data on mobile devices.
Proc. Priv. Enhancing Technol., 2022(1):586–607.
ICISSP 2023 - 9th International Conference on Information Systems Security and Privacy
756