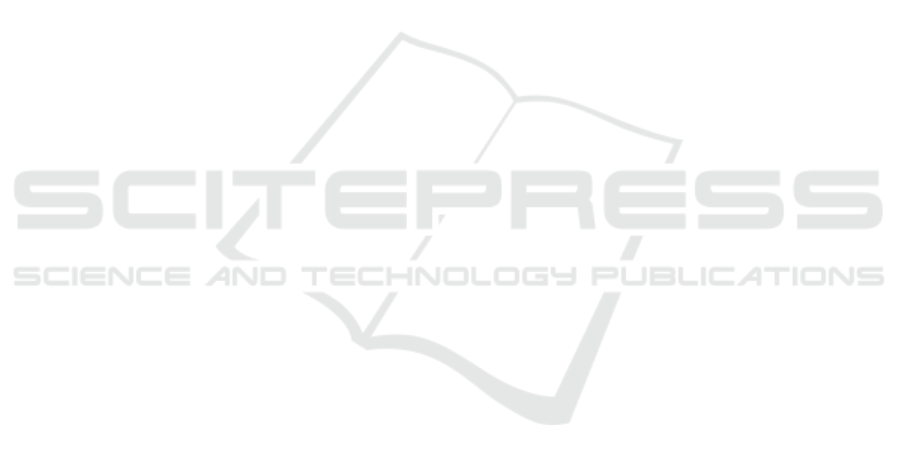
graspability based on grasp regression for better grasp
prediction. ICRA.
Douillard, A., Cord, M., Ollion, C., Robert, T., and Valle, E.
(2020). Podnet: Pooled outputs distillation for small-
tasks incremental learning. ECCV.
Ebrahimi, S., Petryk, S., Gokul, A., Gan, W., Gonzalez,
J. E., Rohrbach, M., and Darrell, T. (2021). Re-
membering for the right reasons: Explanations reduce
catastrophic forgetting. ICLR.
Goodfellow, I. J., Mirza, M., Xiao, D., Courville, A.,
and Bengio, Y. (2014). An empirical investigation
of catastrophic forgeting in gradientbased neural net-
works. In In Proceedings of International Conference
on Learning Representations (ICLR.
Jiang, Y., Moseson, S., and Saxena, A. (2011). Efficient
grasping from rgbd images: Learning using a new
rectangle representation. In 2011 IEEE International
Conference on Robotics and Automation, pages 3304–
3311.
Kirkpatrick, J., Pascanu, R., Rabinowitz, N., Veness, J.,
Desjardins, G., Rusu, A. A., Milan, K., Quan, J.,
Ramalho, T., Grabska-Barwinska, A., Hassabis, D.,
Clopath, C., Kumaran, D., and Hadsell, R. (2017).
Overcoming catastrophic forgetting in neural net-
works. Proceedings of the National Academy of Sci-
ences, 114(13):3521–3526.
Kumra, S., Joshi, S., and Sahin, F. (2020). Antipodal robotic
grasping using generative residual convolutional neu-
ral network. In 2020 IEEE/RSJ International Confer-
ence on Intelligent Robots and Systems (IROS), pages
9626–9633.
Kurmi, V. K., Patro, B. N., Subramanian, V. K., and Nam-
boodiri, V. P. (2021). Do not forget to attend to uncer-
tainty while mitigating catastrophic forgetting. WACV.
Li, Z. and Hoiem, D. (2016). Learning without forgetting.
ECCV, abs/1606.09282.
Lomonaco, V. and Maltoni, D. (2017). Core50: a new
dataset and benchmark for continuous object recog-
nition. CoRR, abs/1705.03550.
Lopez-Paz, D. and Ranzato, M. (2017). Gradient episodic
memory for continual learning. NIPS.
Morrison, D., Corke, P., and Leitner, J. (2018). Closing the
Loop for Robotic Grasping: A Real-time, Generative
Grasp Synthesis Approach. In Proc. of Robotics: Sci-
ence and Systems (RSS).
Redmon, J. and Angelova, A. (2015). Real-time grasp de-
tection using convolutional neural networks. ICRA.
Rusu, A. A., Rabinowitz, N. C., Desjardins, G., Soyer,
H., Kirkpatrick, J., Kavukcuoglu, K., Pascanu, R.,
and Hadsell, R. (2016). Progressive neural networks.
CoRR, abs/1606.04671.
She, Q., Feng, F., Hao, X., Yang, Q., Lan, C., Lomonaco,
V., Shi, X., Wang, Z., Guo, Y., Zhang, Y., Qiao, F., and
Chan, R. H. M. (2020). Openloris-object: A robotic
vision dataset and benchmark for lifelong deep learn-
ing. ICRA.
Shin, H., Lee, J. K., Kim, J., and Kim, J. (2017). Continual
learning with deep generative replay. NIPS.
van de Ven, G. M. and Tolias, A. S. (2019). Three scenarios
for continual learning. CoRR, abs/1904.07734.
Verma, V. K., Liang, K. J., Mehta, N., Rai, P., and Carin, L.
(2021). Efficient feature transformations for discrimi-
native and generative continual learning. CVPR.
Zenke, F., Poole, B., and Ganguli, S. (2017). Continual
learning through synaptic intelligence. ICML.
Zhou, X., Lan, X., Zhang, H., Tian, Z., Zhang, Y., and
Zheng, N. (2018a). Fully convolutional grasp de-
tection network with oriented anchor box. IROS,
abs/1803.02209.
Zhou, X., Lan, X., Zhang, H., Tian, Z., Zhang, Y., and
Zheng, N. (2018b). Fully convolutional grasp detec-
tion network with oriented anchor box. IROS.
VISAPP 2023 - 18th International Conference on Computer Vision Theory and Applications
710